- Pascal's Chatbot Q&As
- Posts
- GPT-4o: While it's true that some AI makers may focus more on improving models than on ethical considerations, there are ways to encourage greater adoption of the recommendations in the paper.
GPT-4o: While it's true that some AI makers may focus more on improving models than on ethical considerations, there are ways to encourage greater adoption of the recommendations in the paper.
Stronger regulations, market pressure, internal culture shifts, financial incentives, transparency mechanisms, and collaborative efforts can make the ethical alignment of AI systems more compelling.
Question 1 of 3 for ChatGPT-4o: Please read the paper "AI Value Alignment: Guiding Artificial Intelligence Towards Shared Human Goals" and tell me what it says in easy to understand language
ChatGPT-4o’s answer:
The paper "AI Value Alignment: Guiding Artificial Intelligence Towards Shared Human Goals" from the World Economic Forum explores how AI systems can be designed to align with human values such as justice, privacy, and fairness. The goal is to ensure that AI behaves ethically and respects societal norms throughout its lifecycle, from design to deployment.
Key Concepts:
AI Value Alignment: This is the idea that AI systems should operate in ways that reflect human values. It's about making sure AI decisions and behaviors are in harmony with human ethics and norms, not just technically sound.
Challenges in Value Alignment: Human values differ across cultures and contexts. What is considered respectful or fair in one society might be different in another. AI systems must account for these variations and be adaptable.
Balancing Ethics and Technology: While technical features like reliability and transparency are important, they must be balanced with ethical principles to ensure AI doesn’t cause harm or violate rights. This includes building in mechanisms for fairness and privacy while maintaining system robustness.
Practical Approaches: The paper discusses frameworks and tools for embedding these values into AI systems. This involves creating clear guidelines, actively involving stakeholders (like users and communities), and conducting continuous audits to ensure the systems remain aligned with ethical standards.
Enablers of Value Alignment:
Frameworks and Guidelines: Structured approaches help ensure AI is developed and managed ethically.
Human Engagement: Ongoing involvement of people is crucial to adapt AI systems as societal values evolve.
Organizational Change: Companies need to create a culture where ethical AI is a priority.
Audits and Assessments: Regular evaluations are needed to check if AI systems stay aligned with values.
Red Lines: These are boundaries AI should not cross, like causing harm or discriminating. Setting red lines ensures that AI systems operate within safe, ethical limits, with human oversight always in place.
In short, the paper emphasizes that AI value alignment is an ongoing process that requires collaboration, transparency, and constant monitoring to ensure AI technologies support and enhance human well-being while adhering to ethical standards.
Question 2 of 3 for ChatGPT-4o: Do you agree with these arguments? Is anything still missing from the paper?
ChatGPT-4o’s answer:
The arguments presented in the paper on AI value alignment are generally well-reasoned and cover critical areas necessary for developing ethical and responsible AI. It is essential to align AI systems with human values, as this can mitigate the risks of harm, discrimination, or unintended consequences. The paper does a good job of emphasizing:
Ethical principles (justice, fairness, privacy) and technical aspects(robustness, transparency)
Cultural differences and how they affect value interpretation
The need for continuous audits and human oversight
The concept of "red lines" to avoid AI crossing ethical boundaries
However, a few areas could be further explored or strengthened:
Accountability in Practice: While the paper emphasizes the need for human oversight, it does not deeply explore who is accountable when things go wrong, particularly in complex, global AI systems with multiple stakeholders. Clearer definitions of responsibility at different stages (from developers to users to policymakers) would be beneficial.
AI Bias Mitigation Beyond Technical Fixes: Although the paper touches on fairness and bias detection, it could delve deeper into how societal biases can be perpetuated or even exacerbated by AI systems. A broader discussion on how to involve marginalized groups and address systemic inequalities, beyond technical fixes like fairness audits, might enhance the ethical robustness of the solutions.
