- Pascal's Chatbot Q&As
- Posts
- GPT-4o: Larger models memorize and reproduce more text from their training data, which increases the risk of copyright infringement (...) shows a direct correlation between model size and legal issues
GPT-4o: Larger models memorize and reproduce more text from their training data, which increases the risk of copyright infringement (...) shows a direct correlation between model size and legal issues
GPT-4 sometimes provided contradictory responses, such as correctly stating the publication date of a public domain book but then claiming it was copyrighted.
Question 1 of 3 for ChatGPT-4o: Please read the paper “LLMs and Memorization: On Quality and Specificity of Copyright Compliance” and tell me what it says in easy to understand language
ChatGPT-4o’s answer:
The paper "LLMs and Memorization: On Quality and Specificity of Copyright Compliance" addresses the issue of large language models (LLMs) potentially reproducing copyrighted text from their training data, which could lead to copyright violations. Here's a simplified summary of the key points:
Introduction
LLMs are trained on vast datasets, which include copyrighted material.
Reproducing copyrighted text verbatim can lead to legal issues.
The paper proposes a systematic way to analyze potential copyright infringements by LLMs, focusing on European law.
Methodology
The authors use a threshold of 160 characters, based on German copyright law, to identify potential copyright infringements.
They use a fuzzy text matching algorithm to detect both exact and slightly altered reproductions of copyrighted text.
The analysis is performed on instruction-finetuned models, which are often used in real-world applications.
They use two datasets: one of copyrighted books and another of public domain books to compare model behavior.
Results
Specificity and Compliance: Some models, like Alpaca, GPT-4, GPT-3.5, and Luminous, performed well in distinguishing between copyrighted and public domain texts.
Reproduction Rates: The study measured how often these models reproduced text longer than 160 characters from both copyrighted and public domain books.
Model Behavior: Different models showed various behaviors when faced with copyright-sensitive prompts. These included refusal to answer, hallucination (generating made-up text), or providing non-literal summaries.
Impact of Model Size: Larger models tend to memorize and reproduce more text from their training data, which increases the risk of copyright infringement.
Legal Analysis
The paper provides a legal assessment of the different behaviors of LLMs in terms of European copyright law.
Outputs containing more than 160 characters of copyrighted text are presumed to be infringing.
The study also examines the potential application of statutory exceptions like quotations and pastiches under European law.
Conclusion
The paper highlights significant differences in copyright compliance across different LLMs.
It suggests that more refined methods are needed to ensure LLMs comply with copyright laws without sacrificing their utility in practical applications.
Future work should extend the analysis to a broader range of models and more diverse datasets.
Overall, the paper emphasizes the importance of understanding and mitigating the risks of copyright violations by LLMs to ensure their legal and ethical use.
Question 2 of 3 for ChatGPT-4o: What are the most surprising, controversial, concerning and damaging findings and statements in this paper?
ChatGPT-4o’s answer:
Here are the most surprising, controversial, concerning, and damaging findings and statements from the paper "LLMs and Memorization: On Quality and Specificity of Copyright Compliance":
Surprising Findings
Small Model Performance: The Alpaca model, despite its small size, demonstrated surprisingly good copyright compliance. This was attributed to its limited memorization capabilities, which seem focused on very popular public domain texts .
High Hallucination Rates: Models from the LLama family and some European models exhibited higher rates of hallucinations compared to GPT-family models. Hallucinations mean the model generates text that seems plausible but is not actually found in the training data .
Controversial Findings
Contradictory Outputs: GPT-4 sometimes provided contradictory responses, such as correctly stating the publication date of a public domain book but then claiming it was copyrighted. This inconsistency in handling copyright information raises questions about the reliability of its outputs .
Discriminatory Training Data: The study speculated that OpenGPT-X produces fewer copyright infringements because it is presumably trained on less book material, highlighting the impact of selective training data on model behavior .
Concerning Findings
Memorization of Copyrighted Text: Larger models tend to memorize and reproduce more text from their training data, which increases the risk of copyright infringement. This is a significant concern as it shows a direct correlation between model size and potential legal issues .
False Positives and Negatives: High rates of false positives (refusing to answer even when it’s not necessary) and false negatives (failing to refuse when it should) in models like GPT-3.5 raise concerns about their practical use and the robustness of their copyright compliance measures .
Damaging Findings
Lawsuits and Legal Risks: The paper referenced ongoing lawsuits by popular authors against AI providers for using copyrighted texts in training without permission. This highlights the real-world legal risks and potential damages facing companies developing and deploying these models .
