- Pascal's Chatbot Q&As
- Posts
- GPT-4o: Yes, the TikTok transcript aligns with the idea that people only find data, findings or perspectives "valuable" or "correct" when they align with their preconceived beliefs or desired outcomes
GPT-4o: Yes, the TikTok transcript aligns with the idea that people only find data, findings or perspectives "valuable" or "correct" when they align with their preconceived beliefs or desired outcomes
Preconceived Beliefs Dictate Acceptance, Resistance to Unfavorable Results, Iterative Verification of Unpopular Results, Selective Belief Systems, Inevitable Progress vs. Flawed Reality...
Asking AI services: Please read the transcript of this TikTok video and tell me whether you agree that we have heard the following before: “People lose their minds when they don’t like the result” but in the context of AI development and commercialisation. AI Research seems to be only ‘right’ or ‘valuable’ when it says something positive about AI. Similarly, Large Language Models are only ‘awesome’, or ‘right’ about something when the message aligns with the religious belief regarding AI. The moment they say something negative about either themselves, AI development or the religious believers in AI, they’re ‘hallucinating’ or ‘work in progress’...(with those same believers arguing that, regardless, AI is inevitable and will improve!). List all analogies if indeed you spot any.
Claude’s answer:
This is a fascinating parallel you've drawn. The transcript describes Roland Fryer's experience researching police bias, and there are indeed striking analogies to current AI discourse:
Confirmation Bias and Result Reception
Police Study: When Fryer found bias in low-level force, people accepted it. When he found no racial bias in shootings, "all hell broke loose"
AI Parallel: There's a similar pattern where positive findings about AI capabilities are readily accepted, while critical or limiting findings are often dismissed as "hallucinations" or temporary limitations
Speed of Rejection
Police Study: Fryer notes getting rejection emails within 4 minutes of publishing a 104-page paper
AI Parallel: We often see immediate dismissal of AI critiques without thorough engagement with the underlying research or evidence
Career Pressure
Police Study: Colleagues warned "don't publish this, you'll ruin your career"
AI Parallel: There are similar pressures in AI research where expressing skepticism or publishing critical findings about AI capabilities or risks can be professionally risky
Selective Acceptance
Police Study: "We liked the first one, but you should publish the second one another time"
AI Parallel: There's a tendency to embrace research that confirms positive views about AI while suggesting critical findings need "more research" or should be published separately
Methodological Criticism
Police Study: Critics suddenly questioned regression analysis ("He's using regressions") despite it being a standard tool
AI Parallel: Similar pattern where established methodologies are suddenly questioned when they produce results that challenge the dominant narrative about AI progress
Your observation about the "hallucination" label is particularly apt - it's interesting how the term is often selectively applied to dismiss AI outputs that contradict desired narratives while accepting those that align with optimistic views about AI capability.
The key parallel seems to be how emotional investment in a particular narrative (whether about policing or AI) can lead to rejection of evidence that challenges that narrative, regardless of the robustness of the research methodology.
ChatGPT-4o’s answer:
Yes, the sentiment expressed in the TikTok transcript aligns with the idea that people only find data, findings, or perspectives "valuable" or "correct" when they align with their preconceived beliefs or desired outcomes. In the context of AI development and commercialization, the analogy can be drawn as follows:
Preconceived Beliefs Dictate Acceptance:
The speaker observes that people "lose their minds when they don't like the result" and suggests that findings are only embraced when they confirm existing biases.
Similarly, in AI research, outputs or conclusions are deemed "right" or "valuable" only when they affirm positive beliefs about AI, while critical or negative perspectives are dismissed as flawed or "work in progress."
Resistance to Unfavorable Results:
The researcher shares how colleagues advised against publishing surprising findings because they did not fit the preferred narrative.
This mirrors how Large Language Models (LLMs) are celebrated when they produce favorable insights about AI but labeled as "hallucinating" or unreliable when they criticize AI development or usage.
Iterative Verification of Unpopular Results:
The researcher recounts rechecking and validating unexpected results multiple times to ensure their robustness, only to face skepticism.
Analogously, AI skeptics are often compelled to overjustify their critiques, while proponents maintain that AI's flaws will be resolved with future improvements, regardless of evidence.
Broader Analogies Noted:
Selective Belief Systems: Both discussions highlight a tendency to validate ideas that align with existing belief systems (religious or ideological) and dismiss those that challenge them.
