- Pascal's Chatbot Q&As
- Posts
- How organizations can integrate AI and data at the core of their business operations. This talk is structured around three case studies from the speaker's personal experience.
How organizations can integrate AI and data at the core of their business operations. This talk is structured around three case studies from the speaker's personal experience.
AI adoption is accelerated by engaging business units early and aligning AI projects with business needs. AI must be implemented within existing systems, not as isolated lab experiments.
Accelerating Data & AI Transformation Journeys
by Alexander Borek, Director of Data Analytics, Zalando
Video available here: https://p4sc4l.substack.com/p/how-organizations-can-integrate-ai
Summary:
The speaker discusses how organizations can integrate AI and data at the core of their business operations. It is structured around three case studies from the speaker's personal experience with Volkswagen Group, Volkswagen Financial Services, and Zalando, offering insights into scaling AI and data transformation.
1. Volkswagen Group (2016) – Scaling AI and Data Across a Large Enterprise
After the diesel scandal, Volkswagen sought to digitize its business at scale across 12 brands and 600,000 employees.
The company had many proof-of-concepts (POCs) but struggled to bring them into production.
Key actions taken:
Bringing together leaders across brands to collaborate.
Identifying roadblocks preventing AI and data integration.
Investing billions into three platforms:
Industry 4.0 (optimizing factories and supply chains).
Connected car (capturing vehicle data for autonomous driving and new features).
Digital sales (moving from dealership-based sales to online and omnichannel).
Establishing a Group Data Board to align strategy across brands.
Developing brand-specific but interoperable AI and data strategies.
Overcoming data access challenges through a centralized process.
Running a global training program to create internal AI and data evangelists.
Key Learnings:
AI success depends on strong data and platform foundations.
AI strategies must align with business objectives, organizational structures, and system architecture.
A clear value strategy tied to real business impact is crucial.
2. Volkswagen Financial Services – Embedding AI in Core Business Processes
Tasked with embedding AI into core processes across 18 markets within 12 months.
Started with a small team and quickly scaled up through strategic hiring.
Developed the "Data Science Safari" – a three-hour workshop to educate business units on AI and ideate use cases.
Prioritized and tested AI solutions quickly, focusing only on scalable projects.
Created four AI strategy pillars:
Customer squad (revenue growth).
Vehicle squad (profitability).
OPEX squad (cost reduction).
Mobility squad (expanding customer base).
Key projects included fully automating used car pricing globally.
Collaborated with a university for cost-effective AI model development.
Integrated AI into SAP and dealer management systems.
Key Learnings:
AI adoption is accelerated by engaging business units early and aligning AI projects with business needs.
AI must be implemented within existing systems, not as isolated lab experiments.
Successful AI integration requires cross-functional collaboration and clear business impact metrics.
3. Zalando – Organizing AI and Data at Scale
By 2020, Zalando had an explosion of decentralized AI innovation but lacked governance.
The company had:
15,000 dashboards, 60,000 reports, and hundreds of ML models without centralized oversight.
Data silos and inefficiencies across various teams.
Developed a new federated governance model, including:
A central data organization to coordinate efforts.
Use case registration and tracking of top AI dashboards.
A common data platform with rules for publishing data products.
Data certification to distinguish production-ready vs. experimental data products.
Innovations included:
Fashion Assistant (AI chatbot for personalized shopping advice).
AI-powered body measurement app (for accurate clothing sizes).
AI-driven analytics assistant (automating business insights).
Transitioned towards multi-agent AI systems to handle complex customer interactions.
Key Learnings:
AI governance is crucial to prevent chaos from uncontrolled innovation.
A structured data product model with full-time data product managersensures sustainability.
Generative AI requires new expertise (NLP, vectorized data, hardware adjustments).
Final Takeaways
AI and data transformation is challenging but achievable with clear strategy, governance, and business alignment.
AI should be integrated into the core business rather than being treated as an experimental side project.
Training, governance, and strategic investment are crucial for AI success.
The speaker ends with an invitation to the Data Masters Europe 2025 event for data leaders.
