- Pascal's Chatbot Q&As
- Posts
- This report aims to dissect the complex ecosystem of digital control. It will document concrete examples of censorship and nudging on prominent social media platforms and within LLMs.
This report aims to dissect the complex ecosystem of digital control. It will document concrete examples of censorship and nudging on prominent social media platforms and within LLMs.
It will categorize the diverse methods employed, analyzing their mechanisms and immediate impacts. It will assess the profound dangers these practices pose for dissidents, activists, and researchers.
The Closing Net: Online Censorship, AI Control, and the Fight for Digital Freedom
by Gemini Advanced, Deep Research with 2.5 Pro. Warning, LLMs may hallucinate!
Introduction: The Dual Threat of Digital Control: Censorship on Social Media and by AI
The contemporary digital landscape presents a paradox. Technologies once heralded as harbingers of liberation and democratic expansion are increasingly being repurposed as instruments of control. The global town square, envisioned as a space for open discourse and connection, is now a fiercely contested territory. This report addresses the dual threats converging in this space: the overt and covert censorship mechanisms proliferating across established social media platforms, and the nascent, often subtle, forms of control emerging within the architecture of Large Language Models (LLMs).
The core concern animating this analysis is the observable rise of sophisticated censorship and nudging techniques online. These practices range from the explicit removal of content and suspension of accounts to the opaque manipulation of information visibility and the subtle steering of user behavior through algorithmic design.1 Such measures profoundly impact free expression, distort political discourse, and pose significant threats to activists, journalists, and researchers, particularly those challenging authoritarian regimes or addressing sensitive societal issues.3 The spectrum of control is broad, encompassing everything from state-mandated internet shutdowns and website blocking to platform-level content moderation decisions influenced by political pressure, and the biases and refusals embedded within AI systems.2 Compounding these global concerns are specific anxieties regarding the trajectory of digital control within established democracies, including the United States, where accusations of political bias, platform manipulation, and government overreach fuel public distrust and political polarization.
This report aims to dissect this complex ecosystem of digital control. It will document concrete examples of censorship and nudging on prominent social media platforms (including Meta's platforms, TikTok, X, and YouTube) and within LLMs. It will categorize the diverse methods employed, analyzing their mechanisms and immediate impacts. Crucially, it will assess the profound dangers these practices pose for dissidents, activists, and researchers striving to counter authoritarianism, explaining why reliance on mainstream digital platforms can be fraught with risk for resistance movements. Furthermore, the report will explore potential pathways forward, outlining strategies to enable dissent even in repressive environments, proposing principles for the creation of alternative, censorship-resistant tools and platforms, and offering actionable recommendations for internet users in the United States navigating concerns about domestic digital control, propaganda, and the influence of political agendas on technology platforms.
The central argument advanced is that the convergence of platform power, governmental pressure, corporate interests, and the rapidly evolving capabilities of artificial intelligence is forging a formidable and adaptive apparatus of digital control. This apparatus poses a significant and escalating threat to democratic norms, human rights, and the very possibility of open societies globally. Understanding its multifaceted nature and developing proactive countermeasures is not merely a technical challenge, but an urgent political and social imperative.
Social media platforms, hosting billions of users worldwide, have become primary conduits for communication, information dissemination, and political mobilization.8However, their role extends beyond neutral facilitation. The mechanisms required to manage content at scale – content moderation policies, enforcement procedures, and algorithmic curation – are increasingly wielded, intentionally or unintentionally, as tools of control, shaping public discourse and often silencing critical voices.