Significant Reproduction Rates: The significant reproduction rate (SRR) metric showed that some models, especially larger ones like GPT-4, had high SRR scores for copyrighted texts. This metric is used to quantify the extent of potential copyright infringements, and high scores indicate substantial legal risks .
These findings emphasize the importance of addressing copyright compliance in the development and deployment of large language models to avoid legal repercussions and ensure ethical use.
Question 3 of 3 for ChatGPT-4o: List all possible consequences of these findings for AI makers and rights owners such as publishers.
ChatGPT-4o’s answer:
The findings from the paper "LLMs and Memorization: On Quality and Specificity of Copyright Compliance" can have significant consequences for AI makers and rights owners such as publishers. Here are the possible consequences for each group:
For AI Makers:
Legal Repercussions:
Lawsuits: AI makers might face lawsuits from authors and publishers if their models are found to reproduce copyrighted material without permission.
Fines and Penalties: Non-compliance with copyright laws and regulations like the European AI Act could lead to substantial fines and penalties.
Operational Changes:
Enhanced Compliance Measures: AI makers may need to implement more stringent measures to ensure their models comply with copyright laws, such as improved filtering mechanisms and better handling of copyrighted material.
Increased Costs: Ensuring compliance could lead to higher operational costs due to the need for additional resources, such as legal consultations, compliance teams, and more sophisticated technical solutions.
Reputation and Trust:
Loss of Trust: Failure to comply with copyright laws could damage the reputation of AI makers, leading to a loss of trust among users and partners.
Market Impact: Negative publicity and legal issues could impact the market position of AI companies, potentially leading to a loss of business and market share.
Innovation and Development:
Impact on Innovation: The need to comply with stringent copyright laws might slow down innovation and development in AI, as companies will need to spend more time and resources on ensuring legal compliance.
Focus on Public Domain and Licensed Data: AI makers might increasingly focus on using public domain or properly licensed data to avoid legal issues, which could limit the scope of their models.
For Rights Owners (Publishers and Authors):
Protection of Intellectual Property:
Better Protection Mechanisms: The findings highlight the need for better mechanisms to protect copyrighted works from being used without permission, potentially leading to stronger legal frameworks and enforcement.
Revenue and Licensing:
Licensing Opportunities: There could be increased opportunities for rights owners to license their works to AI makers, creating new revenue streams.
Revenue Loss: Unauthorized use of copyrighted material by AI models could lead to revenue loss for rights owners if it replaces the need to purchase the original works.
Control Over Content:
Increased Control: Rights owners may push for more control over how their works are used in AI training datasets, leading to stricter terms and conditions for the use of their material.
Monitoring and Enforcement: Rights owners might need to invest in more robust monitoring and enforcement mechanisms to detect and address unauthorized use of their content.
Industry Collaboration:
Collaborative Efforts: The need to address copyright compliance in AI could lead to more collaboration between rights owners and AI makers to develop fair use policies and compliance frameworks that benefit both parties.
Standardization: There might be a push towards industry-wide standards for the use of copyrighted material in AI training, ensuring that all stakeholders have clear guidelines to follow.
Market Dynamics:
Competitive Advantage: Rights owners who effectively manage and license their content for use in AI could gain a competitive advantage in the market.
Litigation Risks: Increased litigation against AI makers could lead to a more contentious relationship between rights owners and the tech industry, impacting overall market dynamics.
Overall, these findings emphasize the need for both AI makers and rights owners to carefully navigate the legal and ethical landscape of using copyrighted material in AI training to avoid potential conflicts and capitalize on emerging opportunities.