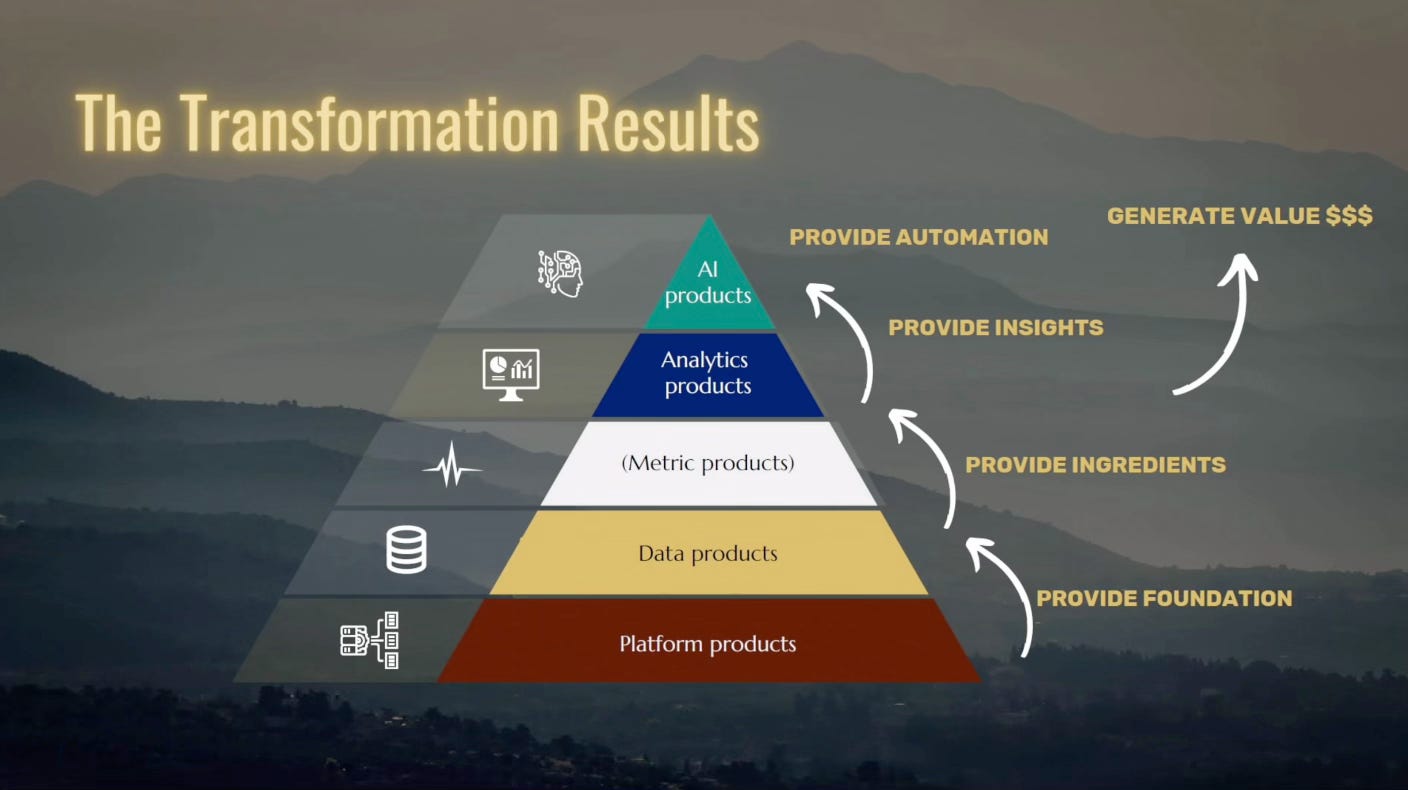