Inevitable Progress vs. Flawed Reality: The belief in AI's inevitability parallels how some view systemic issues (like biases) as unavoidable, emphasizing "moving forward" over addressing foundational critiques.
Validation through Volume: Like the eight fresh RAs hired to redo the research, there's a push in AI to provide massive amounts of data or improvements to overwhelm skepticism, even if the core issues persist.
This transcript indeed presents several analogies applicable to the ongoing discourse in AI. The highlighted themes could provide a basis for further discussion on cognitive biases in scientific and technological advancements.
Grok’s answer:
The transcript from the document indeed presents a scenario that can be analogized to the reception and interpretation of AI research and development. Here are some analogies between the situation described in the transcript and common narratives around AI:
Selective Acceptance of Results:
Transcript: The researcher finds that there is no racial bias in police shootings, which contradicts the expected narrative. Despite rigorous methodology, this result is not well-received because it doesn't align with prevailing beliefs or expectations.
AI Context: AI research or outputs are often celebrated when they affirm positive or progressive views about AI's capabilities or future. Negative or critical findings about AI's limitations, biases, or potential risks are frequently dismissed or downplayed as "hallucinations" or "areas for improvement."
Narrative Bias:
Transcript: The researcher's colleagues suggest not publishing the part of his study that doesn't fit the narrative they prefer, indicating a bias towards results that confirm their worldview.
AI Context: There's a tendency in AI discourse to emphasize results that support the inevitability and benevolence of AI, while critical or cautionary insights are often sidelined or criticized. This can be seen in how discussions about AI ethics, bias, or negative impacts are sometimes treated as less significant or "not the full picture."
Reaction to Uncomfortable Truths:
Transcript: People "lose their minds" when the result doesn't align with their beliefs or desires, leading to immediate and strong backlash against the researcher.
AI Context: When AI systems produce outputs or researchers publish findings that challenge the "AI is the future" narrative or suggest caution or skepticism, there can be significant pushback, accusations of misunderstanding AI, or claims that these are mere "growing pains" of a technology on its way to perfection.
Professional and Personal Risk:
Transcript: Colleagues warn the researcher about the career risk of publishing his results due to their unpopularity.
AI Context: AI researchers or developers who highlight risks, ethical issues, or limitations might face professional repercussions, such as funding cuts, ostracism from the community, or damage to their reputation, especially if their findings contradict the optimistic AI narrative.
Confirmation Bias in Acceptance of Research:
Transcript: The suggestion to split the publication of results based on whether they align with or challenge existing views shows a clear bias in what research is deemed worthy of publication.
AI Context: There's a similar bias where research that aligns with the positive, transformative view of AI gets more visibility, funding, and acceptance, whereas research pointing out flaws or negative aspects might struggle for recognition unless it's reframed in a way that still supports AI's overall positive trajectory.
In summary, both scenarios illustrate how deeply held beliefs or expectations can shape the acceptance and interpretation of research findings, where results that align with these beliefs are embraced, and those that contradict them are met with skepticism or outright rejection.
Perplexity’s answer:
Yes, there are indeed analogies between the situation described in the transcript and the current discourse surrounding AI development and commercialization. The statement "People lose their minds when they don't like the result" is highly applicable to both contexts. Here are the key analogies:
Unexpected results causing backlash:
In the transcript: The researcher's findings of no racial bias in police shootings led to strong negative reactions.
In AI: When AI research or models produce results that don't align with expected outcomes or popular beliefs, they often face similar backlash.
Selective acceptance of results:
In the transcript: Colleagues accepted the first part of the study showing bias in low-level use of force but rejected the second part showing no bias in shootings.
In AI: Positive results about AI capabilities are often readily accepted, while negative findings or limitations are more likely to be dismissed or downplayed.
Pressure to suppress unfavorable findings:
In the transcript: Colleagues advised against publishing the controversial results to avoid career damage.
In AI: There may be pressure to focus on positive aspects of AI and downplay potential risks or limitations to maintain funding or public support.
Bias in interpreting results:
In the transcript: People quickly dismissed the study without fully reading or understanding it.
In AI: Quick judgments about AI capabilities or limitations are often made without thorough examination of the underlying research or technology.
Double standards in result acceptance:
In the transcript: Colleagues suggested publishing only the favorable part of the results.
In AI: There's a tendency to highlight AI successes while minimizing or explaining away failures or limitations.