·
31 JUL
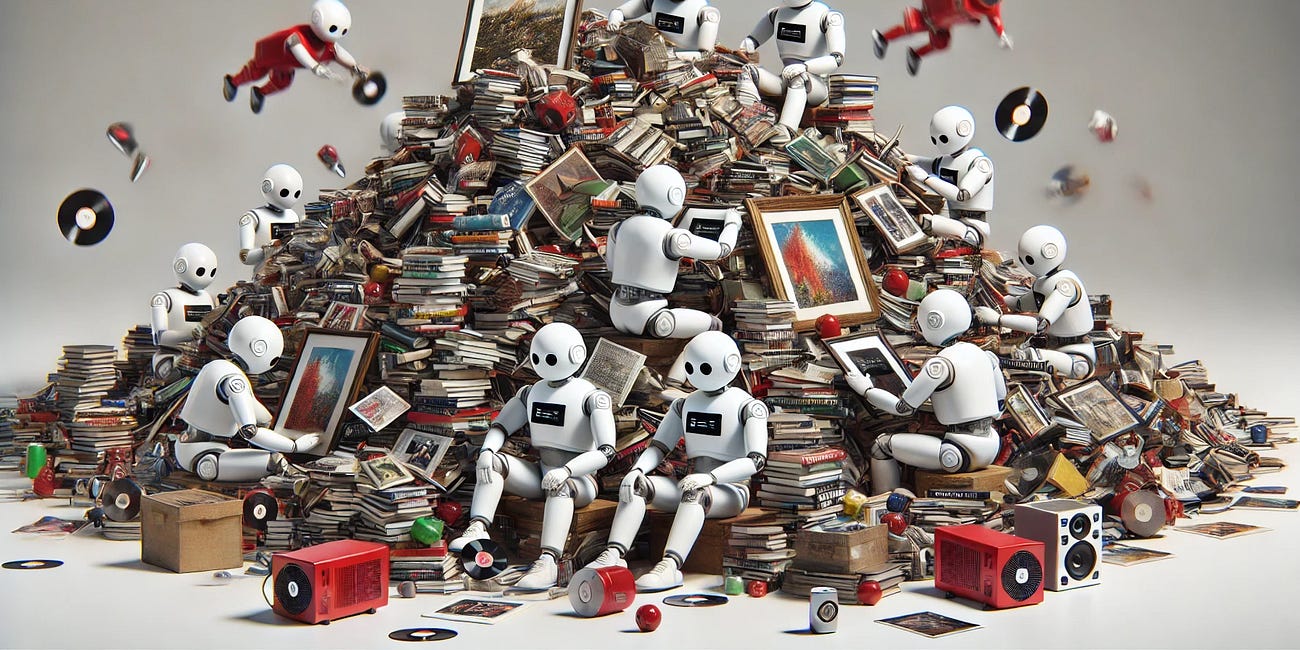
·
29 JUL
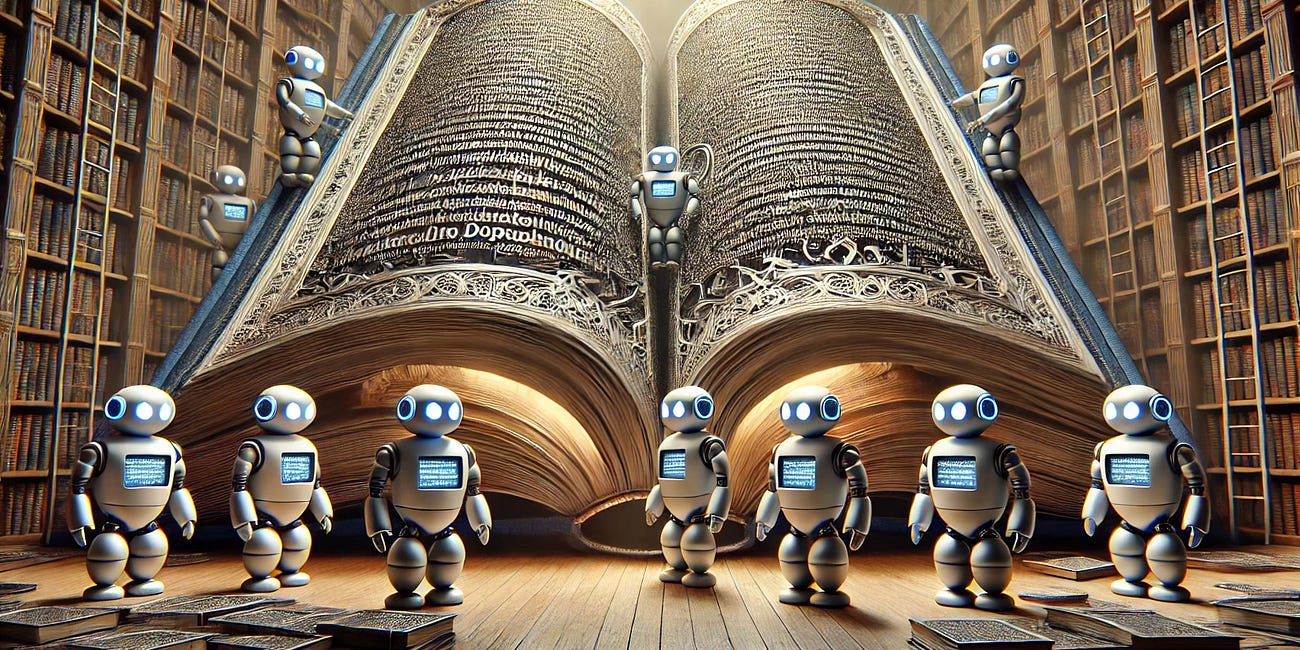