- Pascal's Chatbot Q&As
- Posts
- Summary of Day 2 of The Generative AI Summit 2025, London Edition. Generative AI must not be a curiosity—it must drive measurable business value...
Summary of Day 2 of The Generative AI Summit 2025, London Edition. Generative AI must not be a curiosity—it must drive measurable business value...
...while protecting the integrity of research, authorship, and institutional trust. Generative AI is no longer optional. It is now a question of governance, differentiation, and long-term relevance.
Day 2 Summary: Generative AI in the Enterprise
Executive Imperatives: Monetize AI While Minimizing Risk
Day 2 of the Generative AI Summit in London emphasized the transition from AI experimentation to enterprise integration. For scholarly publishers, the key takeaway is that generative AI must not be a curiosity—it must drive measurable business value, while protecting the integrity of research, authorship, and institutional trust.
Clustered Insights and Strategic Takeaways
1. Data Foundations Are Non-Negotiable
Clean, structured, permissioned data is the foundation of all AI value.
In pharma and finance, poor data quality stalled AI deployments—even in invoice processing and forecasting.
FAIR data (Findable, Accessible, Interoperable, Reusable) continues to be a key enabler for effective AI use.
For Scholarly Publishers:
Publishers must inventory and optimize their metadata, ontologies, and content repositories to ensure AI-readiness.
Clean citation graphs, licensing metadata, and author disambiguation will be essential for any monetizable AI service.
Invest in internal data governance before scaling outward-facing AI features.
2. Monetization Requires Measurable ROI—Not Just Hype
AI success is often framed around cost avoidance, efficiency gains, and coverage expansion, not just revenue.
Early adopters noted that AI adoption improved speed, quality of insight, and employee satisfaction—all indirectly tied to financial health.
However, hallucinations, security concerns, and trust gaps slow ROI and lead to stalled implementations.
For Scholarly Publishers:
Monetize AI via:
AI-enhanced discovery (e.g., semantic search, synthesis tools).
Intelligent editorial tools that reduce time-to-publish.
Content repackaging (e.g., summarizations, visualizations, tutoring content).
Measure not just income, but:
Reviewer and author experience gains.
Reduced time-to-insight for readers.
Decrease in support tickets or manual interventions.
3. Don't Scale Without Strategy: Build vs. Buy vs. Co-Create
AI maturity varies; leaders cautioned against rushing to deploy tools without a lifecycle plan.
Pharma and finance executives shared pitfalls from buying "off-the-shelf" models that couldn’t scale or align with regulatory needs.
Co-creation with trusted partners (including academic institutions) is emerging as a powerful middle ground.
For Scholarly Publishers:
Treat AI projects like products—with lifecycle funding, sunset plans, and governance.
Build when you own the data and the use case is critical.
Buy when speed-to-market matters more than differentiation.
Co-create when long-term differentiation and risk-sharing are needed (e.g., AI citation advisors, education products).
4. Inclusive AI = Sustainable AI
True inclusivity means designing AI for non-native English speakers, low-resource users, and low-data domains.
Several education leaders warned: AI can widen the gap unless equal access is designed in (e.g., language bias, access costs, AI literacy).
Enterprises with diverse inputs performed better in model training and stakeholder adoption.
For Scholarly Publishers:
Ensure AI tools work across global author bases—especially in the Global South.
Integrate content from underrepresented regions into training data (ethically and legally).
Offer public-access or low-cost AI tools for researchers and students in less-privileged contexts.
Universities now teach students to critically evaluate AI outputs, not just consume them.
AI is no longer “a tool”—it’s a co-pilot, a tutor, and a potential generator of scholarly-sounding text.
The role of the educator (and publisher) is evolving into contextual guide and integrity guardian.
For Scholarly Publishers:
Develop services that enhance epistemic trust, not dilute it:
Fact-checking and provenance tools.
AI-generated content flags and disclaimers.
Author verification and citation traceability.
Support institutions in maintaining educational rigor with tools that promote critical thinking, not shortcuts.
6. Risk Management: AI Is a Compliance and IP Issue
Companies in finance and pharma were emphatic: no AI deployment without data access controls, audit trails, and model explainability.
Trust is hard to regain once lost—especially in regulated environments.
For Scholarly Publishers:
Implement AI guardrails: license enforcement, content leakage prevention, and human-in-the-loop oversight.
Be proactive in setting internal standards for AI-generated metadata, abstracts, or summaries.
Collaborate with legal and ethics teams on permissible uses for internal and external AI tools.
Final Thought for C-Level Leaders
Generative AI is no longer optional. It is now a question of governance, differentiation, and long-term relevance.
The summit made it clear: those who align AI strategy with business health, intellectual trust, and ethical inclusion will lead—not lag—this transformation.