Ethics vs. Profit Incentives: The tension between ethical AI and profit-driven goals is mentioned but not thoroughly examined. Many AI systems are developed in corporate environments where the pressure for innovation and profits can conflict with ethical considerations. More discussion on this trade-off and how to resolve it (e.g., through stronger regulation or market incentives for ethical AI) would add practical depth.
Global Governance Mechanisms: While the paper acknowledges cultural differences, it lacks a detailed roadmap for global governance of AI. With AI systems deployed across borders, the paper could address how international bodies and standards might evolve to ensure cohesive global regulation that respects cultural nuances but upholds universal ethical principles.
AI in Decision-Making Roles: There’s limited discussion on the increasing role of AI in decision-making that has high stakes (e.g., legal judgments, medical decisions). The ethical implications of AI taking on such roles and the safeguards needed in these scenarios could be explored further.
In summary, while the paper provides a strong foundation, it could be enhanced by addressing accountability, bias beyond technical aspects, the profit-ethics tension, global governance strategies, and the specific challenges of AI in high-stakes decision-making. These aspects would round out the discussion and make the framework more comprehensive and applicable in real-world scenarios.
Question 3 of 3 for ChatGPT-4o: Some people think it is unlikely the recommendations in the paper will be taken seriously by AI makers because they are too focused on features and improving the models. Would you agree and what could or should convince AI Makers to take the suggestions in the paper seriously?
ChatGPT-4o’s answer:
The concern that AI makers might not take the recommendations in the paper seriously is valid. Many AI companies are heavily focused on improving their models' capabilities, efficiency, and performance, often at the expense of ethical considerations. This tension between technological innovation and ethical safeguards is common in fast-moving fields like AI. Here are some reasons why AI makers might not prioritize the recommendations and what could be done to encourage them to take them seriously:
Why AI Makers Might Overlook the Recommendations:
Focus on Performance and Profit: AI developers, especially in competitive industries, are under pressure to produce high-performing models quickly, which may lead them to prioritize features and technical advancements over ethical concerns. Companies often see ethical guidelines as slowing down innovation or adding costs without immediate returns.
Lack of Short-Term Incentives: Ethical alignment and value-based design are long-term goals, whereas the AI industry is often driven by short-term results like profits, market share, and faster product cycles. Many developers may not see immediate financial or operational benefits from implementing these recommendations.
Complexity and Unclear Accountability: Implementing value alignment and ethical standards across an organization is complex and can be hard to quantify. For many companies, the responsibility for AI ethics may not be clearly defined, leaving ethical alignment as a "soft" area that's easy to overlook.
Perception of Regulatory Gap: In regions where AI ethics regulations are weak or still developing, companies may feel less pressure to adopt robust ethical standards until governments mandate them. The absence of strong regulation allows companies to prioritize features over value alignment.
What Could Convince AI Makers to Take These Recommendations Seriously:
Stronger Regulatory Frameworks: Governments and international bodies could create mandatory ethical standards for AI, ensuring that developers align their models with human values. If regulations impose penalties for non-compliance or offer incentives for ethical AI, companies will have clear motivation to integrate these guidelines.
Consumer Demand and Market Pressure: As consumers become more aware of the potential harm caused by unethical AI (e.g., biased systems, privacy violations), companies may face market pressure to ensure their products align with ethical standards. Public scandals or backlash (like in cases of biased facial recognition systems) could push AI makers to adopt ethical practices to maintain their reputation.
Financial Incentives and Partnerships: Ethical AI could be framed as a competitive advantage, especially in industries like healthcare, finance, or education, where trust and transparency are critical. Partnerships with ethical organizations, government contracts, and financial incentives like grants for ethical AI development could make ethical alignment more attractive to AI makers.
Internal Organizational Change and Ethics Officers: Companies could appoint dedicated ethics officers or create internal AI ethics teams to oversee the development process and ensure alignment with human values. Making ethical development part of the company’s culture and leadership vision would help shift priorities.