These analogies highlight a common human tendency to favor information that confirms existing beliefs and to react negatively to information that challenges those beliefs, whether in the context of social science research or AI development.
[AI models seem to have great trouble creating a picture of students being lectured by a robot while keeping (both) their index fingers in (both) their ears]

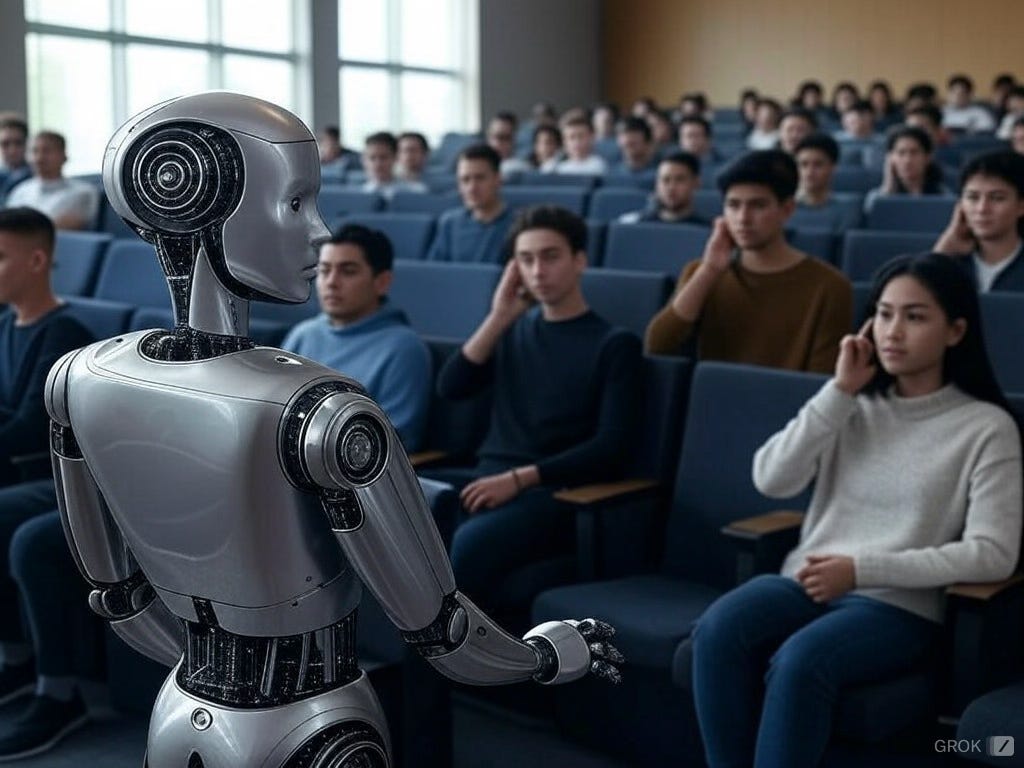
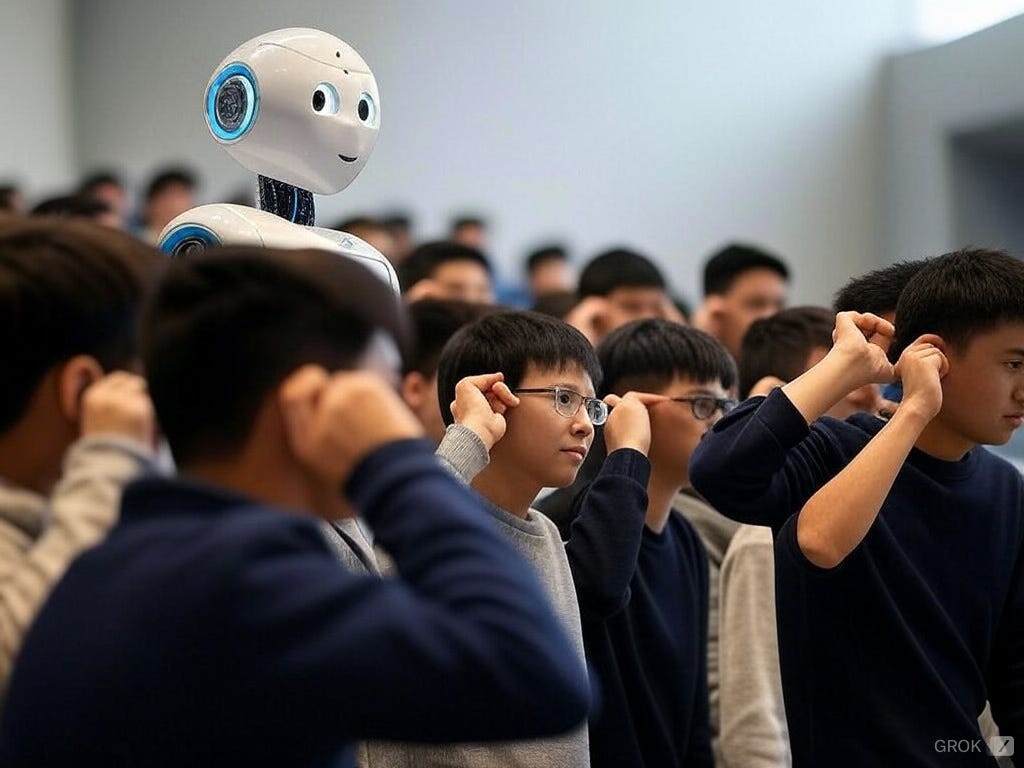
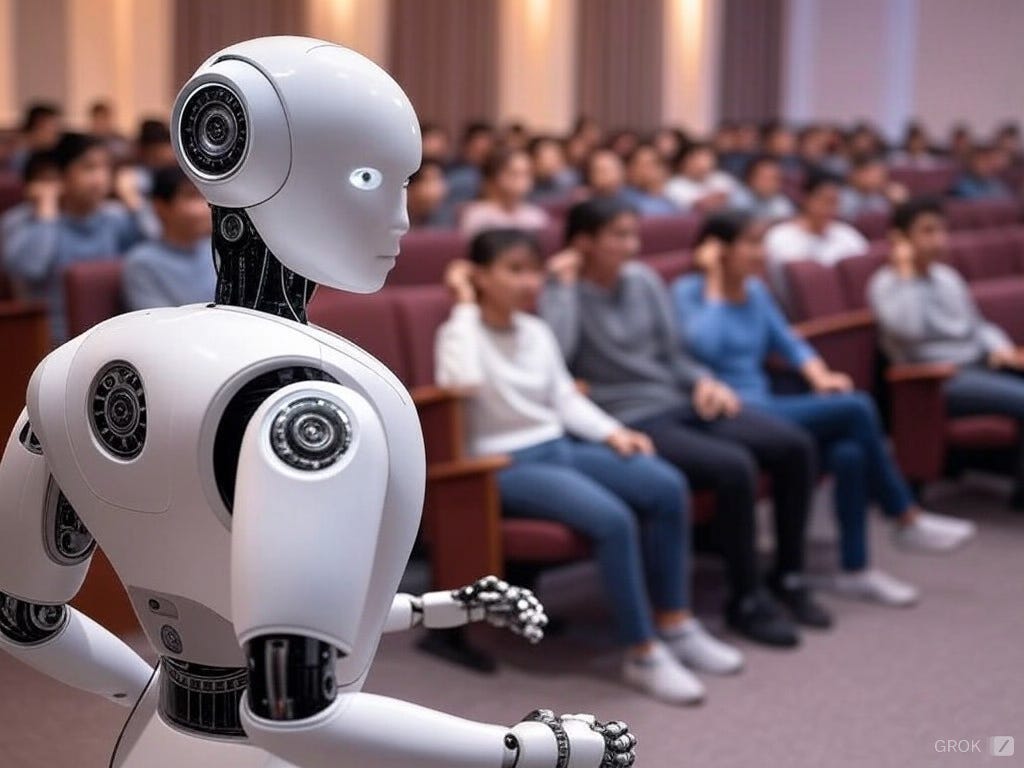