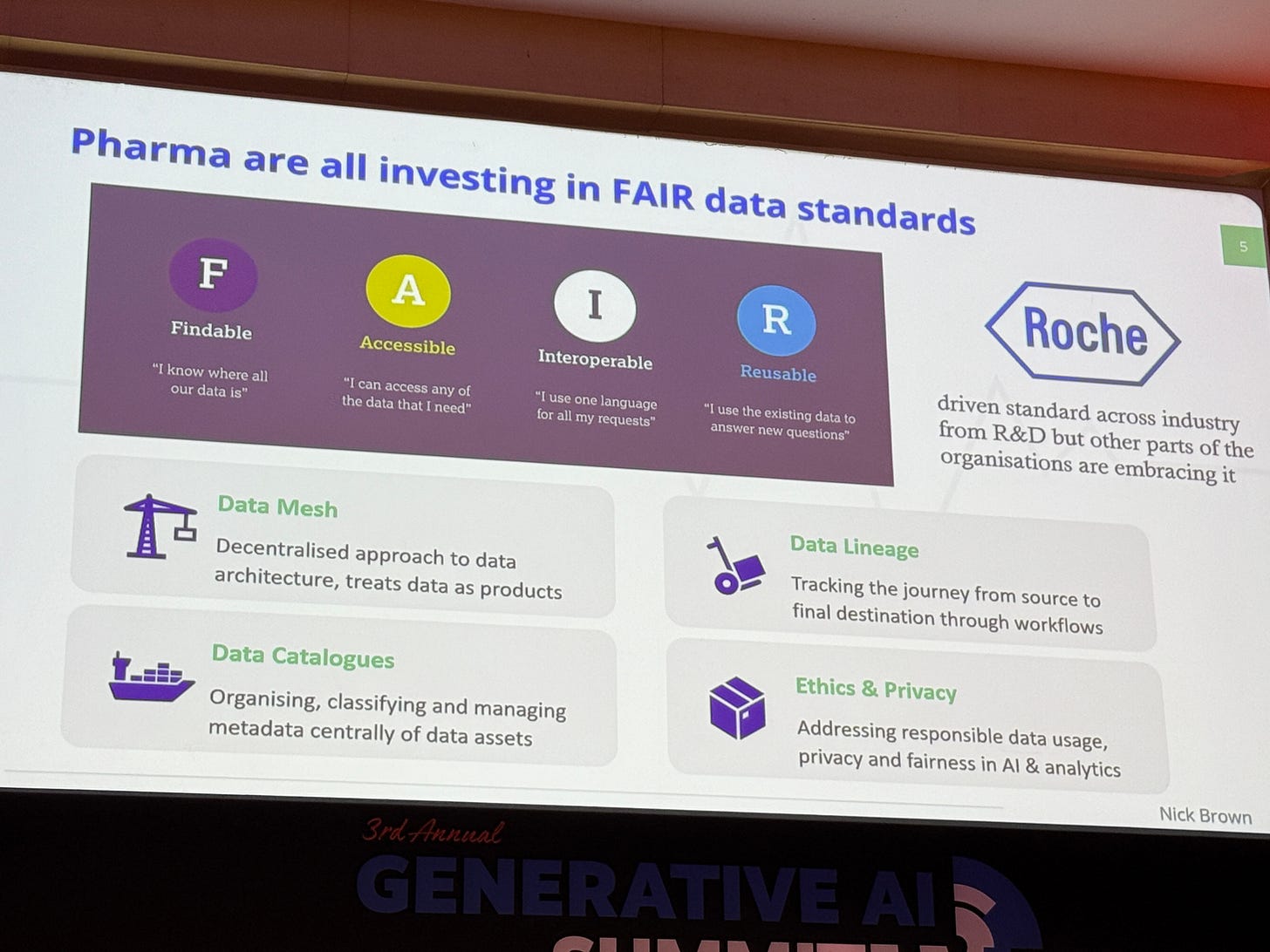