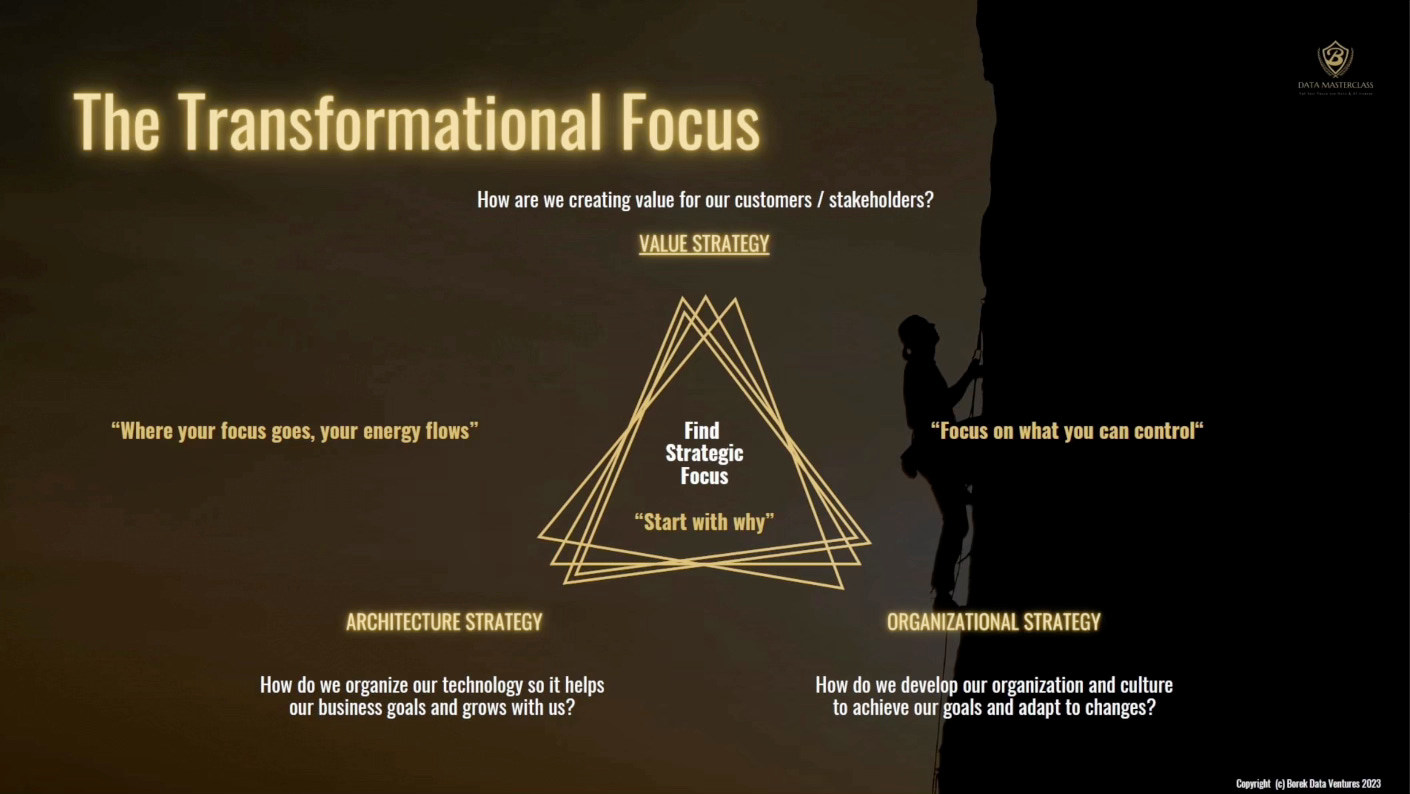