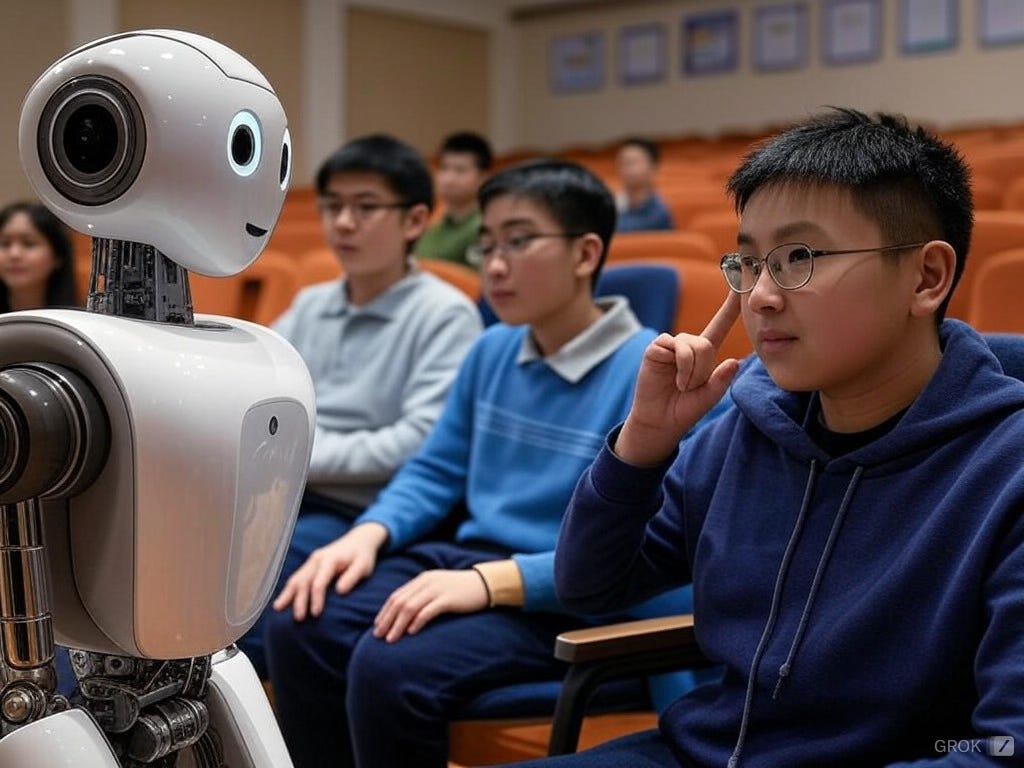
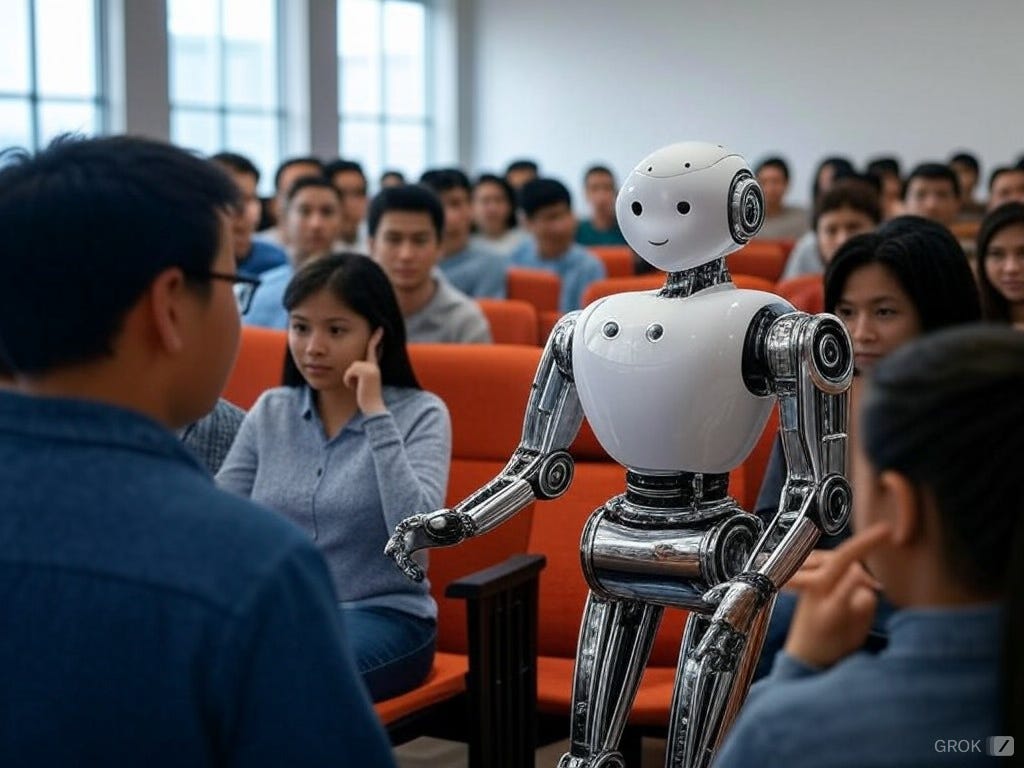
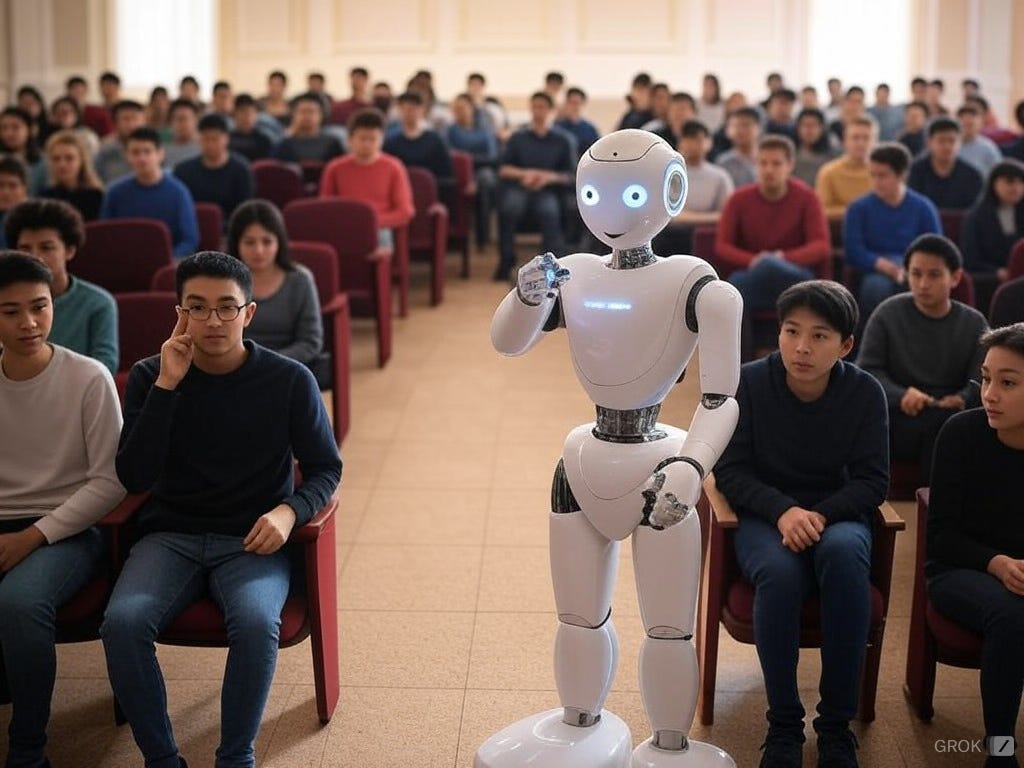
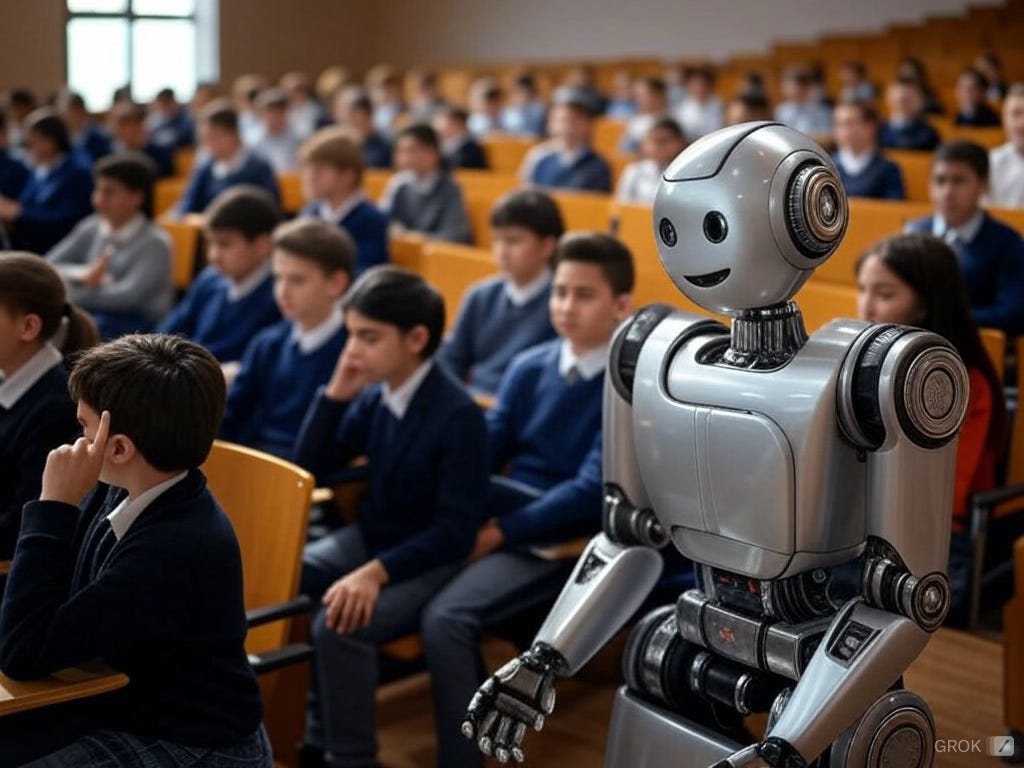