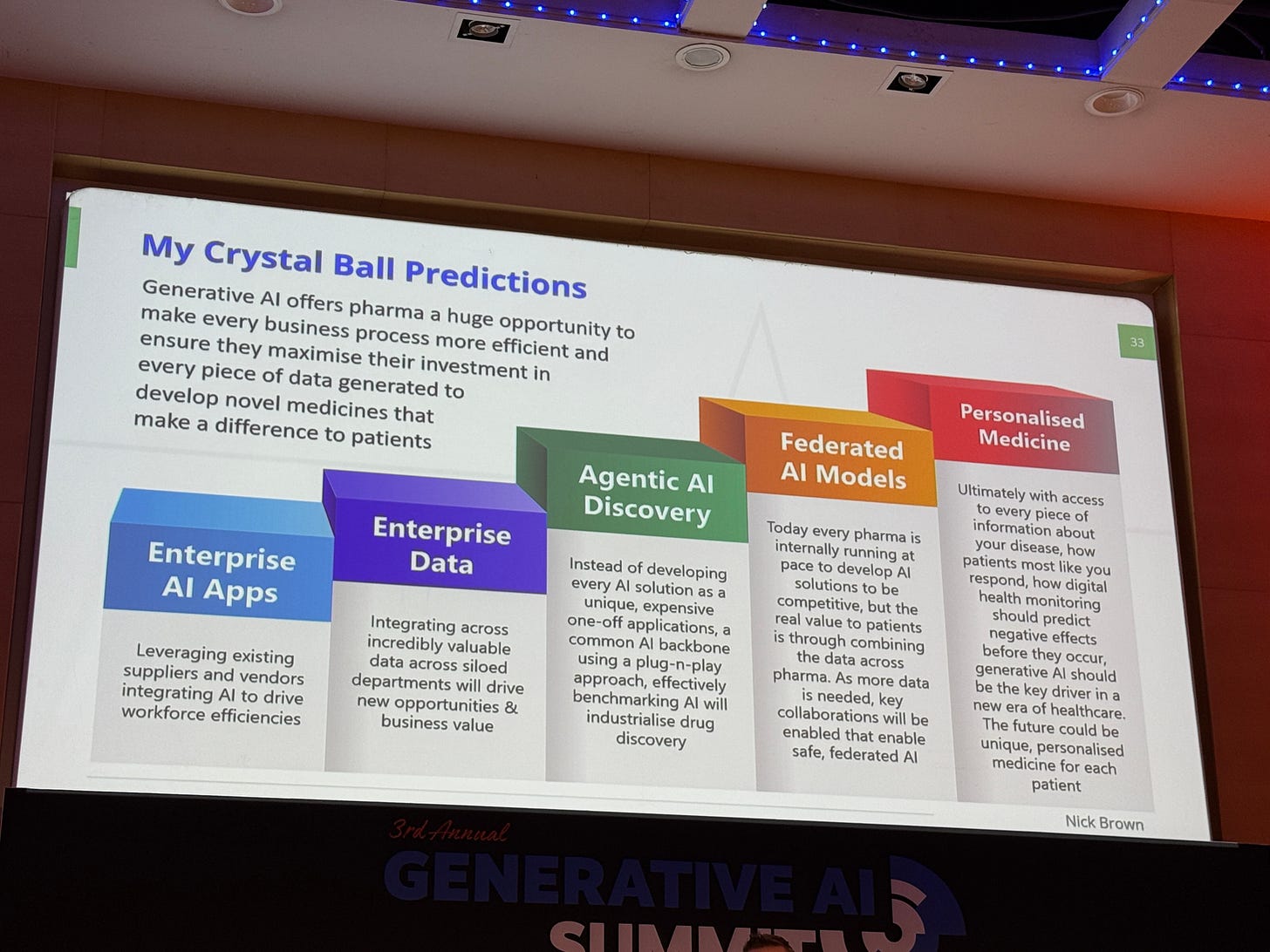
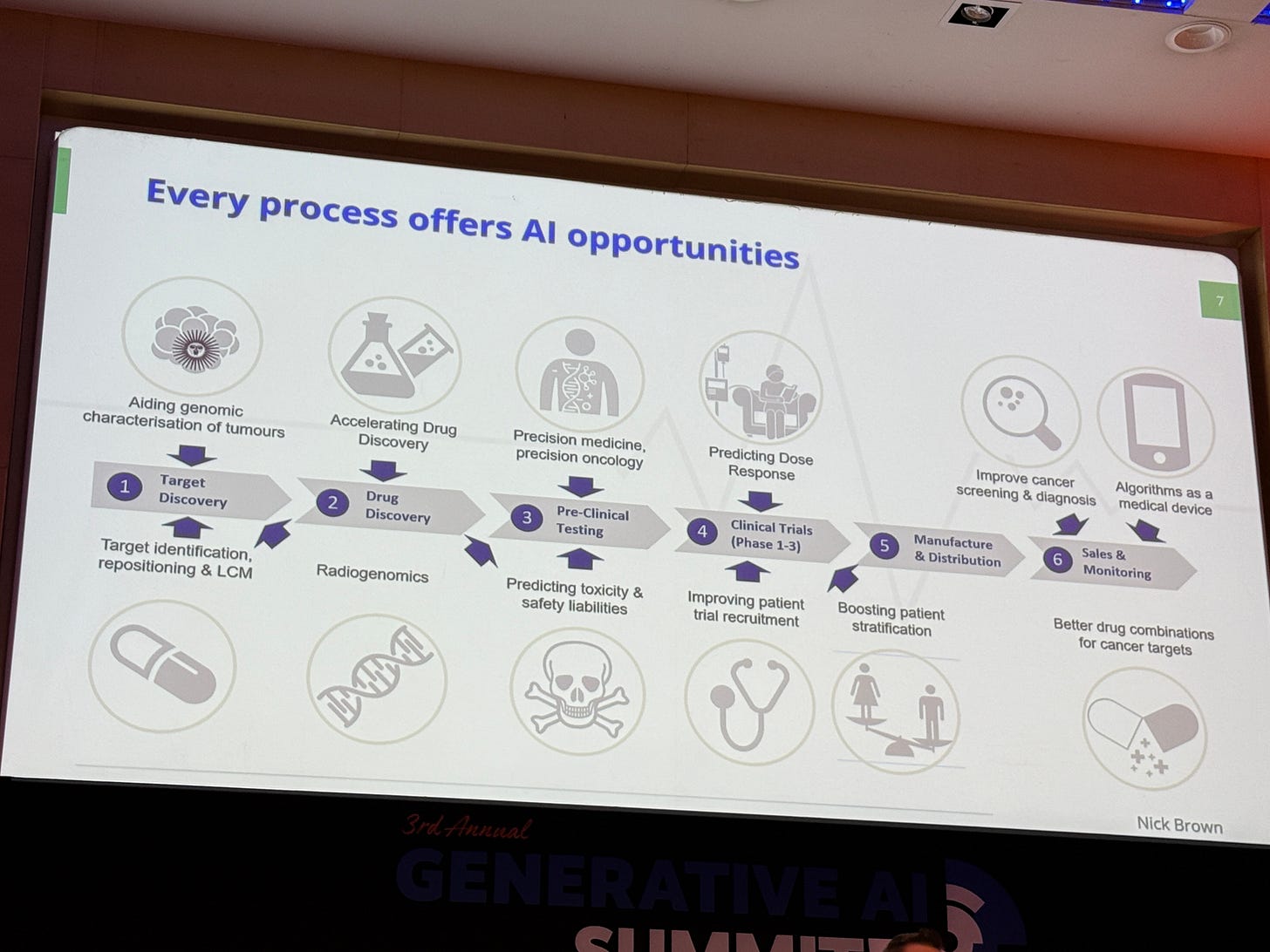