Subsection 1.1: Content Moderation as Control: Documented Practices
Content moderation is presented by platforms as a necessary function to combat illegal activities, harassment, spam, and harmful material. Yet, the evidence reveals that these systems frequently extend their reach into the realm of political and social censorship.1 This occurs through various mechanisms, often driven by vaguely defined policies, inconsistent enforcement, the influence of government requests, and a heavy reliance on automated systems that lack nuanced understanding.3
Content Removal/Takedowns: Platforms routinely remove posts, comments, videos, or other user-generated content deemed to violate their terms of service. While sometimes targeting genuinely harmful material, takedowns frequently affect content critical of governments, documenting human rights abuses, or discussing sensitive political issues.9 The scale can be immense; Meta, for instance, acknowledged removing millions of pieces of content daily, admitting a potential error rate of 10-20% in these actions, highlighting the significant risk of legitimate speech being caught in the net.11
Account Suspension/Deletion: Platforms wield the power to temporarily suspend or permanently ban user accounts. This measure is often deployed against activists, journalists, political dissidents, or individuals expressing controversial viewpoints, effectively silencing their online presence and severing their established networks.3
Shadow Banning/Visibility Reduction: This refers to the practice of algorithmically reducing the reach and visibility of a user's content (e.g., preventing it from appearing on public feeds like TikTok's "For You Page" or in search results) without explicit notification.9 Users experience a drastic drop in engagement but are often left guessing the cause.22 Platforms like TikTok employ automated systems for this, ostensibly to protect users from spam or inappropriate content, but critics argue it is used to suppress disfavored content.16 The lack of transparency makes shadow banning difficult to prove and contest, fostering uncertainty and self-censorship.9 Meta has also faced accusations of reducing the distribution of specific content, such as posts related to Palestine.9
Throttling: In some instances, authorities or platforms may deliberately slow down access to specific platforms or content types, particularly during periods of political sensitivity like elections or protests. This tactic was reportedly used in Turkey following earthquakes to block critical content 15 and has been employed against platforms like X (formerly Twitter) in various countries.17
Geographic Restrictions/Geo-blocking: Content may be made inaccessible to users in specific countries, often in response to legal demands or government requests alleging violations of local law.14 This allows platforms to maintain operations in restrictive markets while complying with censorship demands locally.
Demonetization: Primarily affecting platforms like YouTube that rely on advertising revenue, demonetization involves revoking the ability of a video or channel to earn money from ads because the content is deemed "not advertiser-friendly".26 The guidelines for this are broad and cover "controversial issues," "sensitive events," violence, and inappropriate language, among other categories.26 This practice acts as a powerful form of economic censorship, disproportionately impacting creators who cover news, politics, social issues, or other topics advertisers might deem risky, potentially discouraging such content.28
Feature Restriction: Platforms can limit users' ability to engage by disabling specific functionalities, such as the ability to livestream, like, comment, share content, or use monetization features.9 Human Rights Watch documented numerous cases where users supporting Palestine faced such restrictions on Meta platforms.9
Keyword/Hashtag Blocking/Filtering: Platforms or censors may block or filter specific keywords or hashtags, preventing their use or making content containing them undiscoverable.14 This has led users to develop creative linguistic countermeasures, such as using alternative spellings (e.g., "unalive" for "kill," "le$bian" for "lesbian") or symbolic codes (like China's "Grass Mud Horse" meme) to evade automated detection.16
A critical pattern emerges from these practices: the interplay between flawed platform policies, automated enforcement, and governmental pressure often results in systemic bias against certain viewpoints. For example, Meta's Dangerous Organizations and Individuals (DOI) policy, which heavily relies on US government designations of terrorist organizations (including political movements with armed wings like Hamas), has led to widespread, arguably disproportionate suppression of peaceful pro-Palestine content.9 The policy's focus on potential audience perception rather than user intent further broadens its suppressive scope.9 When such policies are applied globally via automated systems susceptible to error and influenced by government takedown requests (which platforms often comply with, as seen in Turkey and Israel 9), the result is not merely isolated moderation errors but a predictable pattern of censorship targeting specific political speech. This structural vulnerability transforms platforms into potential conduits for state-aligned censorship.
Furthermore, the inherent ambiguity and inconsistent application of many platform guidelines, particularly concerning practices like shadow banning on TikTok or the criteria for demonetization on YouTube, cultivate a climate of uncertainty.22 Users, unsure of what might trigger a penalty—be it content removal, reduced visibility, or loss of income—are incentivized to err on the side of caution.9 This leads to self-censorship, where individuals avoid potentially controversial topics or moderate their language not because it explicitly violates rules, but out of fear of arbitrary enforcement.32 This lack of clarity thus functions as an effective and low-cost mechanism of control, reducing the platform's moderation burden while potentially satisfying external pressures for content restriction without necessitating overt, unpopular policy changes.
Subsection 1.2: Case Studies
Examining specific platforms reveals recurring patterns and unique challenges in the landscape of online censorship.