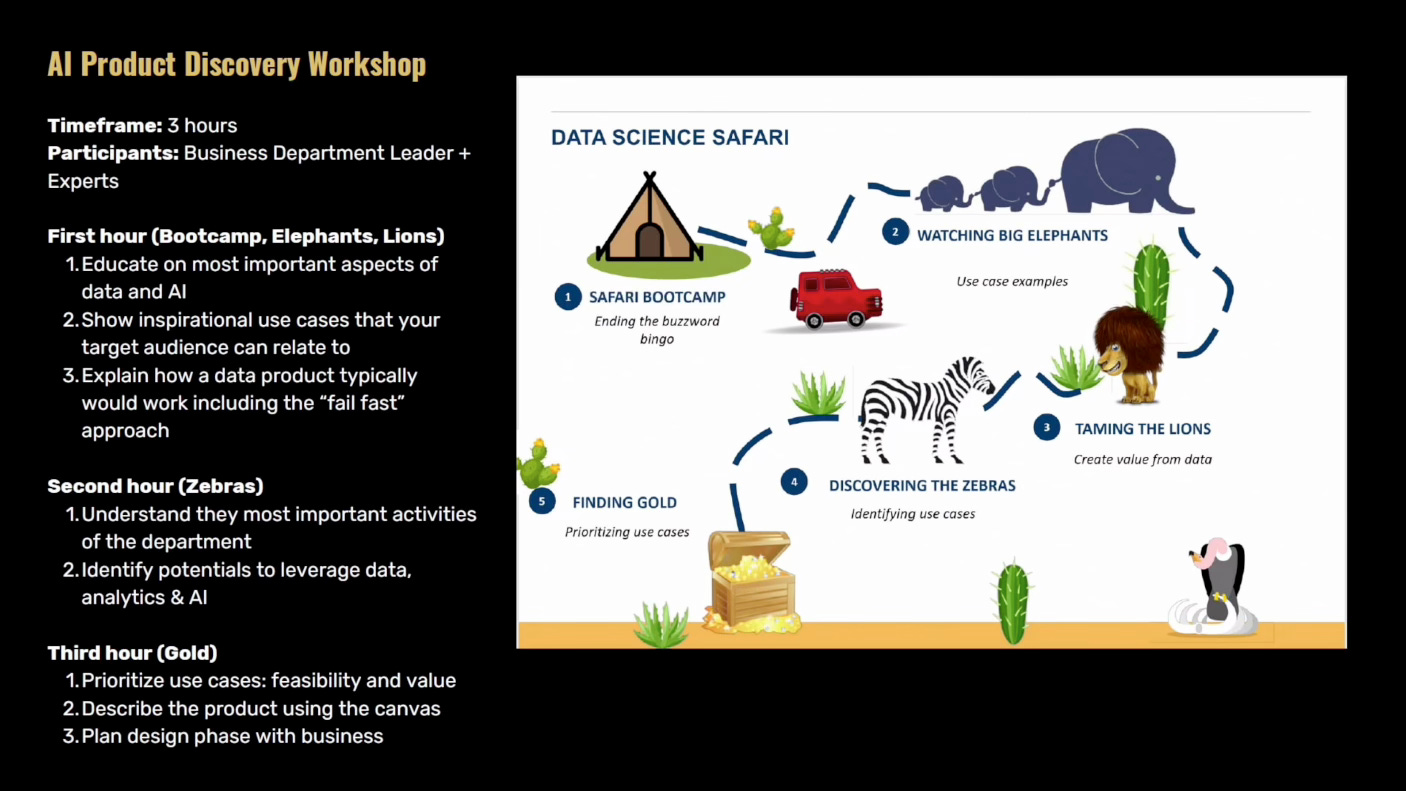