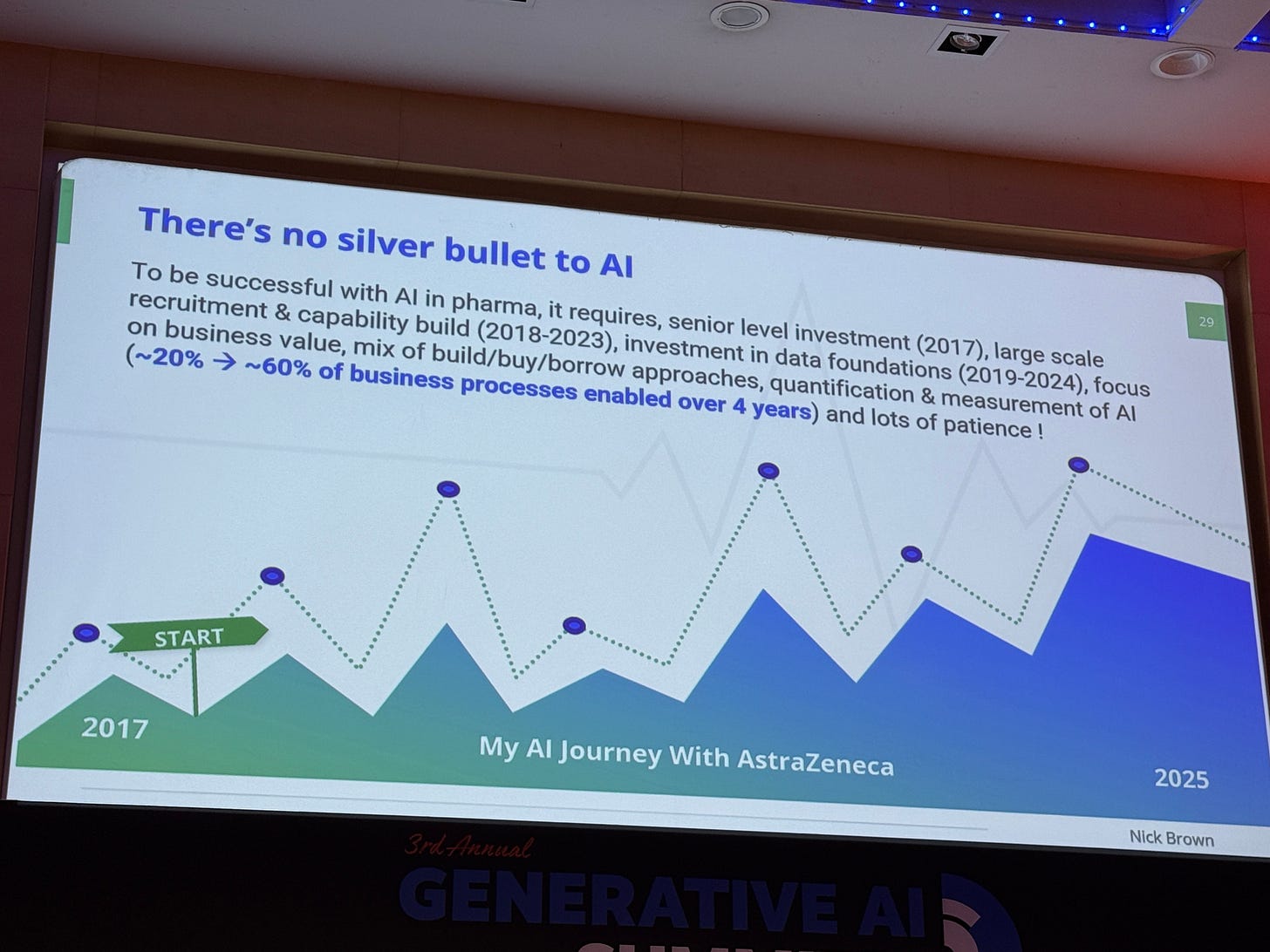