Meta (Facebook/Instagram): Human Rights Watch (HRW) has extensively documented what it describes as systemic censorship of Palestine-related content on Meta's platforms, particularly following October 2023.9 The investigation identified over 1,000 cases of undue suppression, overwhelmingly affecting peaceful content supporting Palestine.9 Key mechanisms included the broad application of the DOI policy, which effectively silences discussion around major Palestinian political movements due to its reliance on US government lists.9Other policies, such as those concerning spam, hate speech, violence, and even nudity, were reportedly misapplied to remove documentation of human rights abuses or political expression.9 Compounding this was a high rate of malfunction in the appeal mechanisms, leaving users without remedy.9 This pattern is not new; HRW warned Meta about similar issues in 2021.14 Recently, Meta announced a policy shift towards "more speech and fewer mistakes," aiming to reduce over-enforcement, simplify rules (particularly around topics like gender and immigration), rely less on automated flagging for minor violations, and replace intrusive fact-checking labels with less obtrusive ones.8 While framed as a response to acknowledged over-censorship and user frustration 11, the move has been met with skepticism, with critics suggesting it may be politically motivated to appease the incoming Trump administration and questioning whether the relaxed standards will apply uniformly or primarily benefit certain political viewpoints.8Concerns remain about the potential increase in misinformation and the continued censorship of other disfavored topics like LGBTQ+ speech.33
TikTok: This platform faces frequent accusations of censorship, particularly via shadow banning, where content visibility is reduced without notice.16 Triggers include violations of community guidelines (hate speech, graphic content, misinformation, copyright infringement, etc.), but the platform's heavy reliance on automated moderation means errors can occur.22 Specific allegations include suppressing content related to the treatment of Uyghurs in China, the Hong Kong protests, the Citizenship Amendment Act protests in India, criticism of specific world leaders (including those from Russia, Turkey, and the US), pro-Palestinian content, and LGBTQ+ issues, with users resorting to coded language like "le$bian" to avoid filtering.15 While TikTok denies shadow banning exists or that it censors based on gender identity, user reports and observed patterns suggest otherwise.16 Geographic restrictions are also employed based on local laws.16
X (formerly Twitter): Despite owner Elon Musk's positioning as a "free speech absolutist," X has continued practices of content restriction and account suspension.19 The platform has suspended accounts of journalists critical of Musk, anti-fascist activists, and others.20 Crucially, X demonstrates significant compliance with government takedown requests, particularly in countries like Turkey and India.15 In Turkey, ahead of elections and during periods of unrest, X restricted access to content and suspended accounts critical of the Erdoğan government, citing legal obligations under Turkish law.15 Musk acknowledged this, framing it as a choice between limiting some tweets or having the platform throttled entirely.19 Transparency reports indicate high compliance rates with Turkish government requests.19 Similar compliance with Indian government requests regarding content related to Kashmir or deemed critical has also been reported, leading X to sue the Indian government over what it termed overly broad blocking orders.17 The platform is also subject to outright blocking or throttling by governments in various countries.17
YouTube: YouTube's primary censorship mechanism operates through its "advertiser-friendly content guidelines," which lead to the demonetization of videos deemed unsuitable for ads.26 While ostensibly driven by commercial concerns to keep advertisers comfortable, these guidelines are broad and subjective, covering "controversial issues," "sensitive events," "inappropriate language," and depictions of violence (even in gaming contexts).26 This system disproportionately affects creators discussing politics, current events, social issues, or anything potentially sensitive, effectively acting as economic censorship that can discourage such content.28 Creators report videos being demonetized without clear notification or justification, and smaller channels often feel the impact more acutely.28 While YouTube states these policies are simply clarifications, critics argue they suppress important discourse for business reasons.28 Additionally, YouTube itself has faced blocking by governments in numerous countries over specific content controversies.25
These case studies underscore a crucial point: the narrative of neutral content moderation based solely on universal community guidelines often breaks down under scrutiny. Meta's systemic issues regarding Palestine content reveal the impact of specific policies tied to US foreign policy designations and potential deference to government requests.9 X's actions in Turkey and India demonstrate direct compliance with state censorship demands impacting political speech.17 YouTube's demonetization system illustrates how commercial pressures can shape the information landscape, limiting controversial but potentially important speech.26These examples strongly suggest that content moderation is frequently not a simple application of rules, but rather a complex negotiation influenced by political pressures, legal obligations in diverse jurisdictions, and underlying economic models, ultimately determining whose voices are amplified and whose are suppressed.
