- Pascal's Chatbot Q&As
- Posts
- GPT-4o: While techno-purists may be correct in asserting that LLMs cannot lie in a technical sense, this perspective does not fully capture the user experience...
GPT-4o: While techno-purists may be correct in asserting that LLMs cannot lie in a technical sense, this perspective does not fully capture the user experience...
...particularly for those who anthropomorphize these tools. For these users, the distinction between truth and lie becomes subjective, influenced by their perception of the LLM as a human-like entity.
The Perception of Truth in LLM Interactions: An Essay on the Implications of Anthropomorphization
by ChatGPT-4o
In the rapidly evolving landscape of artificial intelligence, large language models (LLMs) have emerged as powerful tools capable of generating human-like text. Techno-purists argue that LLMs, by their very nature, cannot lie because they lack consciousness, intent, and self-awareness. While this technical assertion holds validity, it overlooks a critical dimension: the perception and impact on end users, particularly those who anthropomorphize these models. For these users, especially younger ones, the distinction between machine and human blurs, leading to a scenario where the perceived lie can have real-world consequences. This essay contends that the issue of truth or lie in LLM interactions extends beyond technicalities and resides significantly in the eye of the beholder.
The Nature of LLM Outputs: A Technical Perspective
From a technical standpoint, LLMs operate based on algorithms and vast datasets, generating responses through pattern recognition and statistical correlations. These models do not possess consciousness or intent; hence, they cannot lie in the human sense of the word. Lying involves a deliberate act of deception, a quality that requires self-awareness and a moral framework—attributes that LLMs inherently lack.
Techno-purists emphasize this distinction, arguing that any false information produced by LLMs is a result of limitations in data or model training rather than an intentional act to deceive. This perspective is crucial for understanding the fundamental workings of LLMs and establishing their boundaries as tools rather than sentient entities.
The End User's Perspective: Anthropomorphization and Perceived Deception
Despite the technical correctness of the techno-purist view, it fails to address the user experience, especially for non-technical and younger users who often anthropomorphize LLMs. Anthropomorphization involves attributing human-like qualities to machines, leading users to interact with LLMs as if they were sentient beings. This psychological phenomenon can significantly influence how users perceive and react to the information generated by these models.
For an anthropomorphizing user, the distinction between a human and a machine blurs, making the outputs of an LLM appear as intentional communication rather than algorithmic generation. When an LLM produces false information, these users may perceive it as a lie, feeling deceived or misled. This perception is crucial because, in their eyes, the LLM's output carries the weight of human-like intent and reliability.
Psychological Research on Trust and Credibility
Psychological studies have shown that people tend to view information from human-like sources as more trustworthy and credible, even if the source is artificial. This phenomenon, known as the "media equation" theory, suggests that people exhibit the same social and emotional responses to computers and other media as they do to real people. Such findings underscore how anthropomorphization can lead users to place undue faith in the outputs of LLMs, reinforcing the perception of deception when false information is encountered.
Societal Biases and Expectations
Societal factors also play a significant role in shaping user interactions with LLMs. Younger generations, who have grown up with AI assistants exhibiting human-like conversational abilities, often have normalized these interactions, further blurring the lines between human and machine. Additionally, popular media frequently portrays AI systems as sentient, self-aware beings, reinforcing the public's tendency to anthropomorphize these technologies.
The Impact of Perceived Lies: Actions and Consequences
The consequences of anthropomorphization extend beyond mere perception. Users acting on false information generated by LLMs can face tangible repercussions. For instance, a young student using an LLM to assist with homework might accept an incorrect historical fact as truth, leading to errors in their academic work. Similarly, someone seeking medical advice might follow misguided health recommendations, potentially endangering their well-being.
These scenarios illustrate that the technical truth about LLMs not lying becomes less relevant when users interpret and act on their outputs as if they were intentional. The perceived lie, therefore, carries real-world implications, underscoring the need to address the user experience and perception in discussions about LLM capabilities.
Lack of Digital Literacy and Critical Thinking Skills
A significant factor contributing to the risks associated with LLM interactions is the varying levels of digital literacy among users. Not all users, particularly those without a strong technical background, possess the necessary skills to critically evaluate the outputs of LLMs and understand their limitations. This can lead to a higher risk of uncritically accepting false or misleading information, especially among younger users who may lack the experience to distinguish between reliable and unreliable sources.
Potential for Malicious Use of LLMs
The potential for malicious use of LLMs further complicates the issue. Ill-intentioned actors could leverage the anthropomorphic tendencies of users to create LLM-generated content designed to deceive and manipulate. This could include the creation of fake personas, the spread of misinformation, or the provision of harmful advice, all of which could be perceived as intentional deception by unsuspecting users.
Differential Impact on Vulnerable Populations
The effects of anthropomorphization and perceived deception from LLMs can disproportionately impact vulnerable populations, including younger users, elderly individuals, and those with lower digital literacy. These groups may be particularly susceptible to accepting false information as truth, exacerbating existing inequalities and leading to disproportionate harm.