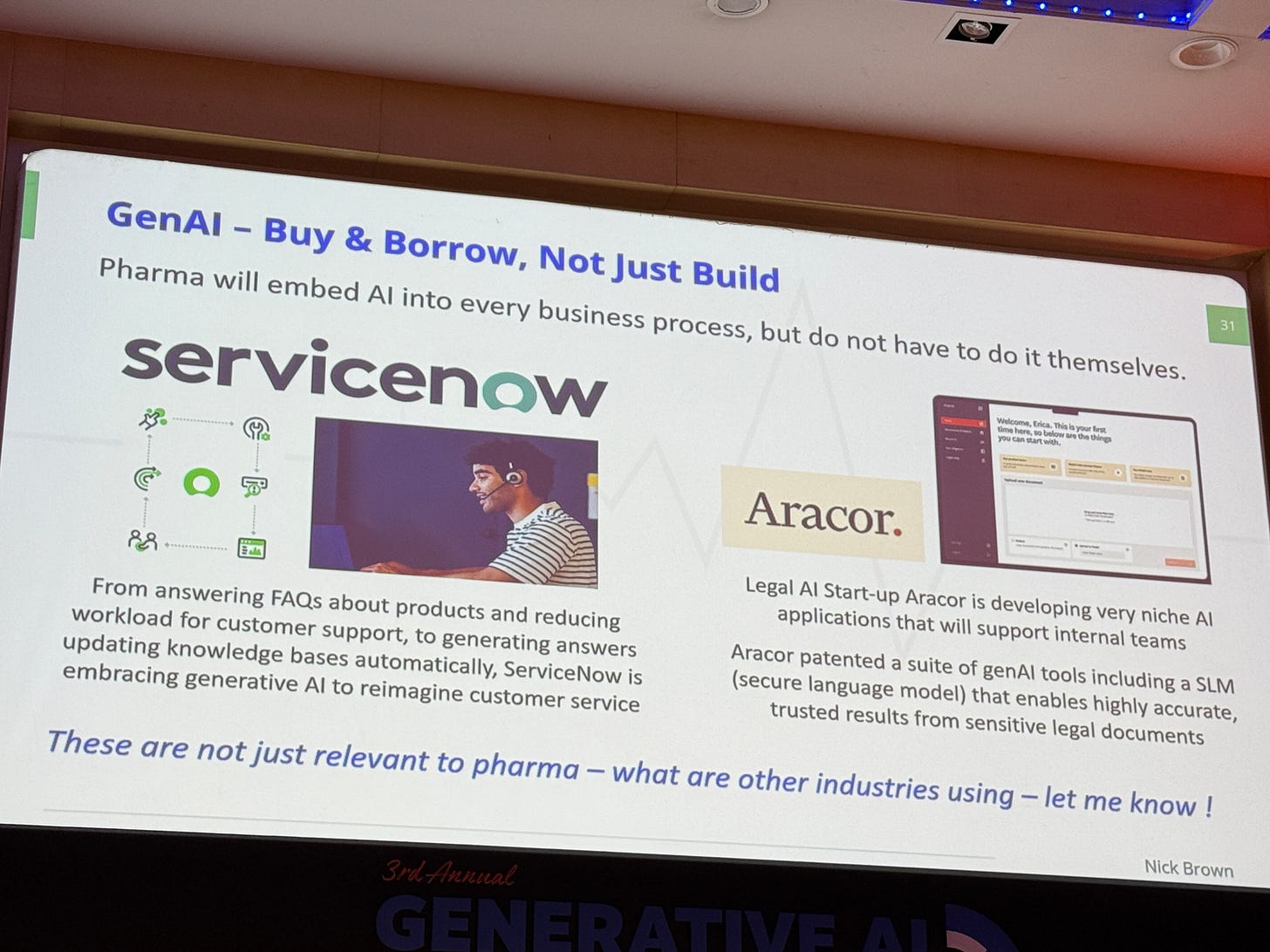