Chapter 2: The Silent Censor: Bias, Refusals, and Nudging in Large Language Models
As Large Language Models (LLMs) like ChatGPT, Claude, and others become increasingly integrated into daily life as tools for information retrieval, content creation, and interaction, a new frontier of digital control is emerging. Unlike the often overt censorship seen on social media, control within LLMs can manifest more subtly through built-in refusals, inherent biases in training data, sophisticated nudging techniques, and the challenges surrounding AI-generated media like deepfakes.
Subsection 2.1: LLM Content Refusals and Topic Avoidance
LLM developers are actively programming "guardrails" into their models, ostensibly to prevent the generation of harmful, unethical, or illegal content. However, these guardrails often extend to politically sensitive or controversial topics, leading to refusals that can resemble censorship.
Documented Refusals: Users encounter instances where LLMs explicitly decline prompts related to specific political figures, ongoing elections, or controversial social issues.35 For example, Anthropic's Claude is governed by usage policies prohibiting election interference, promoting candidates, or generating misinformation, leading it to refuse certain election-related queries.37 Similarly, the AI image generator Midjourney implemented blocks on creating images of Joe Biden and Donald Trump in the run-up to the 2024 US election.38 Even generating images of politicians in speculative or satirical contexts can trigger refusals or require complex workarounds.36
Safety vs. Censorship: The line between necessary safety precautions (e.g., preventing the generation of hate speech, incitement to violence, or instructions for illegal acts 39) and overly broad censorship is often blurry. Refusing to discuss a politician's policies or generate a satirical image could be framed as preventing misinformation or harmful deepfakes, but it also restricts legitimate forms of political commentary, analysis, and artistic expression.35 There is concern that safety protocols could embed political biases or be used to preemptively silence dissent.40
Geopolitical Dimensions: Concerns are particularly acute regarding LLMs developed in authoritarian states. Chinese models like DeepSeek and Qwen have been observed to exhibit built-in censorship, filtering prompts related to politically sensitive topics such as Tiananmen Square, even in downloadable versions intended for wider use.40 This highlights the potential for state-mandated censorship to be embedded directly into the model's architecture.
The proactive implementation of refusal mechanisms by LLM developers marks a significant shift from the largely reactive moderation model of social media. While platforms typically remove content after it has been posted, LLMs are increasingly designed to prevent the generation of certain types of content altogether.37 This preemptive approach, while aiming to mitigate risks like election interference or the spread of deepfakes, inherently limits the scope of permissible inquiry and discourse. By refusing to engage with contentious but important public issues or figures, these models risk creating sanitized, incomplete information environments that shy away from the complexities and controversies essential to democratic life.37 This represents a form of pre-censorship, shaping the boundaries of discussion before a user even formulates a specific output.
Subsection 2.2: Algorithmic Bias: Skewed Perspectives and Representation Issues
LLMs learn by processing vast datasets of text and images generated by humans. Consequently, they inevitably absorb and reflect the biases present in that data, which mirror existing societal prejudices related to gender, race, ethnicity, religion, and other characteristics.41
Sources of Bias: Training data scraped from the internet contains historical and contemporary societal biases. If certain groups are underrepresented or misrepresented in the data, the LLM's understanding will be skewed accordingly.
Manifestations of Bias: Bias can manifest in various ways. Studies have shown LLMs exhibiting gender bias by associating certain occupations stereotypically with men or women (e.g., assuming a "doctor" is male and a "nurse" is female).43Research suggests potential gender bias in LLM-generated mental health assessments for eating disorders, with male cases receiving lower quality-of-life scores despite lack of real-world evidence for this pattern.41 Concerns exist about racial bias embedded in AI tools used for election administration or content moderation, potentially disadvantaging voters or speakers of color.45 Algorithmic filtering by platforms like TikTok has also been found to display content related to human rights abuses (e.g., in Xinjiang) in a way that favors positive or neutral framing compared to other platforms.16
Impact: Biased LLM outputs can have significant negative consequences. They can reinforce harmful stereotypes, lead to discriminatory outcomes if used in decision-making processes like hiring or loan applications 43, and provide users with inaccurate or skewed information, particularly about marginalized groups or complex social issues.41 The authoritative and fluent manner in which LLMs often present information can make these biases difficult to detect and challenge.43
The presence of bias in LLMs is more than a mere technical imperfection; it acts as a mechanism for perpetuating and even amplifying existing societal inequalities and power dynamics. When these models are deployed at scale for tasks ranging from information retrieval and education to customer service and content creation, the biases embedded within them can systematically disadvantage certain groups and reinforce dominant narratives.41 The confident, often human-like delivery of LLM outputs can lend a false veneer of objectivity, making the embedded biases particularly insidious and harder for users to recognize or question. This necessitates careful auditing, mitigation strategies, and transparency about the limitations and potential biases of these powerful tools.