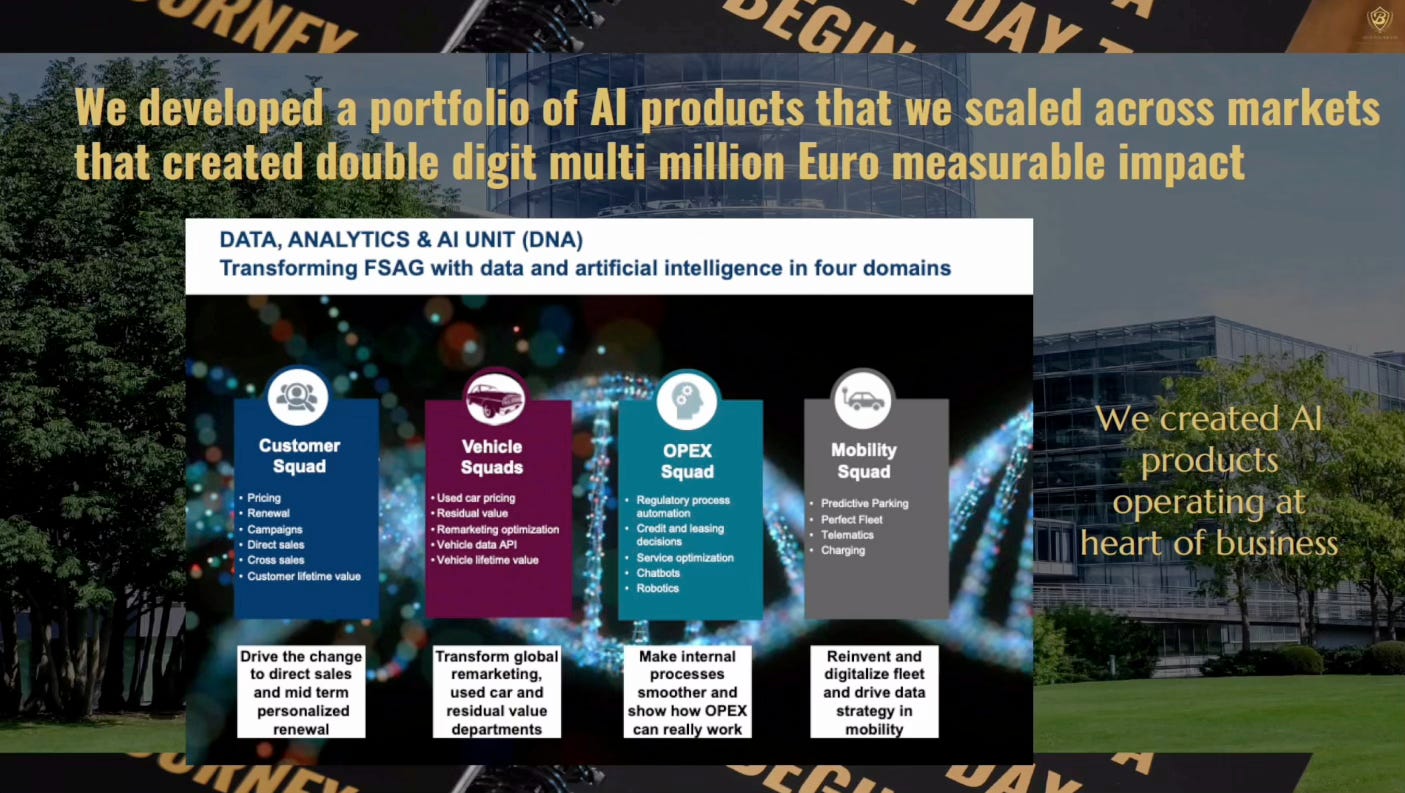
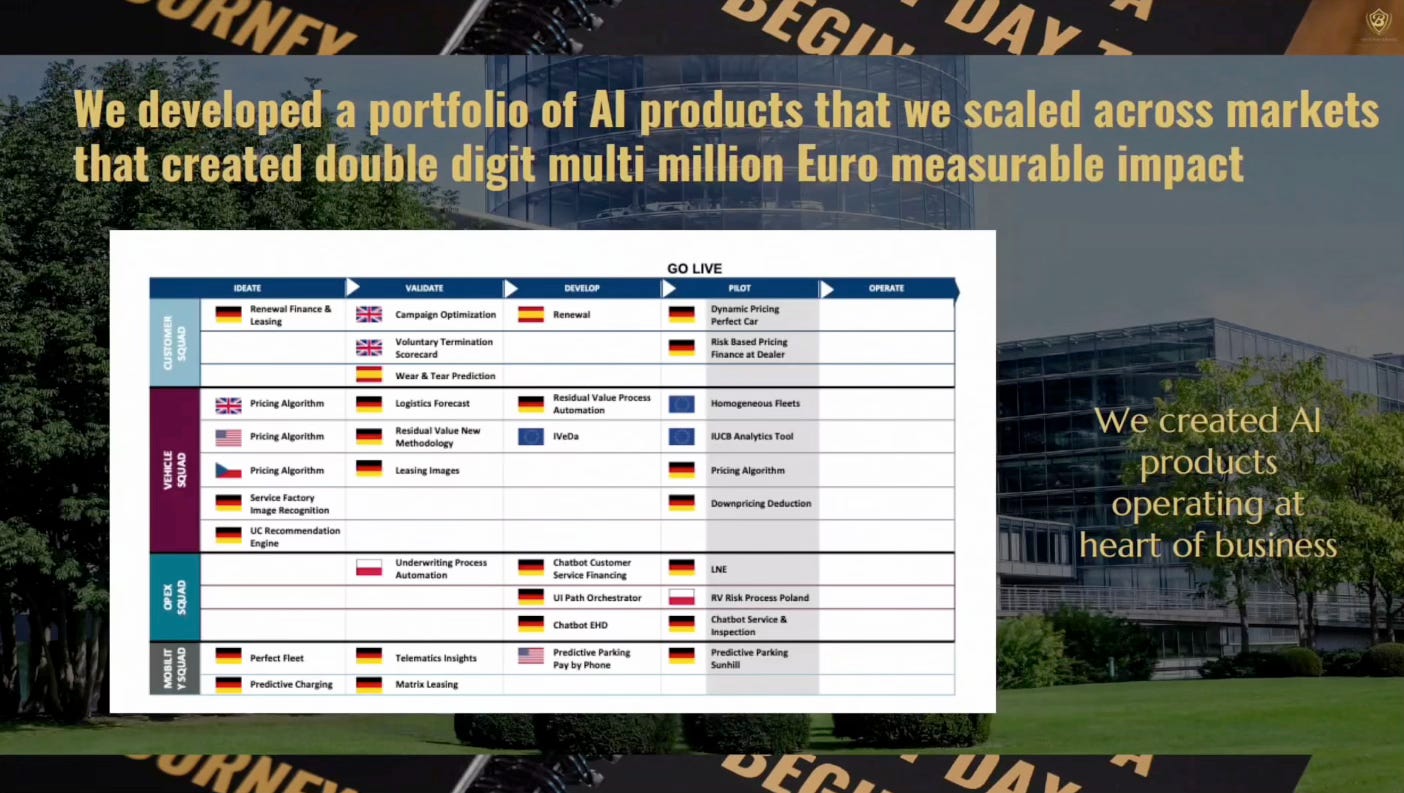