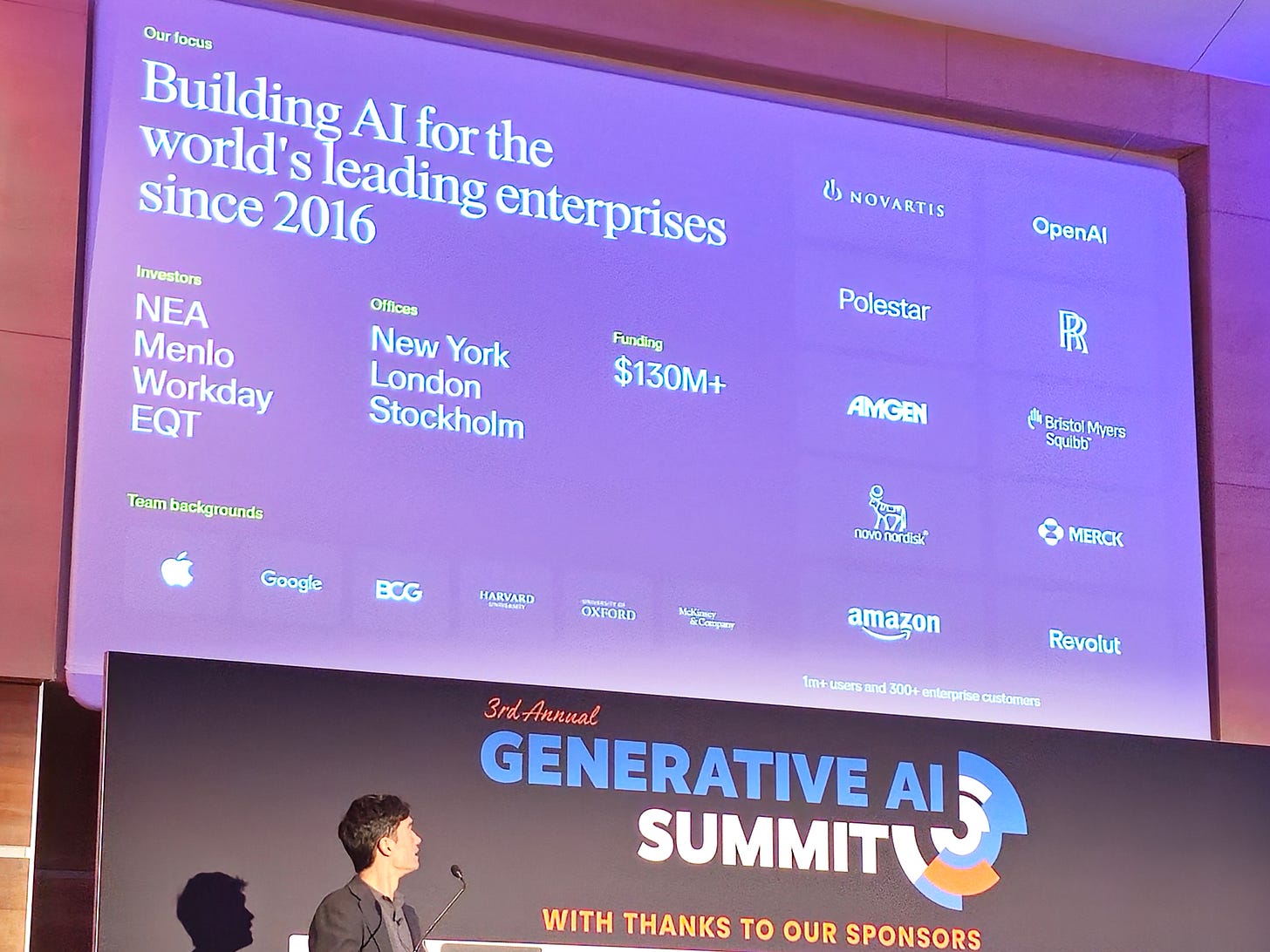
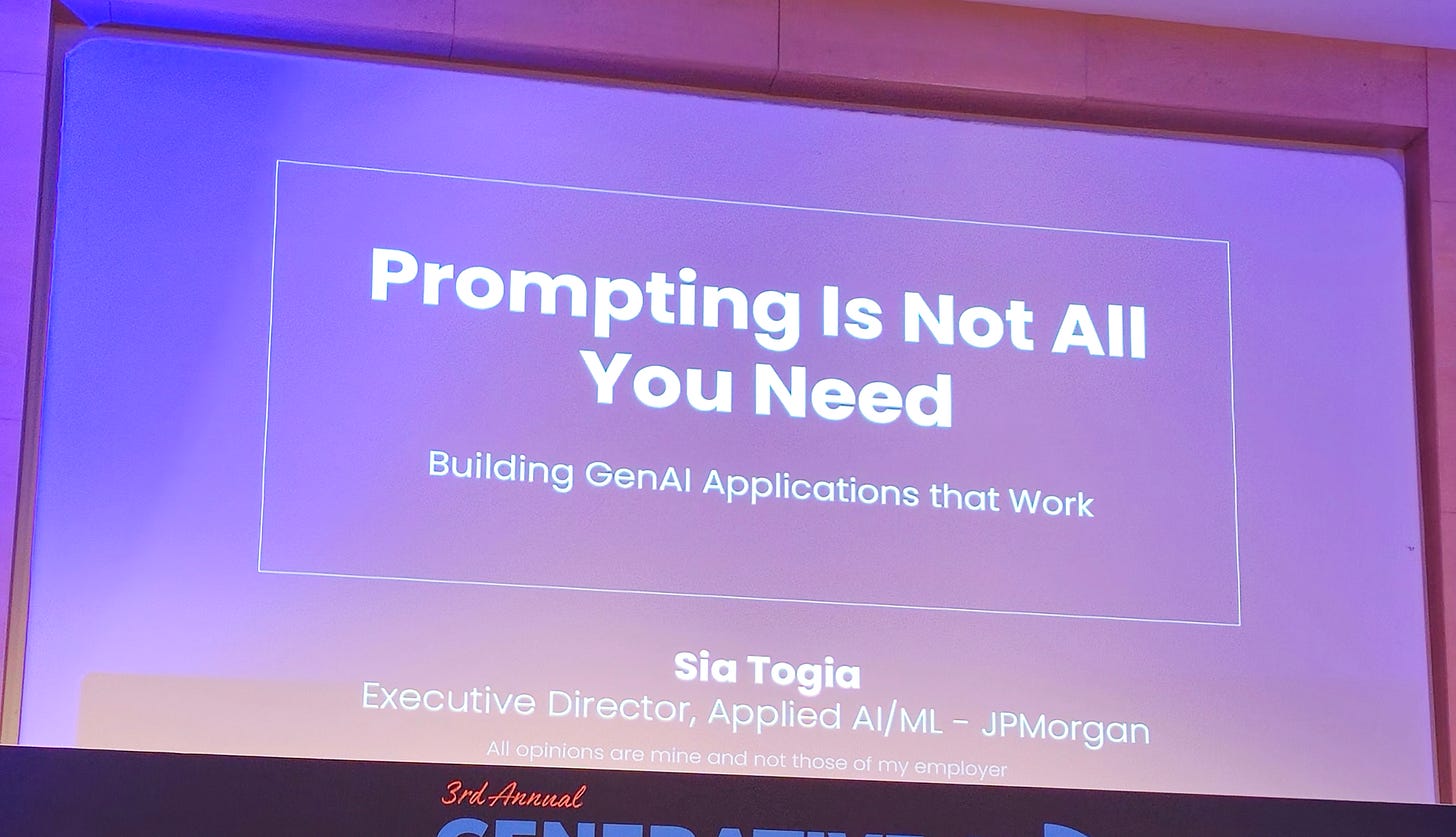