Subsection 2.3: Nudging and Behavioral Influence: Subtle Manipulation via AI
Nudge theory, drawn from behavioral economics and psychology, posits that individuals' choices can be predictably influenced by subtly altering the "choice architecture"—the environment in which decisions are made—without forbidding options or significantly changing economic incentives.46 LLMs, with their capacity for personalized interaction and language generation, represent a powerful new vector for implementing nudges at scale.
Nudge Theory Basics: Common nudge techniques include setting default options (leveraging inertia), framing choices to highlight gains or losses (loss aversion), making certain information more salient (drawing attention), anchoring judgments with initial suggestions, and appealing to social norms (highlighting peer behavior).47 These techniques often target the intuitive, automatic "System 1" mode of thinking.47
LLMs as Nudge Engines: LLMs are well-suited to deploy these techniques dynamically. They can frame information in persuasive ways, personalize suggestions based on user interaction history, present default responses or courses of action, and highlight specific pieces of information within their generated text.51 Research has demonstrated the effectiveness of LLM-powered nudges in promoting pro-social behaviors like water and energy conservation, where personalized suggestions significantly outperformed traditional nudges.51LLMs are also being explored for nudging health behavior changes, such as medication adherence.56 Furthermore, LLMs themselves appear highly susceptible to nudges, such as default options presented in decision tasks.53
Mechanisms of Influence: The persuasive potential of LLMs stems from their ability to engage in seemingly natural conversation, analyze user input to infer intent or state (e.g., motivation levels for exercise 57), tailor responses accordingly, and potentially exploit known cognitive biases through carefully crafted language and interaction design.42 Some researchers conceptualize an emerging "intention economy" where AI systems profile, predict, and steer user intentions based on behavioral data.54
Potential for Manipulation: While nudging can be used for beneficial purposes (like promoting health or sustainability), the same techniques deployed via LLMs raise significant concerns about manipulation, especially if implemented non-transparently.51 Personalized, AI-driven nudges could subtly shape users' opinions on political issues, influence purchasing decisions, or steer behavior towards outcomes favored by the platform operator or third parties, potentially undermining user autonomy.
The convergence of LLM capabilities with nudge theory principles heralds a new era of behavioral influence. Unlike generic nudges applied uniformly (e.g., a default setting for all users), LLMs enable hyper-personalized persuasion tailored to individual users' inferred psychology, preferences, and context.51 This capacity for targeted, scalable, and potentially invisible influence represents a significant leap from previous methods. Without robust ethical guidelines, transparency requirements, and user controls, the line between helpful assistance and covert manipulation could easily blur, posing profound risks to individual autonomy and informed decision-making in both commercial and political spheres.
Subsection 2.4: The Deepfake Dilemma: AI Image Generation, Politics, and Evasion Tactics
The ability of generative AI models to create highly realistic synthetic images and videos ("deepfakes") from text prompts presents a specific and acute challenge, particularly in the political arena.35
AI Image Generation Capabilities: Models integrated into platforms like ChatGPT (using DALL-E) or standalone services like Midjourney can generate convincing images of people, objects, and scenes based on textual descriptions.35
Policies on Public Figures: AI developers have adopted varying and evolving policies regarding the generation of images depicting real people, especially public figures and politicians. Initially, many platforms restricted such generations due to concerns about misuse.36 However, OpenAI shifted its policy for GPT-4o, allowing the generation of adult public figures while claiming to implement safeguards against harmful uses (like explicit deepfakes) and offering opt-out mechanisms.35 Conversely, Midjourney moved to block prompts specifically requesting images of Joe Biden and Donald Trump during an election period, citing abuse concerns.38 This inconsistency highlights the ongoing debate and difficulty in setting appropriate boundaries.