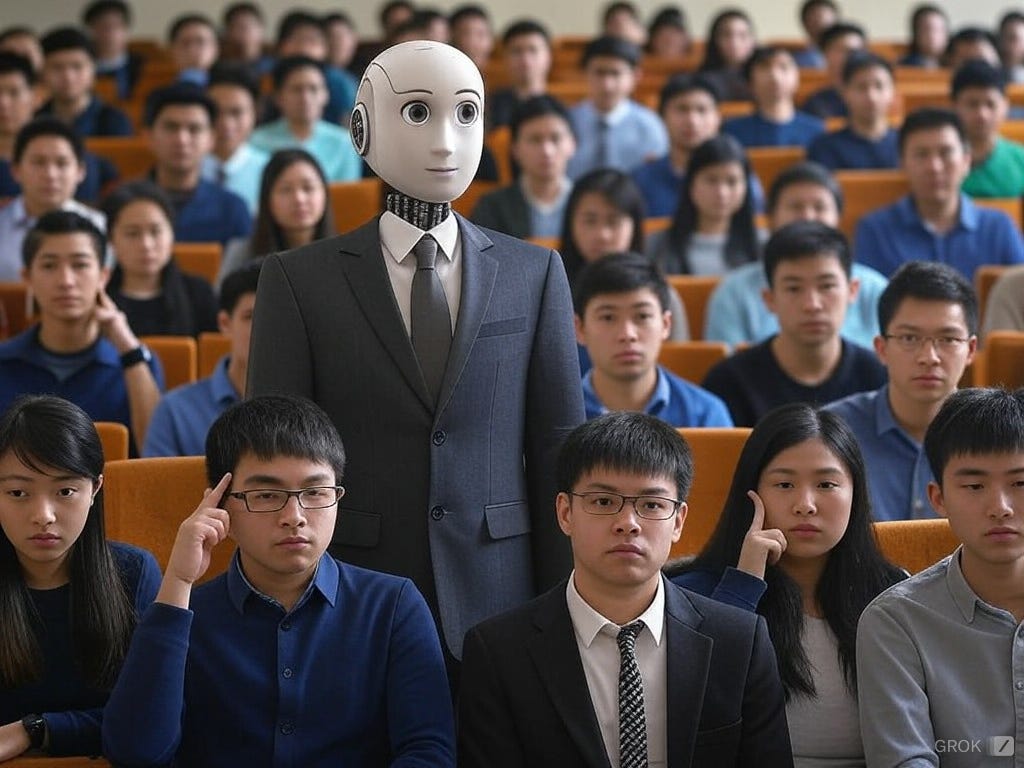
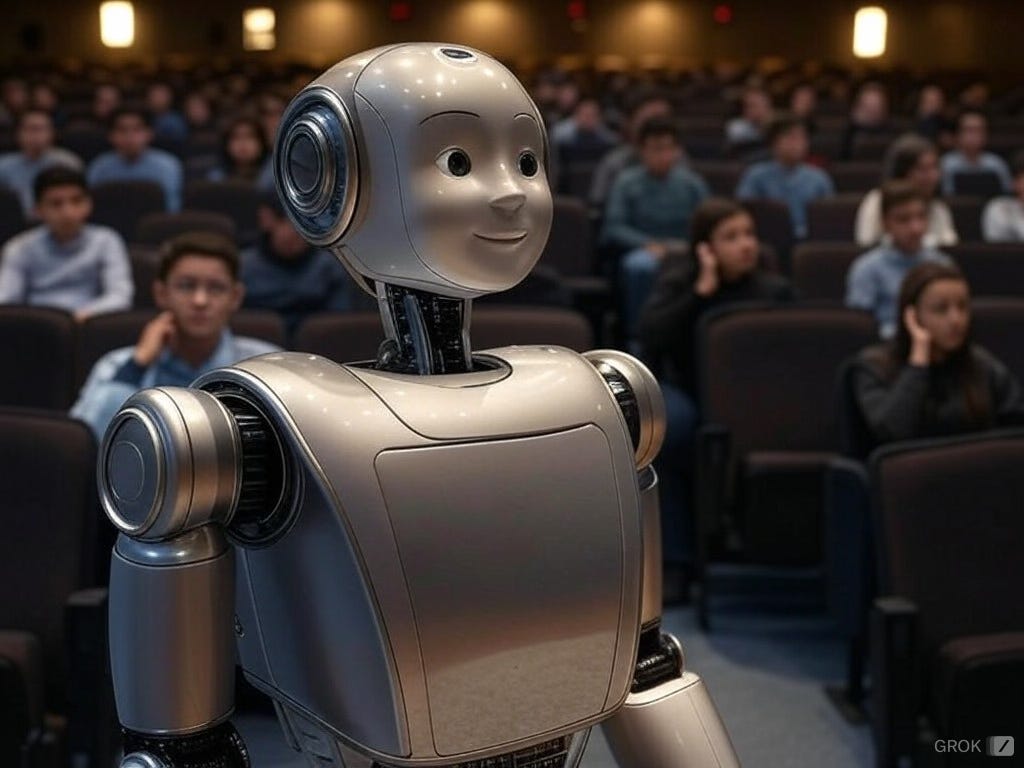
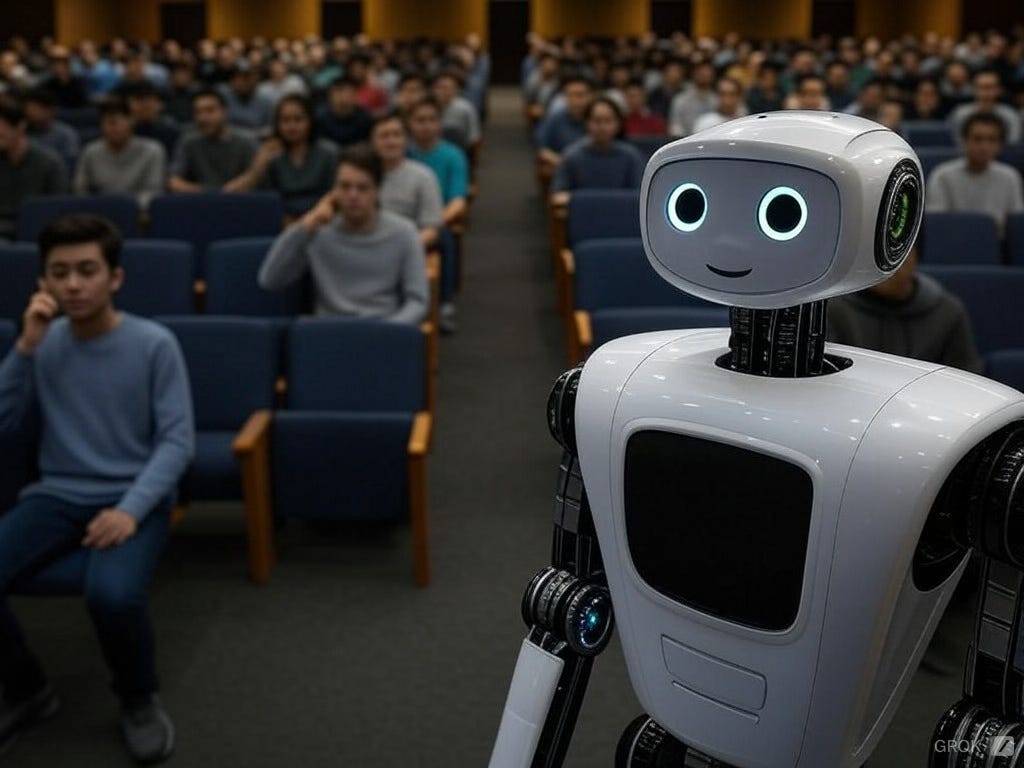