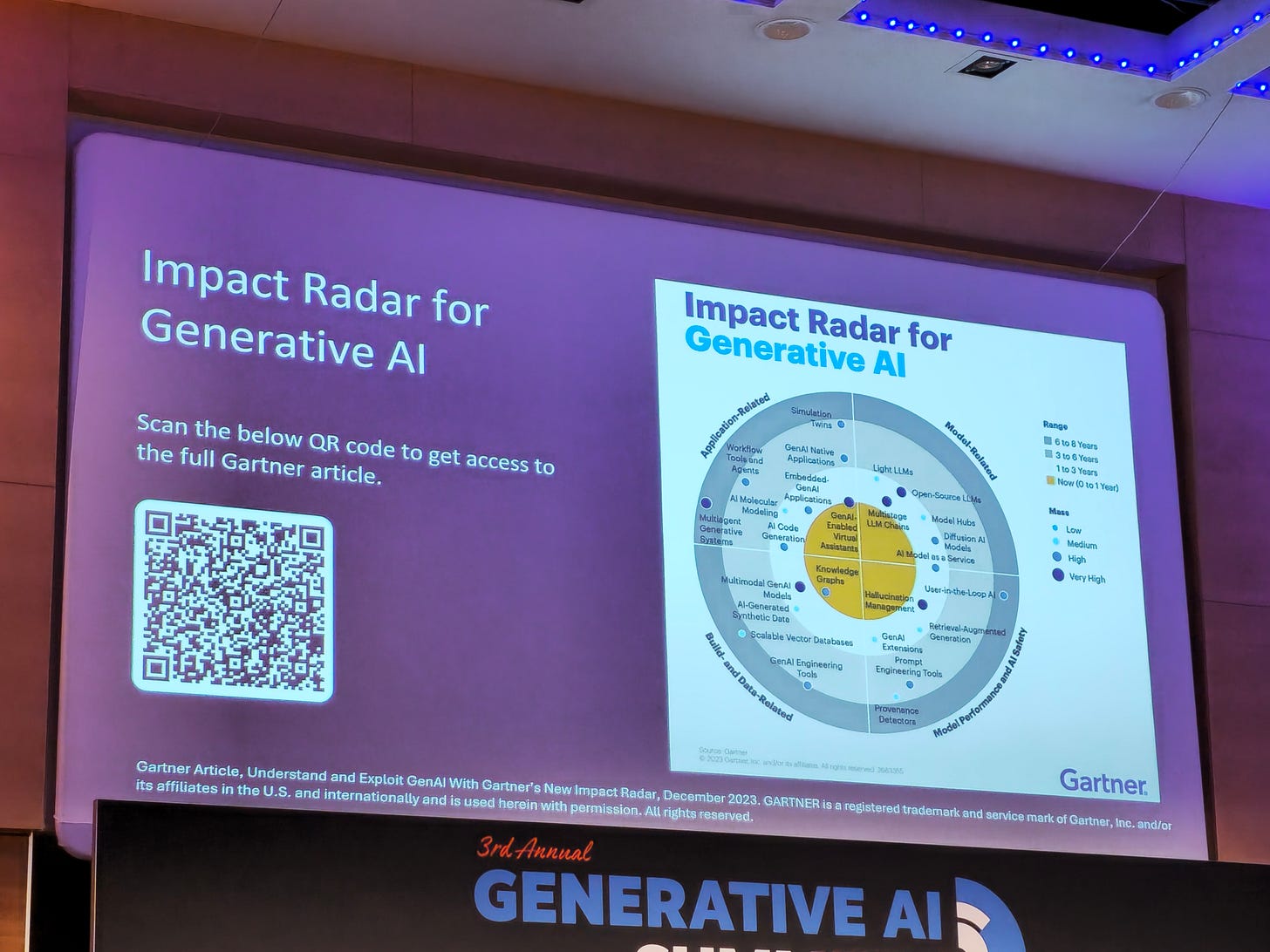