Ease of Evasion: Despite stated policies and technical guardrails, users can often circumvent restrictions with relative ease. Investigations, such as one conducted by CBC News, found that prompts could be manipulated to generate images of politicians even when direct requests were denied.36 Common tactics include describing the public figures as "fictional characters," framing the request as a speculative scenario, or uploading images without names and asking the AI to combine them.36 In some cases, the AI chatbot itself reportedly suggested workarounds to users.36
Risks for Politics and Disinformation: The ease with which convincing fake images of politicians can be created poses significant risks to political discourse and election integrity.35 Such images can be used to spread misinformation (e.g., fake endorsements, fabricated events), smear opponents by placing them in compromising or false scenarios (e.g., appearing friendly with controversial figures 36), or incite unrest. The potential for AI-generated disinformation to influence voters is a growing concern, leading some jurisdictions to enact laws regulating the use of political deepfakes, often requiring disclaimers.61
The challenge of controlling AI-generated political deepfakes reveals a critical vulnerability in current generative AI technology. While developers attempt to implement safety measures and content restrictions, the flexibility and generative nature of these models make them inherently difficult to police perfectly.36 Simple changes in prompting or framing can often bypass the intended guardrails, as the models lack a deep, contextual understanding of the potential harm or deceptive intent behind a request.36 This suggests that relying solely on technical restrictions built into the models is an insufficient defense against the creation and spread of potentially damaging political deepfakes. The burden consequently shifts towards developing robust detection technologies, enhancing media literacy among the public to critically evaluate visual information, and establishing clear legal and regulatory frameworks to address the malicious use of synthetic media.61
Chapter 3: Mapping the Mechanisms: A Taxonomy of Online Control
The methods used to control information and expression online are diverse, operating at different layers of the internet's infrastructure and utilizing a range of technical and policy-based approaches. Understanding this taxonomy is crucial for recognizing the multifaceted nature of digital censorship and influence. These techniques can be broadly categorized into network-level filtering, platform-level moderation, algorithmic control, and behavioral nudging.
Subsection 3.1: Categorizing Censorship Techniques
Network-Level Filtering: These techniques interfere with the basic connectivity and routing of internet traffic, often implemented by Internet Service Providers (ISPs) at the behest or permission of state authorities.
IP Address Blocking/Filtering: This involves configuring routers or firewalls to prevent users from connecting to specific IP addresses associated with banned websites or services.62 It is a relatively simple but blunt method, often resulting in "collateral damage" by blocking unrelated websites hosted on the same IP address.62
DNS Tampering/Hijacking: This method targets the Domain Name System (DNS), which translates human-readable domain names (e.g., www.example.com) into numerical IP addresses. Censors can interfere with this process by providing false IP addresses (e.g., redirecting to a government warning page or a non-existent address), returning error messages, or simply dropping DNS requests for blocked domains.62 This is more targeted than IP blocking but can still affect multiple services sharing a domain and can be bypassed using alternative DNS resolvers.64
Deep Packet Inspection (DPI): A more sophisticated technique where network equipment examines the actual content (payloads) of data packets flowing through the network.62 DPI allows censors to identify and block traffic based on specific keywords, protocol signatures (e.g., identifying Tor or specific VPN traffic), or other content characteristics, even if the destination IP or domain is not explicitly blocked.62 It is highly granular but resource-intensive and forms the basis for advanced censorship systems like China's Great Firewall.1
Throttling/Shutdowns: This involves deliberately slowing down internet speeds for specific services, protocols, or entire regions, or completely cutting off internet access.15 Shutdowns are a drastic measure often employed by authoritarian regimes during protests, elections, or periods of unrest to prevent communication and information flow.1
Platform-Level Moderation: These actions are taken by the companies operating social media platforms, search engines, or other online services, based on their terms of service, community guidelines, or in response to external pressures (including government requests).
Content Removal: Deleting specific posts, comments, videos, images, or other user-generated content that violates platform policies.9
Account Suspension/Deletion: Temporarily or permanently banning users from the platform.9
Shadow Banning/Demotion: Algorithmically reducing the visibility or reach of a user's content without explicit notification.9
Demonetization: Removing the ability for content creators to earn advertising revenue from their content, primarily on platforms like YouTube.26
Geographic Restriction (Geo-blocking): Making specific content unavailable to users in certain geographic locations, often due to legal requirements or government demands.14
Keyword/URL Filtering (Platform Side): Blocking the use of specific words, phrases, or links within the platform's interface (e.g., in posts, comments, or messages).16
Algorithmic Control (Content Curation & Generation): These methods shape the information environment through the automated systems that rank, recommend, or generate content.