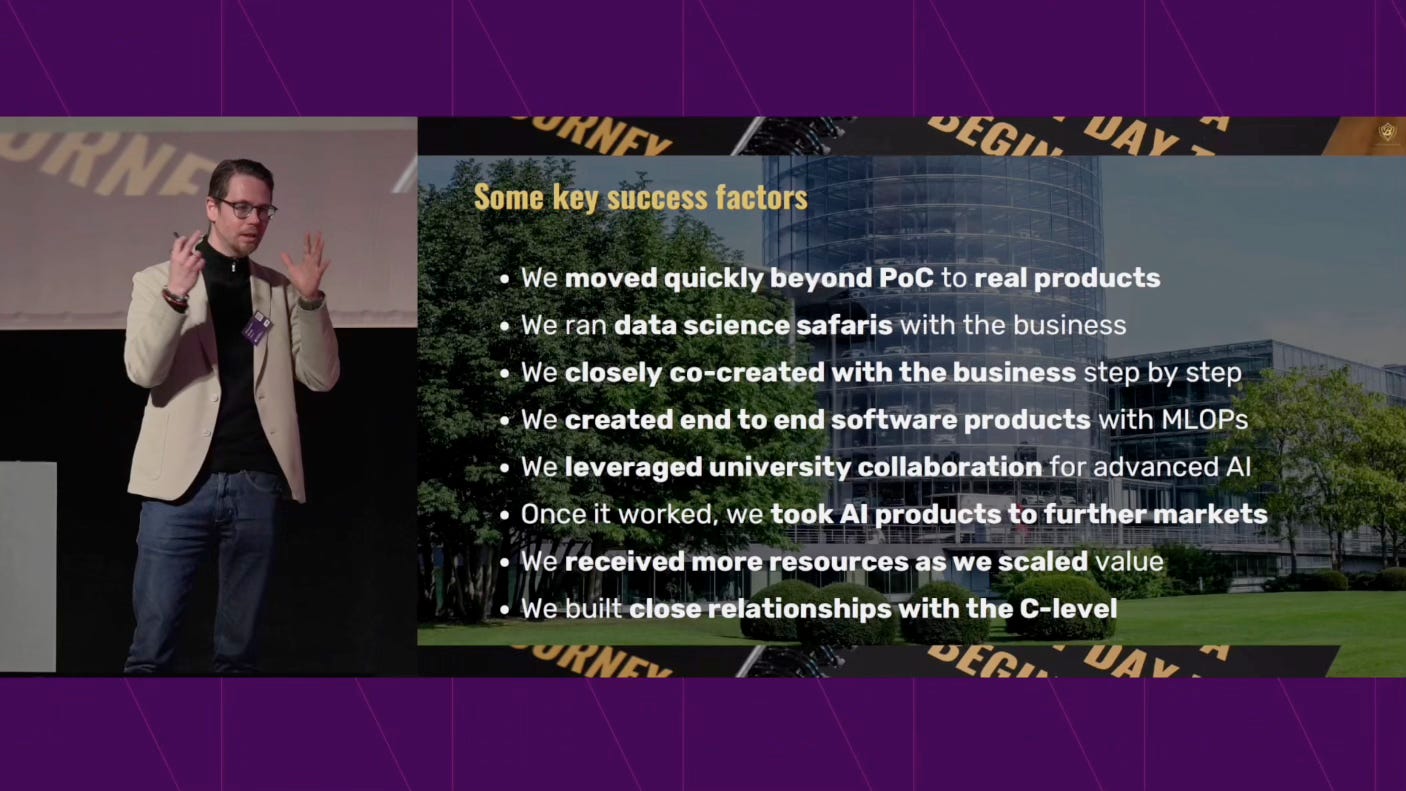
