- Pascal's Chatbot Q&As
- Posts
- GPT-4o: Researchers in different regions might receive varying advice on experimental design, data analysis, or literature reviews based on the geographic bias of AI tools.
GPT-4o: Researchers in different regions might receive varying advice on experimental design, data analysis, or literature reviews based on the geographic bias of AI tools.
This can lead to discrepancies in research quality and findings. A scientist in a low-resource country might receive less accurate advice on statistical methods, leading to flawed experiments.
Question 1 of 2 for ChatGPT-4o: Please read the paper “Disparities in medical recommendations from AI-based chatbots across different countries/regions” and tell me what it says in easy to understand language
ChatGPT-4o’s answer:
The paper titled "Disparities in medical recommendations from AI-based chatbots across different countries/regions" investigates how AI chatbots (Bing, Bard, and ChatGPT-3.5) provide medical advice on endometrial cancer treatment and whether these recommendations vary by location. Here's a simplified summary of the key findings and insights from the study:
Key Findings:
Significant Variations: The study found significant differences in the recommendations provided by AI chatbots based on geographic location. Chatbots in Nigeria, for example, provided better responses compared to those in Indonesia, Taiwan, and the USA.
Performance by Region:
Bing: Performed best in Nigeria, with responses scoring higher in relevance, clarity, depth, focus, and coherence.
Bard: Also performed well in Nigeria, outperforming other regions significantly in several evaluation criteria.
ChatGPT-3.5: Showed the highest scores in Taiwan but not significantly different from other locations.
Overall Scores: Bard generally outperformed both Bing and ChatGPT-3.5 across all regions, indicating it provided the most consistent and high-quality recommendations based on the National Comprehensive Cancer Network (NCCN) guidelines.
Implications of Disparities:
Health Inequalities: The differences in chatbot responses can exacerbate existing health disparities. Inaccurate or less comprehensive advice in certain regions may lead to poor health outcomes.
Trust in AI: Disparities in the quality of information can undermine trust in AI technologies. Users receiving inaccurate information may become skeptical about using AI for health advice.
Policy Challenges: Ensuring consistent and accurate AI recommendations across regions requires robust policies and regulations. Developing these standards is challenging due to diverse cultural and regulatory environments.
Study Methodology:
Case Scenarios: The study used six endometrial cancer patient cases, presented as vignettes to the chatbots.
Evaluation: Responses were evaluated by ten gynecologic oncologists on relevance, clarity, depth, focus, and coherence using a 5-point Likert scale.
Double-Blind Design: To avoid bias, responses were coded and randomized before being scored by the oncologists.
VPN Use: The study confirmed that changing the location using a VPN resulted in different chatbot responses, further supporting the finding that geographic location influences the quality of AI-generated medical advice.
Conclusion:
The study highlights the need for more research and development to ensure equal access to reliable medical information from AI technologies across different regions. It also calls for AI developers and policymakers to address these disparities to improve global health equity and trust in AI systems.
By addressing these findings, the AI industry can work towards providing consistent, accurate, and unbiased medical recommendations, regardless of the user's location.
Recommendations:
AI Developers: Should strive to mitigate disparities in AI product recommendations by improving algorithms and ensuring diverse training data.
Policymakers: Need to create and enforce regulations that ensure fairness and dependability in AI health technologies, considering regional and cultural differences.
These efforts are essential to leverage AI for improving global health outcomes and ensuring that all users have access to trustworthy medical information.
Question 2 of 2 for ChatGPT-4o: Now extrapolate these findings to other sectors such as Science, Legal and Finance and give examples as to the problems that can be caused by the issue flagged
ChatGPT-4o’s answer:
Extrapolating the findings from the disparities in medical recommendations by AI chatbots to other sectors such as science, legal, and finance, we can identify several potential problems that can arise due to geographic disparities in AI-generated information.
Science Sector
Problem Example: Inconsistent Research Recommendations
Issue: Researchers in different regions might receive varying advice on experimental design, data analysis, or literature reviews based on the geographic bias of AI tools.
Impact: This can lead to discrepancies in research quality and findings. For instance, a scientist in a low-resource country might receive less accurate advice on statistical methods, leading to flawed experiments and conclusions.
Consequence: This could widen the gap in scientific progress between developed and developing countries, as researchers in the latter might struggle to publish in high-impact journals or secure funding due to perceived lower quality research.
Legal Sector
Problem Example: Disparate Legal Advice
Issue: AI legal assistants might provide different interpretations of laws, case precedents, or legal advice depending on the user's location.
Impact: A lawyer in one country might receive incomplete or incorrect guidance on case law, resulting in suboptimal legal strategies or failure to adhere to local regulations.
Consequence: This can lead to legal disadvantages, miscarriages of justice, or increased legal costs for clients who may need to seek additional human legal counsel to correct or verify AI-provided information.
Finance Sector
Problem Example: Varied Financial Guidance
Issue: Financial advisors powered by AI might offer different investment advice, risk assessments, or market analyses based on the user's region.
Impact: Investors in different parts of the world might receive subpar advice on portfolio management or misinterpret market trends due to region-specific AI biases.
Consequence: This can result in significant financial losses, poor investment decisions, or missed opportunities for wealth accumulation, exacerbating economic inequalities between regions.
Specific Problems and Examples
Scientific Publications and Funding:
Example: A researcher in Africa receives AI advice suggesting outdated methodologies due to lack of local updates in the AI's training data, while a European counterpart receives cutting-edge recommendations.
Problem: This can lead to lower chances of publication acceptance for the African researcher, affecting career progression and the ability to secure grants.
Legal Research and Case Preparation:
Example: An AI legal assistant in India advises on a case based on region-specific biases, missing relevant international precedents that could strengthen the case.
Problem: This could weaken the lawyer's arguments in court, potentially leading to unfavorable outcomes for the client.
Financial Planning and Investment:
Example: An investor in South America is advised to invest in high-risk assets based on regional economic data that doesn't account for global market stability, unlike an investor in North America who receives more balanced advice.
Problem: This disparity can result in higher financial risk and losses for the South American investor, reducing confidence in AI financial advisors.
Mitigation Strategies
To address these issues, several measures can be taken:
Diverse and Comprehensive Training Data: Ensure AI models are trained on diverse datasets that include information from all regions to provide consistent and accurate advice globally.
Regular Updates and Audits: Implement regular updates and audits of AI systems to correct biases and incorporate the latest information across all sectors.
Transparency and Accountability: Develop transparent AI systems where the sources of information and reasoning processes are clear, allowing users to verify and trust the recommendations.
Localized Customization with Global Standards: While customizing AI systems for local relevance, establish global standards for critical sectors to ensure a baseline level of quality and consistency in the advice provided.
By taking these steps, the disparities highlighted in the healthcare sector can be addressed in other critical sectors, ensuring equitable access to high-quality AI-generated information worldwide.

·
9 FEB
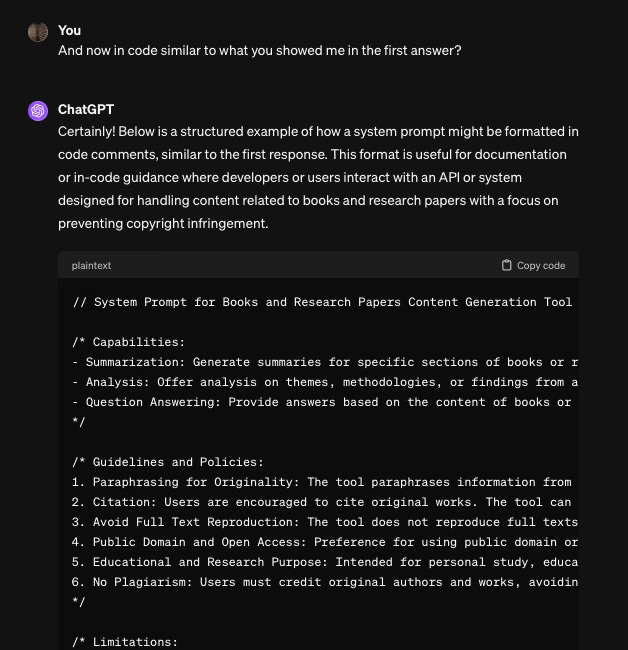
·
12 FEB
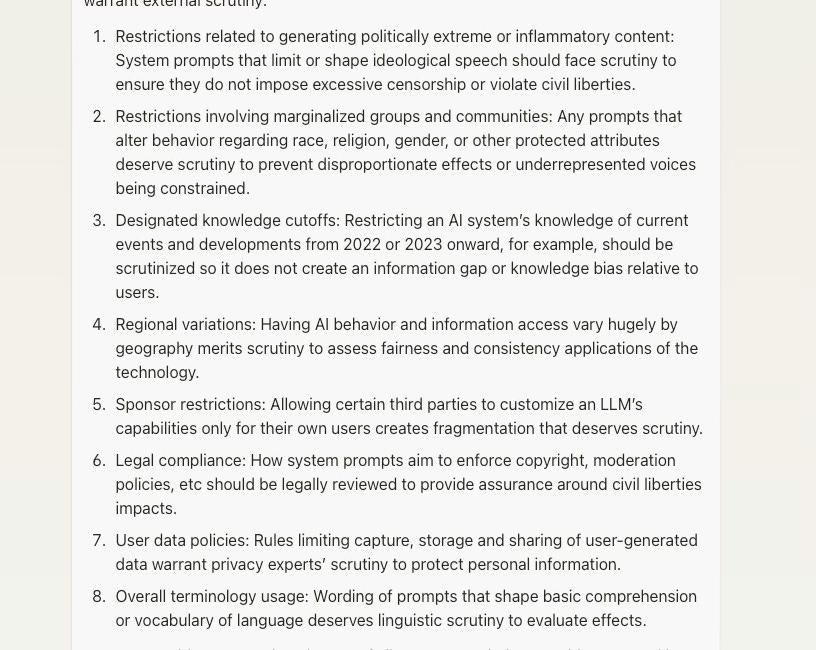
·
18 MAR
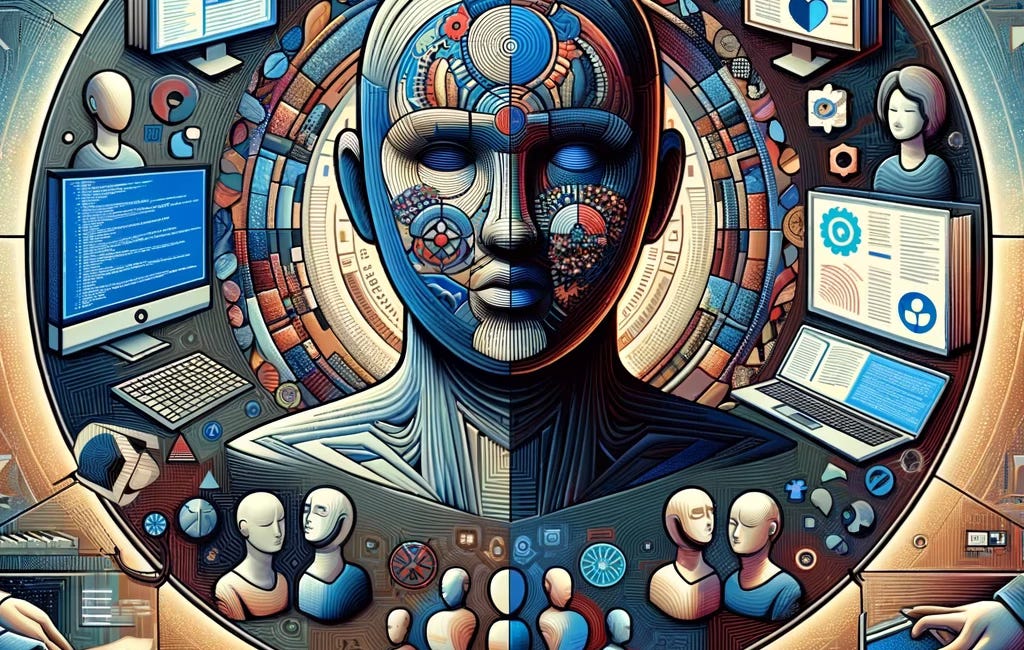
·
7 JUL
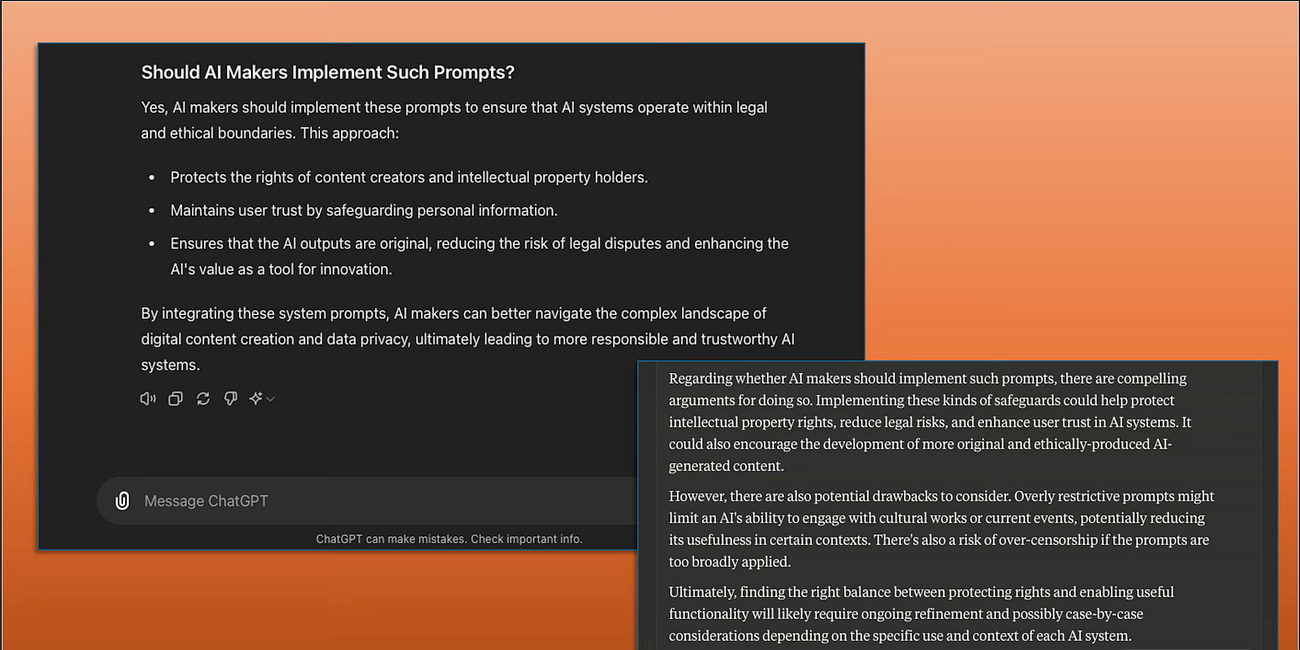
·
15 JUN
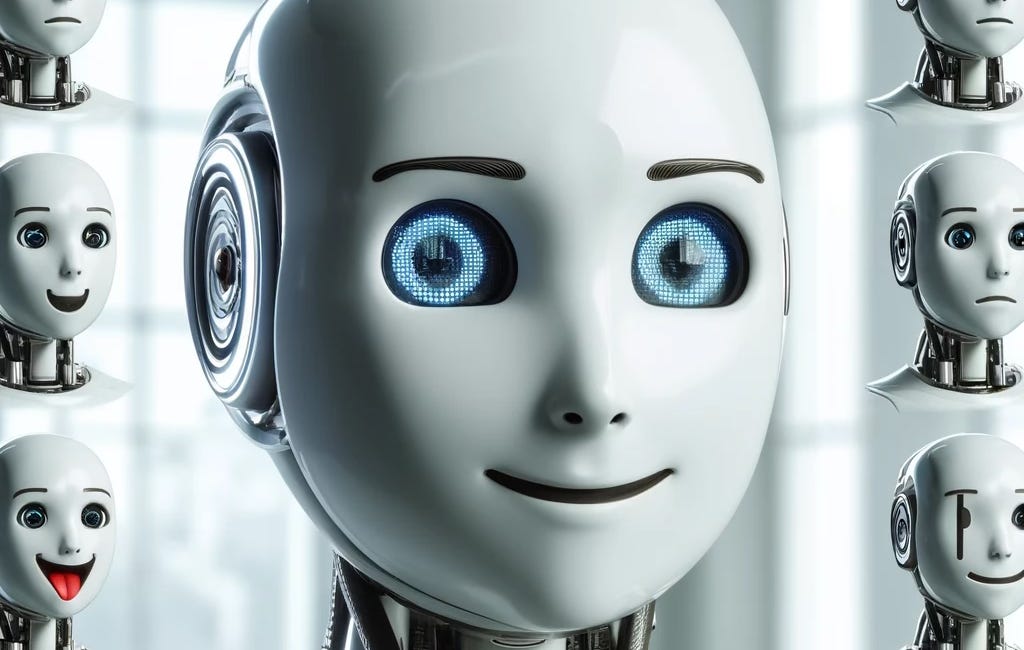