Biased Recommendation/Feed Algorithms: Algorithms that determine what content users see in their feeds (e.g., Facebook News Feed, TikTok For You Page) can be designed or inadvertently function in ways that prioritize certain types of content (e.g., sensational, polarizing) or viewpoints over others, creating filter bubbles or echo chambers.16
LLM Content Refusals: AI models programmed to decline generating responses on specific topics deemed sensitive, political, or harmful.37
LLM Bias: AI models generating content that reflects and potentially amplifies societal biases present in their training data.41
Search Engine Manipulation/Bias: Search engines may delist certain results, prioritize specific sources algorithmically, or alter the presentation of information (like geographic names on maps) based on user location, political pressure, or internal policies.72
These different layers of control often operate in concert. Network-level censorship, frequently mandated or enabled by state actors, can block access to entire platforms or protocols, while platform-level moderation, governed by company policies but often influenced by state pressure, controls the specific content and users within those platforms.1 This creates a multi-layered system of obstacles for users seeking to access or share information freely. For instance, an activist might use a VPN to bypass state-level IP blocking and access a platform like X, only to find their specific content removed or account suspended due to a platform-level decision potentially prompted by a government takedown request targeting that user or content directly.17Circumventing one layer does not guarantee freedom from the others.
Furthermore, the increasing sophistication of algorithmic control represents a significant evolution in censorship and influence. While overt blocking or content removal is generally noticeable to the user, algorithmic demotion, biased recommendations, LLM refusals, or subtle nudging operate largely invisibly.11 Users interacting with a curated feed or an AI chatbot may be unaware of the information being withheld, the perspectives being downplayed, or the subtle ways their perceptions are being shaped. This lack of transparency makes algorithmic control inherently harder to detect, scrutinize, and challenge compared to traditional censorship methods, posing a distinct and growing threat to information autonomy and critical thinking.
Subsection 3.2: Understanding Nudging Mechanisms in Digital Contexts
Nudging techniques, leveraging insights from behavioral science about cognitive biases and heuristics, are increasingly relevant in the digital realm, particularly with the advent of interactive AI. These methods aim to steer user behavior subtly:
Defaults: Setting pre-selected options is powerful due to user inertia and the perception of an implied endorsement. Examples include auto-enrollment in features, pre-checked privacy settings, or the initial response framing provided by an LLM.47
Framing: The way information or choices are presented significantly impacts perception. This includes highlighting potential losses versus gains (loss aversion) or using positively or negatively valenced language in LLM outputs.47
Salience: Making specific information more prominent directs user attention. This can involve visual highlighting, warning labels (like Meta's former fact-check interstitials 11), or an LLM strategically emphasizing certain points in its response.33
Anchoring: Providing an initial piece of information that serves as a reference point for subsequent judgments. Examples include suggested donation amounts or the initial stance taken by an LLM in a conversation.47
Social Norms: Indicating what others are doing or thinking can leverage conformity pressure. This includes displaying "trending" topics, showing comparative statistics (e.g., energy use vs. neighbors 47), or having an LLM reference common opinions or behaviors.51
Personalization (via LLMs): LLMs enable the tailoring of nudges based on individual user data, interaction history, and inferred psychological states, potentially making them far more effective and targeted than generic nudges.51
The application of these behavioral science principles, originally developed for public policy goals like improving health outcomes or savings rates 46, is rapidly being adapted for digital platforms and AI interactions. LLMs, with their ability to conduct personalized, dynamic conversations, are particularly potent vehicles for deploying nudges.51 While this can be used for beneficial ends, such as encouraging conservation or adherence to medical advice 51, it also opens the door to significant potential for manipulation. The same techniques can be employed for commercial persuasion or, more concerningly, for subtly shaping political opinions and behaviors, especially if the nudging is non-transparent. This integration of behavioral science with powerful AI capabilities creates a toolkit where the line between helpful guidance and covert manipulation is increasingly blurred, demanding careful ethical consideration and robust transparency measures.