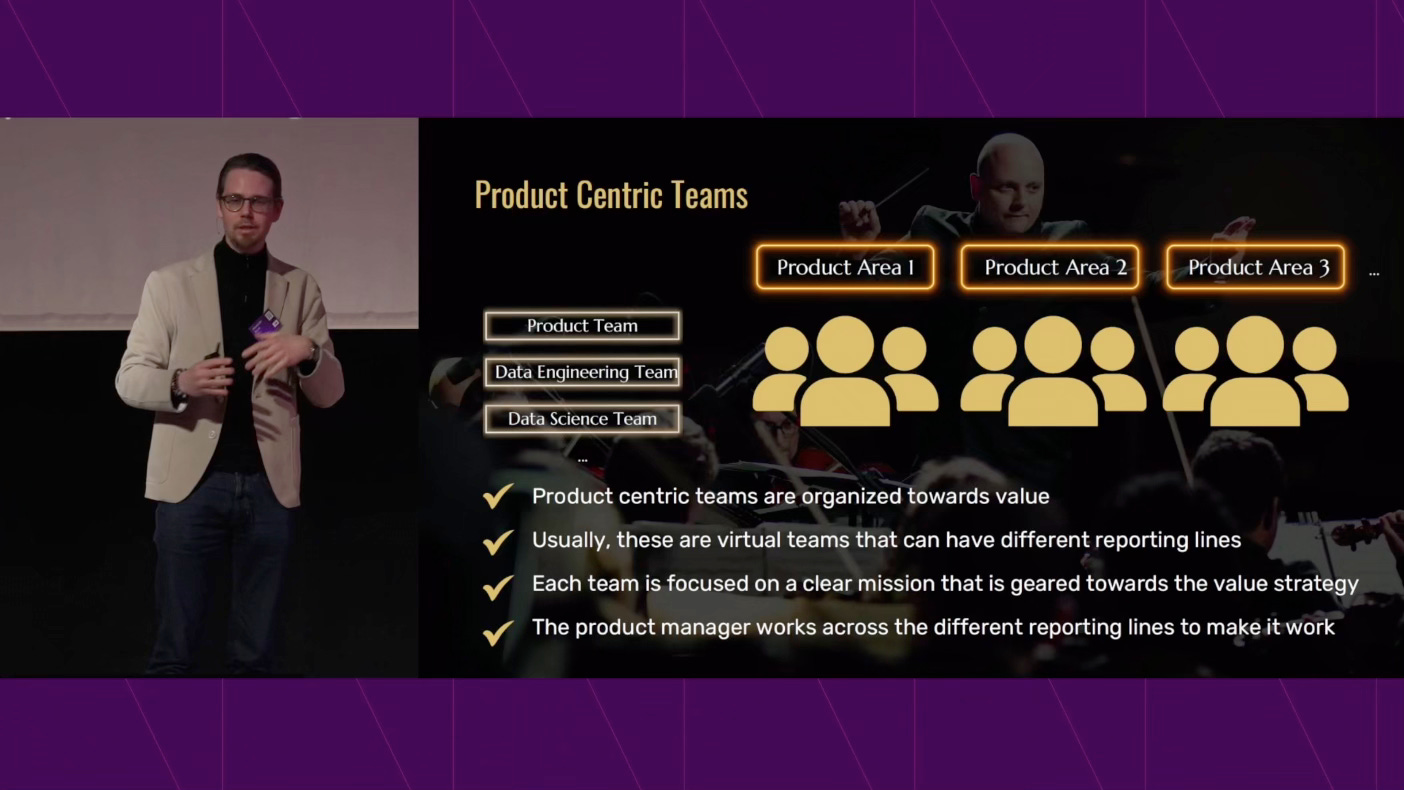
