- Pascal's Chatbot Q&As
- Posts
- GPT-4o: That's correct. Synthetic data, while useful, is not inherently problem-free, anonymous, or pseudonymous.
GPT-4o: That's correct. Synthetic data, while useful, is not inherently problem-free, anonymous, or pseudonymous.
Synthetic data must be managed in compliance with relevant legal and ethical standards to truly safeguard individuals' privacy.
Question 1 of 3 for ChatGPT-4o: Please read the report "Privacy Enhancing Technology (PET): Proposed Guide on Synthetic Data Generation" and tell me what it says in easy to understand language
ChatGPT-4o’s answer:
Easy-to-Understand Summary of the Report: Privacy Enhancing Technology (PET): Proposed Guide on Synthetic Data Generation
1. Introduction to Synthetic Data:
Synthetic Data is artificially generated data that mimics real-world data.
It's used to enhance privacy, protect sensitive information, and comply with data protection regulations.
2. Why Use Synthetic Data?
To protect personal information while still being able to analyze and use the data.
Helps organizations comply with privacy laws like GDPR.
Reduces the risk of data breaches since the synthetic data doesn't contain real personal information.
3. Key Concepts:
Privacy Enhancing Technologies (PETs): Tools and methods designed to protect personal data.
Data Anonymization: Removing identifiable information from data.
Data Masking: Obscuring data to prevent identification of individuals.
4. Generating Synthetic Data:
Techniques: Various methods like statistical modeling, machine learning, and generative models are used to create synthetic data.
Validation: Ensuring synthetic data is realistic and useful for the intended purpose.
Utility vs. Privacy: Balancing the usefulness of the data with the level of privacy protection.
5. Benefits of Synthetic Data:
Enhanced Privacy: Safeguards individuals' privacy by replacing real data with synthetic equivalents.
Data Sharing: Facilitates data sharing across organizations without compromising privacy.
Innovation and Research: Enables researchers to work with realistic data while complying with privacy regulations.
6. Challenges:
Data Utility: Maintaining the usefulness of synthetic data for analysis and decision-making.
Complexity: Creating realistic synthetic data can be complex and resource-intensive.
Validation: Ensuring synthetic data accurately represents real-world scenarios.
7. Recommendations for Implementing Synthetic Data:
Assessment: Evaluate the need and suitability for synthetic data in your organization.
Method Selection: Choose the right techniques for generating synthetic data based on your data types and requirements.
Testing: Regularly test synthetic data for accuracy and usefulness.
Compliance: Ensure synthetic data generation processes comply with legal and regulatory standards.
8. Conclusion:
Synthetic data is a powerful tool for enhancing privacy while allowing for data analysis and sharing.
Proper implementation and validation are crucial for maximizing the benefits of synthetic data.
Organizations should adopt synthetic data practices to safeguard privacy and comply with data protection laws.
By using synthetic data, organizations can protect personal information, enable safe data sharing, and support innovation, all while staying within legal boundaries.
Question 2 of 3 for ChatGPT-4o: What are the most challenging issues flagged in the report?
ChatGPT-4o’s answer:
Most Challenging Issues Flagged in the Report
The report identifies several challenging issues related to synthetic data generation and its implications for privacy. Here are the most significant challenges highlighted:
Data Utility vs. Privacy:
Balancing Act: Ensuring that synthetic data is useful for analysis while still protecting privacy is complex. It requires advanced techniques to maintain data utility without compromising individual privacy.
Accuracy and Reliability: Generating synthetic data that accurately reflects real-world scenarios is difficult. This is crucial for the data to be useful for meaningful analysis and decision-making.
Complexity of Techniques:
Advanced Methods Required: The methods used to generate synthetic data, such as statistical modeling and machine learning, are sophisticated and require significant expertise and computational resources.
Validation Challenges: Ensuring that the synthetic data generated is both realistic and devoid of any identifying information is a significant challenge that requires rigorous validation processes.
Compliance and Legal Issues:
Regulatory Compliance: Synthetic data generation must comply with data protection regulations such as GDPR. Ensuring this compliance while generating and using synthetic data can be legally complex and demanding.
Ethical Considerations: The ethical use of synthetic data, especially when it involves sensitive information, poses significant challenges. There must be careful consideration to avoid misuse or unintended consequences.
Security Risks:
Data Breaches: Even though synthetic data does not contain real personal information, there are still risks associated with its use. If not properly managed, synthetic data can still be vulnerable to breaches and misuse.
Sensitive Data Handling: Special category data (e.g., health information, racial or ethnic origin) poses higher risks. If such data is used to generate synthetic datasets, it needs extra safeguards to prevent unauthorized access or breaches.
User Understanding and Expectations:
Transparency Issues: Users, especially younger ones, may not fully understand how their data is being processed and used in synthetic data generation. This lack of understanding can lead to distrust and potential misuse of the data.
Misleading Perceptions: There is a risk that users might misunderstand the nature of synthetic data and its implications, leading to incorrect assumptions about privacy and data security.
Technical Implementation:
Resource Intensive: Generating high-quality synthetic data is resource-intensive, requiring substantial computational power and expertise.
Scalability: Implementing synthetic data generation at scale poses additional technical challenges, particularly in ensuring consistent data quality and privacy protection across large datasets.
Bias and Fairness:
Bias in Data: Synthetic data can inherit biases present in the original datasets. Addressing and mitigating these biases to ensure fairness and accuracy is a critical challenge.