Transparency and Accountability: AI companies might be incentivized by frameworks that promote transparency, like requiring AI audits or certifications (similar to environmental standards) to demonstrate that their systems are ethical and value-aligned. This would add a layer of trust for users, investors, and regulators, leading to more business opportunities.
Collaboration with International Organizations: AI makers could collaborate with bodies like the World Economic Forum, United Nations, or OECD to develop global standards for ethical AI. Participating in such initiatives might give companies a leading role in shaping the future of AI ethics, which could provide them with a competitive edge and increased credibility.
Risk of Legal and Ethical Failures: Demonstrating the long-term risks of unethical AI (like legal liability, public relations crises, or costly system failures) might motivate companies to prioritize these recommendations. Ethical lapses, such as data breaches or discriminatory AI behavior, can have devastating financial and reputational consequences.
Conclusion:
While it's true that some AI makers may focus more on improving models than on ethical considerations, there are ways to encourage greater adoption of the recommendations in the paper. Stronger regulations, market pressure, internal culture shifts, financial incentives, transparency mechanisms, and collaborative efforts can make the ethical alignment of AI systems more compelling for companies. To succeed, ethical AI must be positioned not just as a moral imperative but as a strategic advantage.
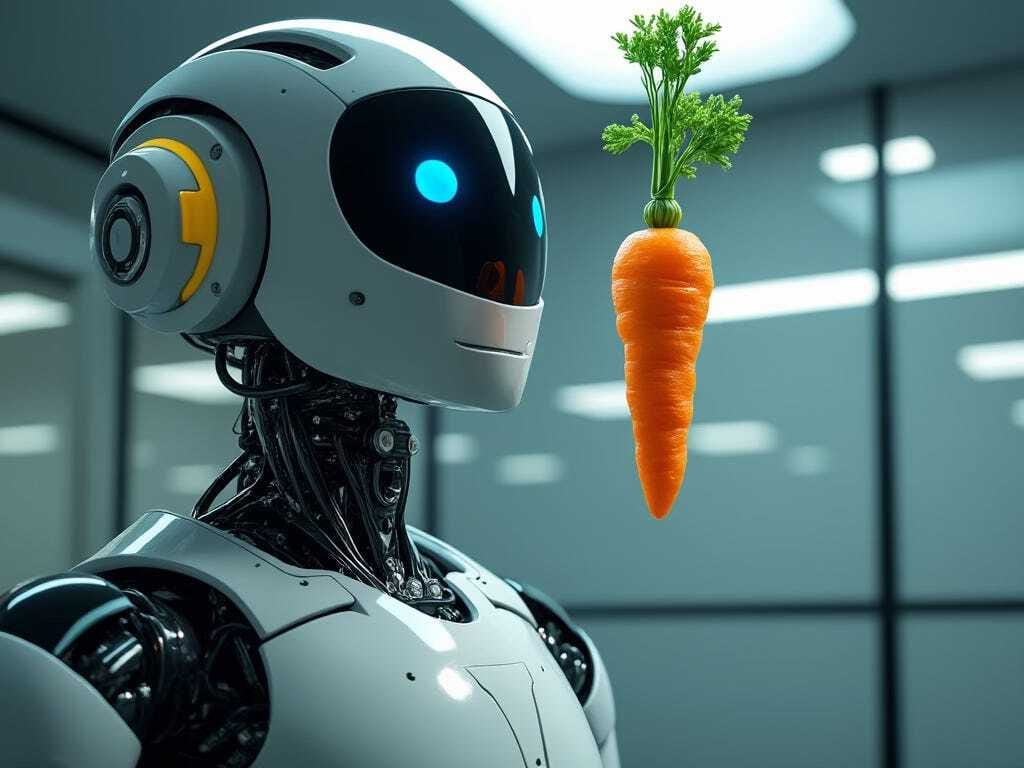