·
1 APR
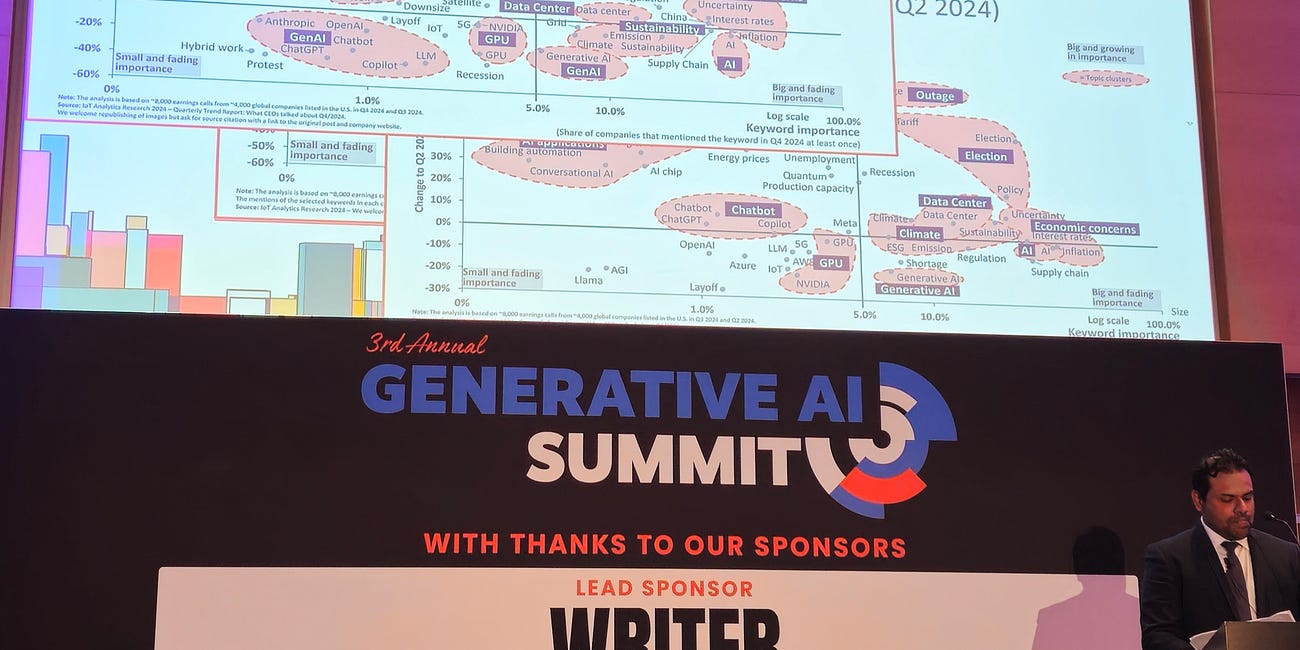