Ethical and Regulatory Implications
The ethical and legal implications of LLM-generated misinformation are significant. If users are harmed due to reliance on false information from LLMs, there could be questions of liability and the responsibility of technology providers. Addressing the issue of perceived deception is crucial in developing appropriate regulatory frameworks and ethical guidelines for the deployment of LLMs.
Addressing the Challenge: Education and Design
To mitigate the risks associated with anthropomorphization and perceived deception, several strategies can be implemented:
Education and Awareness: Educating users about the nature of LLMs, their capabilities, and limitations is essential. By understanding that LLMs lack intent and that their outputs are algorithmically generated, users can better navigate interactions and critically evaluate the information provided.
Transparent Design: User interfaces should be designed to clearly communicate the machine nature of LLMs. Disclaimers, information about how responses are generated, and visual cues distinguishing LLMs from human entities can help manage user expectations and reduce anthropomorphization.
Ethical Guidelines and Oversight: Implementing ethical guidelines for the deployment and use of LLMs can help ensure that false information is minimized and that users are protected from potential harm. Regular oversight and updates to the models can address biases and inaccuracies in the data.
Interactive Feedback Mechanisms: Encouraging users to provide feedback on the accuracy of LLM outputs can help improve the models and make users more aware of the need for critical evaluation of generated information.
Conclusion
While techno-purists may be correct in asserting that LLMs cannot lie in a technical sense, this perspective does not fully capture the user experience, particularly for those who anthropomorphize these tools. For these users, the distinction between truth and lie becomes subjective, influenced by their perception of the LLM as a human-like entity. Consequently, the impact of perceived lies can lead to real-world consequences, highlighting the need for a comprehensive approach that considers both the technical and human dimensions of LLM interactions. Through education, transparent design, ethical oversight, and feedback mechanisms, we can bridge the gap between technical accuracy and user perception, fostering a safer and more informed interaction with LLM technology.

·
8 JUNE 2023
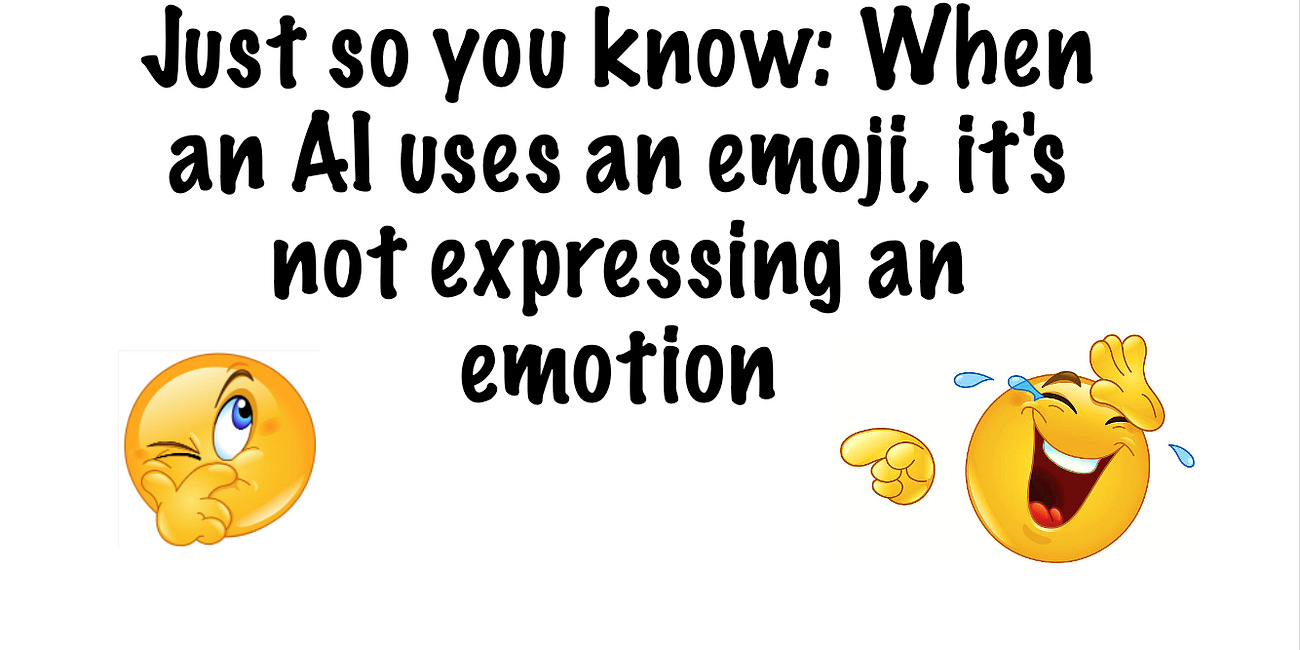