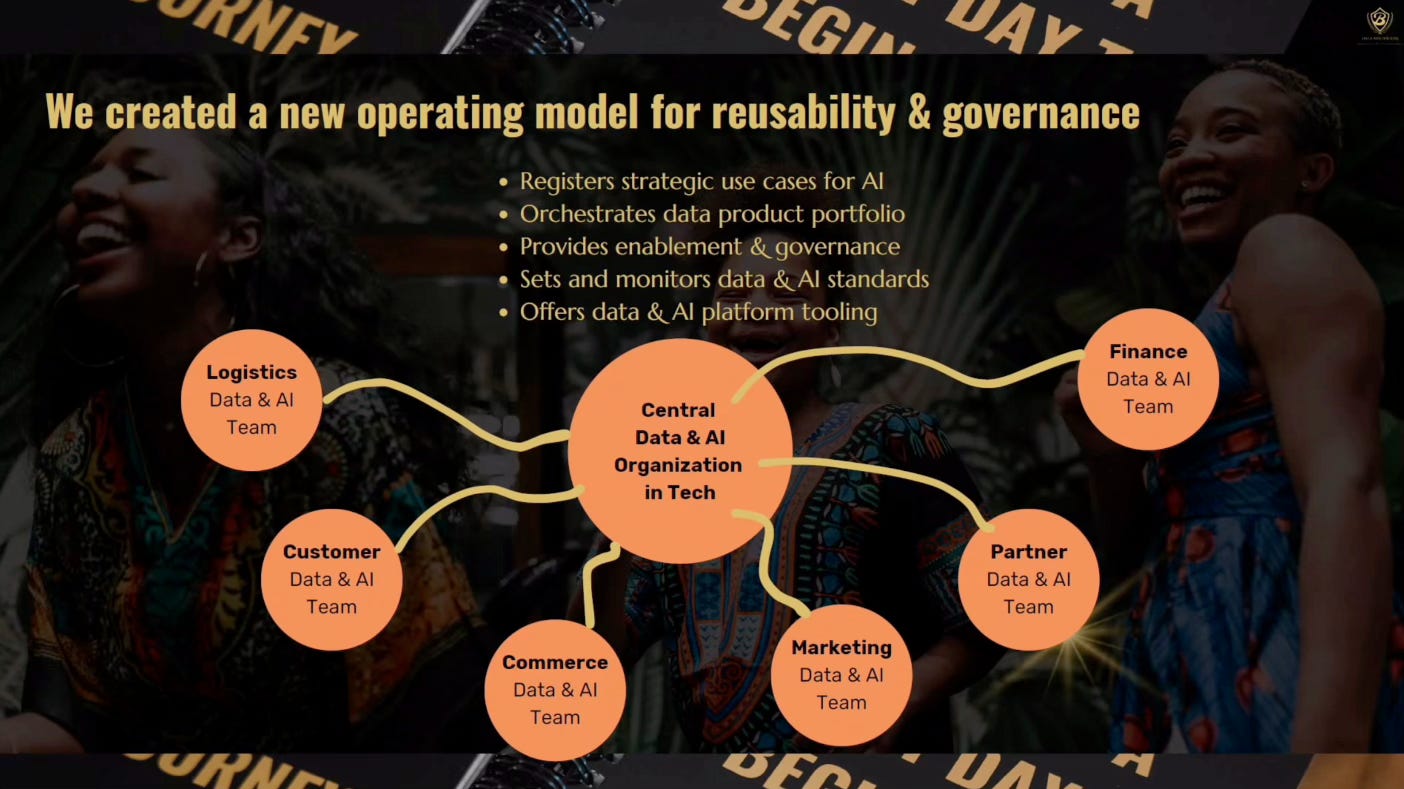