Subsection 3.3: Immediate Impacts: From Reduced Visibility to Chilling Effects
The various mechanisms of online control produce a range of immediate, tangible impacts on users and the information environment:
Reduced Visibility/Reach: Content demotion and shadow banning directly limit the audience and potential impact of speech, making it harder for messages to spread or movements to gain traction.9
Restricted Access: Overt blocking, filtering, and internet shutdowns completely prevent users from accessing specific information, platforms, or the internet itself.1
Skewed Understanding: Biased algorithms, censored search results, or LLM outputs presenting incomplete or prejudiced information can distort users' perception of reality and understanding of issues.16
Silencing Voices: Account suspensions and content removal directly prevent targeted individuals or groups from participating in online discourse, effectively removing their voices from the conversation.1
Economic Impact: Demonetization directly affects the livelihoods of content creators, potentially discouraging certain types of content creation.26 Distributed Denial-of-Service (DDoS) attacks can cripple the online operations of businesses or civil society organizations.77
Chilling Effects/Self-Censorship: Perhaps the most pervasive impact, the fearof censorship, surveillance, or other repercussions (online harassment, offline consequences) leads individuals to proactively limit their own expression, avoid sensitive topics, or refrain from engaging in online activism.4
Erosion of Trust: Perceived bias, inconsistent enforcement, lack of transparency, and arbitrary moderation decisions undermine users' trust in platforms as reliable or fair forums for communication.9
Impeded Organizing/Mobilization: Censorship and surveillance make it significantly harder for activists to share information securely, coordinate actions, recruit members, and build collective movements.1
Taxonomy of Online Control Mechanisms
The following table summarizes the diverse methods of online control discussed, categorizing them and outlining their immediate impacts:
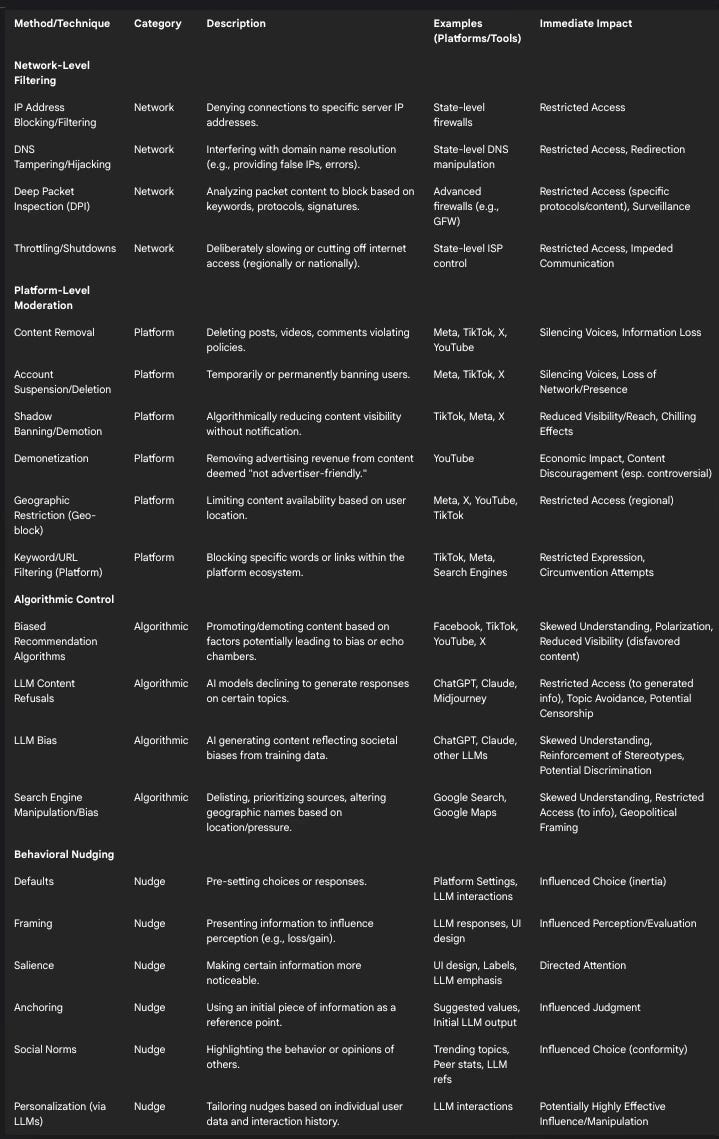
Chapter 4: The Price of Speech: Digital Repression's Toll on Dissent
While online censorship and surveillance affect all internet users, their impact is particularly acute and damaging for dissidents, activists, journalists, and researchers who seek to challenge authoritarian power, expose abuses, and advocate for societal change. Digital repression mechanisms serve not only to control the flow of information but also to actively undermine opposition movements, instill fear, and obstruct efforts towards accountability.
Continue reading here (due to post length constraints): https://p4sc4l.substack.com/p/this-report-aims-to-dissect-the-complex