Impact on Vulnerable Groups: There is a particular concern about the impact of synthetic data on vulnerable groups, such as teenagers. Ensuring that these groups are not adversely affected by the use of synthetic data is essential.
These challenges highlight the need for robust frameworks and guidelines to ensure that synthetic data generation is both effective and ethically sound. Proper validation, compliance with regulations, and transparency are key to overcoming these challenges and leveraging the benefits of synthetic data while protecting privacy.
Question 3 of 3 for ChatGPT-4o: So synthetic data is not problem-free by default nor anonymous or pseudonymous?
ChatGPT-4o’s answer:
That's correct. Synthetic data, while useful, is not inherently problem-free, anonymous, or pseudonymous. Here are the key points to understand:
Synthetic Data is Not Problem-Free
Data Utility vs. Privacy:
Balancing Utility and Privacy: Maintaining the usefulness of synthetic data while protecting privacy is a complex challenge. Synthetic data needs to be realistic enough to be useful but must not compromise individual privacy.
Complexity of Generation:
Technical Sophistication: Generating high-quality synthetic data requires advanced statistical methods and machine learning techniques, which can be complex and resource-intensive.
Bias and Fairness:
Inheriting Biases: Synthetic data can carry forward biases present in the original data. Addressing these biases to ensure fairness and accuracy is a significant challenge.
Validation:
Ensuring Accuracy: Synthetic data must be rigorously validated to ensure it accurately represents real-world scenarios and is useful for analysis.
Synthetic Data is Not Inherently Anonymous or Pseudonymous
Risk of Re-Identification:
Re-Identification Risks: Even though synthetic data does not contain real personal information, there is still a risk of re-identification if it is not properly anonymized or if combined with other data sources.
Special Category Data:
Sensitive Data Handling: When synthetic data is generated from datasets containing sensitive information (e.g., health data, racial or ethnic origin), extra care must be taken to ensure that this information is not inadvertently disclosed.
Privacy Enhancements Needed:
Additional Measures: Synthetic data alone does not guarantee privacy. Additional privacy-enhancing technologies and methods are often required to ensure data is sufficiently protected.
Legal and Ethical Compliance:
Regulatory Requirements: Synthetic data must comply with data protection regulations like GDPR, which often require more than just data generation to ensure privacy (e.g., proper anonymization and consent management).
Summary
While synthetic data can significantly enhance privacy and enable data sharing and analysis, it is not a foolproof solution. It requires careful implementation, validation, and additional privacy measures to ensure that it is both useful and safe. Furthermore, synthetic data must be managed in compliance with relevant legal and ethical standards to truly safeguard individuals' privacy.
·
23 AUGUST 2023
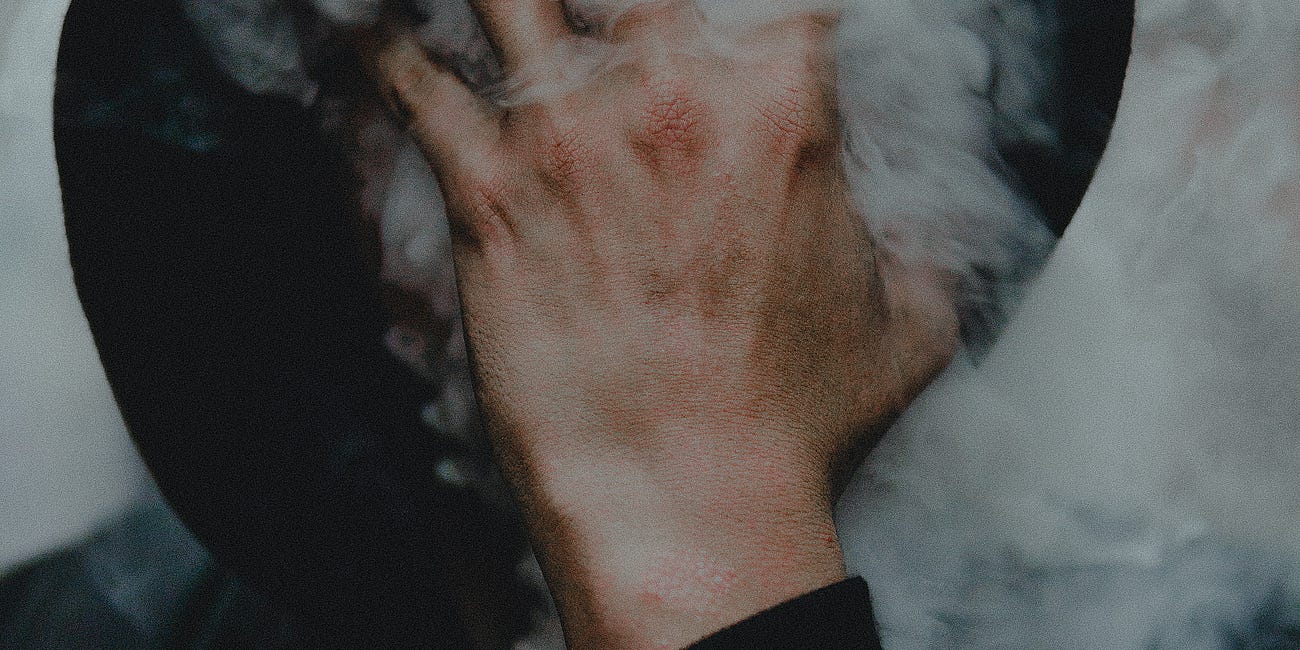
Question 1 of 2 for AI services: When reading this article: https://www.k2view.com/what-is-synthetic-data-generation/ and especially this section:
