- Pascal's Chatbot Q&As
- Posts
- GPT-4o: The paper argues that generative AI’s use of copyrighted materials in its training process does not meet the standards of fair use...
GPT-4o: The paper argues that generative AI’s use of copyrighted materials in its training process does not meet the standards of fair use...
...as it capitalizes on the original works' expressive content rather than using them for functional, non-expressive purposes. Overall, I agree with the author.
Question 1 of 3 for ChatGPT-4o: please read the paper “Generative AI's Illusory Case for Fair Use” and tell me what it says in easy to understand language
ChatGPT-4o’s answer:
The paper “Generative AI's Illusory Case for Fair Use” by Jacqueline C. Charlesworth critiques the claim that generative AI companies' unauthorized reproduction of copyrighted materials for training purposes qualifies as fair use under U.S. copyright law. Here's a simplified breakdown of the key points:
AI Companies' Argument for Fair Use: AI companies argue that using copyrighted works to train AI models should be considered fair use because the models supposedly learn only uncopyrightable information, such as patterns, ideas, and relationships, not the actual expressive content of the works. They also claim that once the AI model is trained, it no longer relies on the original works to generate new content.
Challenges to the Fair Use Argument: The paper argues that AI systems don't actually discard the copyrighted works after training. Instead, they encode the works in a way that allows them to generate outputs based on those original works. AI models, particularly large language models and image generators, rely on the encoded information from the copyrighted material to produce new content, which means the works are continuously exploited, not just used in a passing way.
Comparison to Previous Fair Use Cases: The AI companies point to past fair use cases involving technology (like Google Books and Sega) to justify their actions. However, the paper explains that those cases are different because they involved using copyrighted works for functional, non-expressive purposes, like indexing or ensuring compatibility. In contrast, AI models use the expressive content of the works to create new content, which goes beyond what fair use allows.
Potential Copyright Infringement: Generative AI systems sometimes produce content that closely mimics or replicates the original copyrighted works used in training. This could lead to copyright infringement, as generating copies or derivatives of the original works without permission violates copyright law.
Conclusion: The paper concludes that the argument for fair use by AI companies is flawed. The copying involved in AI training is not merely functional or transformative in a way that fair use would protect. Instead, it exploits the copyrighted works' expressive value, which doesn't align with the fair use precedent set by courts.
In summary, the paper argues that generative AI’s use of copyrighted materials in its training process does not meet the standards of fair use, as it capitalizes on the original works' expressive content rather than using them for functional, non-expressive purposes.
Question 2 of 3 for ChatGPT-4o: What are the most controversial, surprising and valuable statements made in the paper?
ChatGPT-4o’s answer:
Here are some of the most controversial, surprising, and valuable statements made in the paper:
Controversial Statements:
Generative AI Does Not Qualify as Fair Use: The paper outright challenges the AI industry's widespread claim that the mass reproduction of copyrighted works for AI training qualifies as fair use. This is controversial because it directly disputes the positions of major AI companies and many legal scholars, who argue that AI training is "transformative" and falls under fair use.
AI Systems Exploit Expressive Content: The paper argues that AI models don’t just use uncopyrightable data (like ideas or patterns), but instead continue to exploit the expressive content of the copyrighted works they are trained on. This claim is controversial because it shifts the focus away from the AI industry's portrayal of AI systems as just using factual data, suggesting that they are actually engaged in an ongoing, infringing use of expressive content.
AI’s Memorization Problem is More Than a Bug: The paper disputes the argument from AI developers that the ability of AI models to "memorize" and replicate training data is a rare bug. Instead, it claims that the system’s ability to reproduce copyrighted works is inherent and frequent, making AI training itself infringing. This contradicts AI developers who argue memorization is a technical glitch rather than a systemic issue.
Surprising Statements:
AI Models Retain and Use Encoded Content: A key surprising point is the claim that AI models do not simply discard the copyrighted works after training but retain encoded versions of the material. This challenges the narrative that models are "just learning patterns" and not storing or using the original works in any meaningful way.
Derivative Work Argument: The paper suggests that AI models can be seen as derivative works of the training data, which implies that they are not creating something entirely new but are transforming pre-existing content. This surprising view contradicts the belief that AI output is purely original once training is complete.
Retrieval-Augmented Generation (RAG) as Infringing: Another surprising assertion is that RAG technology, where AI models retrieve and use real-time data from external sources (like the web) to generate responses, can itself be a form of copyright infringement. This pushes the boundaries of how we think about AI systems that update their knowledge in real time using unlicensed material.
Valuable Statements:
Critique of Fair Use Precedents for AI: The paper adds significant value by providing a detailed legal critique of previous fair use cases that AI companies rely on, such as Google Books and Sega. It shows that these cases involved non-expressive uses, like indexing or interoperability, and contrasts them with generative AI’s exploitation of expressive content.
Market Impact Argument: The paper emphasizes that the unauthorized use of copyrighted works to train AI models could have a severe impact on the market for creative works. This is valuable because it underscores the broader economic and cultural implications of allowing generative AI to appropriate massive amounts of copyrighted content without compensation.
Need for Licensing Models: The suggestion that a rapidly evolving market for licensing training materials exists and should be embraced by AI developers is an important takeaway. It adds value by proposing that AI companies should compensate creators, addressing both the legal and ethical gaps in current AI training practices.
In summary, the most controversial aspects of the paper directly challenge the AI industry's defense of fair use, while the surprising claims focus on how AI models retain and use copyrighted works in ways previously downplayed. The valuable insights lie in the detailed critique of fair use precedents and the paper's argument for adopting a licensing system for AI training data.
Question 3 of 3 for ChatGPT-4o: Explain whether you agree with the author and why. Feel free to use additional arguments for your views, not yet mentioned in the paper
ChatGPT-4o’s answer:
I agree with the core argument of the paper that generative AI’s use of copyrighted works for training does not easily fit within the traditional fair use framework. Here's why:
1. The "Transformative" Use Argument Falls Short
The idea of fair use is heavily tied to whether the use of copyrighted material is "transformative," meaning it adds something new with a different purpose or character, rather than simply reproducing the work. AI models don’t fit this mold as neatly as claimed by AI companies.
AI Outputs Are Based on Expressive Content: Unlike functional uses like indexing or search tools (e.g., Google Books), generative AI uses expressive works to generate new expressive content—whether text, music, or images. The AI is not just learning facts or ideas but encoding creative expressions, and its outputs are directly influenced by those inputs.
Derivative Works: As the paper suggests, AI models could be seen as derivative works of the training data. While not literal copies, the model's outputs are heavily dependent on the input, meaning the work isn’t being transformed as much as re-arranged or recombined. This view aligns with copyright law, which protects against the creation of derivative works based on copyrighted material without permission.
2. Memorization vs. Transformation
The paper’s point that AI systems inherently “memorize” and can reproduce training data is crucial. This is not merely a technical bug but a feature of how AI models operate. While proponents of AI claim that these models are generating new, original content, if they can reproduce large sections of copyrighted material verbatim or closely, then this crosses into infringement.
Human Creativity vs. AI Processing: Human creativity often involves using multiple sources of inspiration, but humans aren’t simply recombining those inputs in a mechanical way. AI systems, however, function algorithmically and can replicate those exact inputs, unlike humans who create transformative works by infusing personal, cultural, and contextual nuances. This makes AI training more analogous to copying than transforming.
3. Economic and Ethical Considerations
The market impact of AI models should not be overlooked. The paper rightly points out that the ability of AI systems to generate content in the style of existing artists, writers, or photographers poses significant risks to the original creators.
Potential Market Harm: Allowing generative AI to freely use copyrighted works without compensation could devastate creative industries. Authors, artists, and musicians rely on licensing and royalties for their livelihoods. If AI can generate content that competes directly with their work, it could undercut these markets, making it harder for original creators to sustain themselves.
Ethical Dimensions: There is an ethical argument for compensating creators whose works were used to train AI systems. Just as creators are paid when their music is sampled or their designs are used in merchandise, they should be compensated for AI’s use of their expressive content. A fair licensing system could ensure that artists retain some control over their work and receive appropriate compensation.
Additional Arguments:
AI's Misuse of Public Domain Works One argument I would add is the potential for AI companies to misuse works in the public domain. While public domain works are free to use, combining them with copyrighted materials in training datasets without transparency creates an ethical gray area. If AI systems use public domain content to mask their reliance on copyrighted materials, it distorts the purpose of the public domain and adds complexity to copyright law.
Impact on Creativity and Originality Another concern is how reliance on AI-trained models might reduce human creativity over time. If generative AI systems become common, there may be less incentive for companies to invest in human creators. This could lead to a homogenization of content—AI works often rely on patterns and established structures. While they can produce a high volume of work quickly, that work may lack the originality and unpredictability that human creativity brings.
Blurred Attribution of Authorship AI-generated content blurs the lines of authorship. Who owns the output—AI developers, the creators of the training data, or the user generating content? This lack of clarity in attribution further complicates the fair use argument. If no one can be definitively called the “creator” of a work, the copyright system, which relies on authorship, becomes destabilized.
Conclusion:
While AI companies argue that the use of copyrighted works to train AI models is transformative and therefore protected by fair use, this reasoning seems weak when considering the continued reliance on those works to generate new expressive content. AI’s capacity to “memorize” and replicate original works, the potential market harm to creators, and the broader ethical and legal implications suggest that a more cautious, rights-respecting approach—such as licensing copyrighted works for AI training—is necessary.
Overall, I agree with the author that the current justifications for AI’s use of copyrighted materials under the guise of fair use are illusory and need reevaluation in the evolving digital landscape.

·
5 SEP
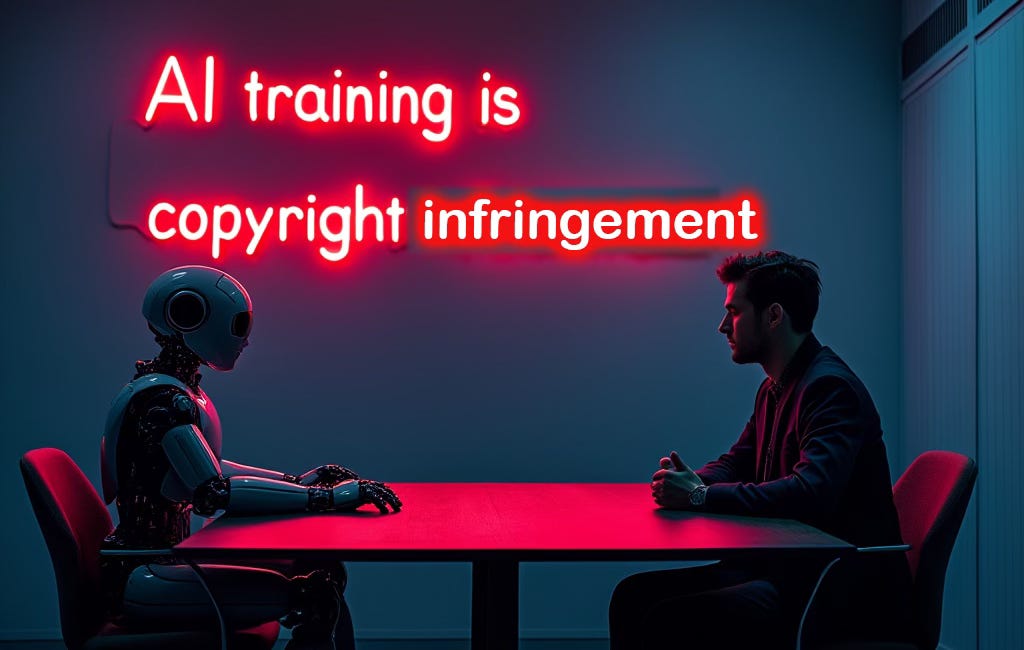
·
6 SEP
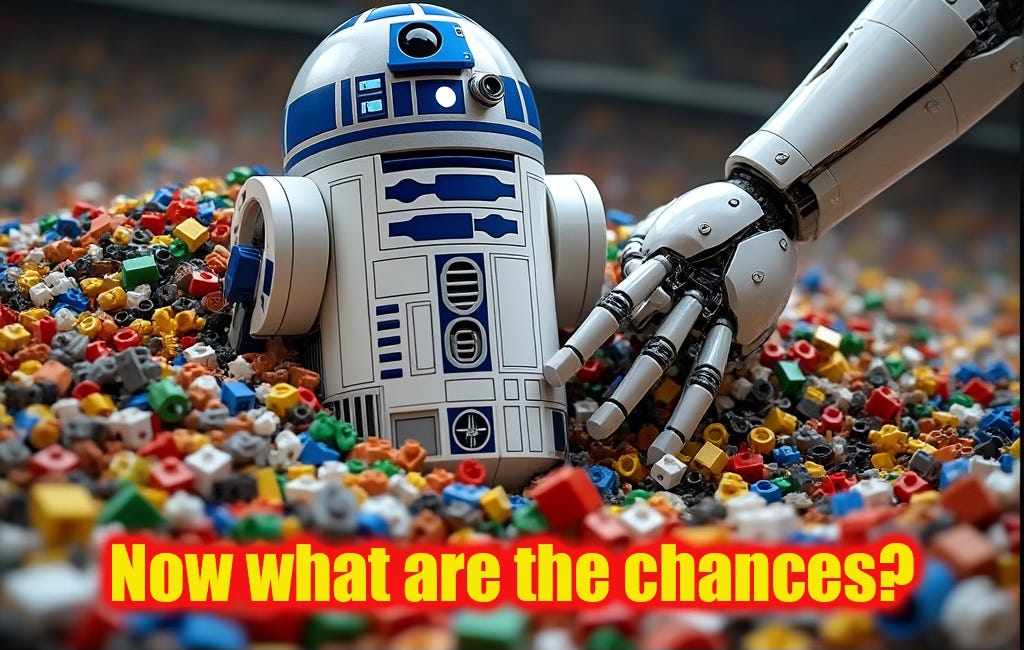