- Pascal's Chatbot Q&As
- Posts
- GPT-4o: While AI, DS, and ML technologies are viewed as critically important, their actual deployment across organizations remains lower than expected...
GPT-4o: While AI, DS, and ML technologies are viewed as critically important, their actual deployment across organizations remains lower than expected...
...especially in sectors outside healthcare, consumer services, and large organizations. This creates a disconnect between the hype and actual use, raising concerns about the real-world challenges.
Question 1 of 3 for ChatGPT-4o: Please read the “AI, Data Science, and Machine Learning Market Study” and tell me what it says, in easy to understand language.
ChatGPT-4o’s answer:
The "AI, Data Science, and Machine Learning Market Study" by Dresner Advisory Services focuses on the current trends, use cases, and future adoption of AI, data science, and machine learning (AI, DS, ML) technologies across industries. Here's a simplified breakdown of the key findings:
Growing Importance: AI, DS, and ML are becoming increasingly important, with organizations using them to improve operations, forecasting, and innovation. The study highlights that while adoption has plateaued recently, there is strong future potential, particularly in healthcare, consumer services, and research and development.
Generative AI on the Rise: One notable trend is the rapid expansion of generative AI, which is being integrated into many companies' operations, with significant growth expected in the next 12 months.
Common Use Cases: The most important use cases for AI, DS, and ML include customer segmentation, demand forecasting, and determining customer lifetime value. However, current deployment remains low but is expected to increase in the near future.
Barriers to Adoption: Although AI, DS, and ML technologies are gaining traction, there have been some hesitations and limited deployments due to uncertainty, limited use cases, and complexity. This is especially true in smaller organizations, which tend to lag behind larger companies in adoption.
Industry Differences: Different industries are at varying stages of adoption. For instance, healthcare and consumer services are leading in full production use, while industries like manufacturing and government are slower to implement AI and DS technologies.
Data Literacy and Success Correlation: Organizations with higher data literacy levels are more likely to use AI, DS, and ML successfully. Companies with more successful business intelligence (BI) programs also show stronger adoption of these technologies.
In short, AI, data science, and machine learning are seen as critical tools for innovation, but their adoption is still in its early stages, with larger companies and certain industries leading the way. The future looks promising, particularly for generative AI and key business applications like customer segmentation and forecasting.
Question 2 of 3 for ChatGPT-4o: What are the most surprising, controversial and valuable statements and findings in the report?
ChatGPT-4o’s answer:
Here are some of the most surprising, controversial, and valuable findings in the AI, Data Science, and Machine Learning Market Study:
Surprising Findings:
Generative AI is expanding rapidly: The study highlights a surprising increase in the use of generative AI, with organizations rapidly integrating it into production and experimental use cases. The widespread adoption expected over the next year shows how quickly this technology is moving from experimental to essential in business operations .
Larger companies dominate AI and ML use cases: Despite the increasing prominence of AI and ML, uptake in smaller organizations is surprisingly low. Very large organizations (more than 10,000 employees) lead significantly in nearly all use cases, including demand forecasting and fraud detection. This disparity is notable, with smaller companies often lagging far behind in AI deployment .
Controversial Findings:
Limited deployment despite high potential: While AI, DS, and ML technologies are viewed as critically important, their actual deployment across organizations remains lower than expected, especially in sectors outside healthcare, consumer services, and large organizations. This creates a disconnect between the hype and actual use, raising concerns about the real-world challenges of deploying these technologies .
Low urgency in small and young companies: There is a noticeable lack of urgency in adopting AI technologies among both the youngest (less than 5 years) and oldest (16+ years) organizations, with the majority of interest coming from companies that are 11-16 years old. This demographic gap raises questions about the real drivers of AI adoption and where the barriers lie for smaller or older companies .
Valuable Insights:
Critical features for AI and DS: The most important features identified for AI and data science include traditional statistical methods like regression models, outlier detection, and model explainability. These features are critical for improving transparency and governance in AI models, highlighting where companies should focus when developing or purchasing AI tools .
Generative AI’s expected growth: Generative AI is poised for major growth, with a significant number of organizations planning to incorporate it into their operations in the next 12 months. This points to generative AI becoming a central tool for innovation and automation in the near future .
These findings reflect the growing but uneven landscape of AI adoption and reveal areas where both opportunities and challenges exist for organizations across industries.
Question 3 of 3 for ChatGPT-4o: When reading the report, which 'ingredients' and factors would you say are critical for success and which factors are likely to determine a less successful outcome for AI adoption or implementation?
ChatGPT-4o’s answer:
The critical factors for success and those that could lead to less successful outcomes for AI adoption and implementation, according to the AI, Data Science, and Machine Learning Market Study, can be categorized as follows:
Critical Factors for Success:
High Data Literacy: Organizations with high levels of data literacy are significantly more likely to have successful AI and data science implementations. Companies with higher data literacy rates (37% adoption rate) tend to deploy AI in production more effectively than those with moderate or low literacy .
Success with Business Intelligence (BI): There is a strong correlation between organizations that consider themselves successful with BI and their success in deploying AI, DS, and ML technologies. Completely successful BI organizations are 31% more likely to report in-production use of AI than somewhat successful or unsuccessful organizations .
Generative AI Adoption: Organizations that actively experiment with and adopt generative AI are more likely to find success, especially in industries like customer segmentation, demand forecasting, and risk management. The study predicts significant growth in generative AI use within the next 12 months .
Organizational Size and Maturity: Very large organizations (>10,000 employees) show much higher success rates in AI deployments (58%) compared to small organizations (1-100 employees, 17%). Larger companies often have the resources and capacity to implement and scale AI projects more efficiently .
Factors Likely to Lead to Less Successful Outcomes:
Lack of Data Literacy: Organizations with lower data literacy face significant challenges in adopting AI technologies. Only 19% of organizations with low data literacy have AI in production, which indicates that a lack of understanding and comfort with data can impede successful deployment .
Limited BI Success: Organizations that struggle with their BI initiatives are less likely to successfully adopt AI technologies. This suggests that companies without a strong foundation in BI may face difficulties in expanding into more complex data science and AI operations .
Uncertainty and Limited Use Cases: Many organizations are still evaluating AI and data science software or using it in limited ways, often as proof of concept. This hesitancy and early-stage experimentation, especially in industries like government and manufacturing, may lead to slower progress and less successful outcomes .
Smaller Organizations Lagging: Smaller companies, especially those with fewer than 100 employees, report significantly lower AI adoption rates. Their size often limits their ability to invest in the necessary infrastructure, talent, and resources to effectively implement AI .
In summary, success in AI adoption is heavily influenced by organizational readiness in terms of data literacy, BI success, and the ability to experiment with and scale technologies like generative AI. On the flip side, lack of readiness, data understanding, and resources can hinder AI deployment and lead to less successful outcomes.
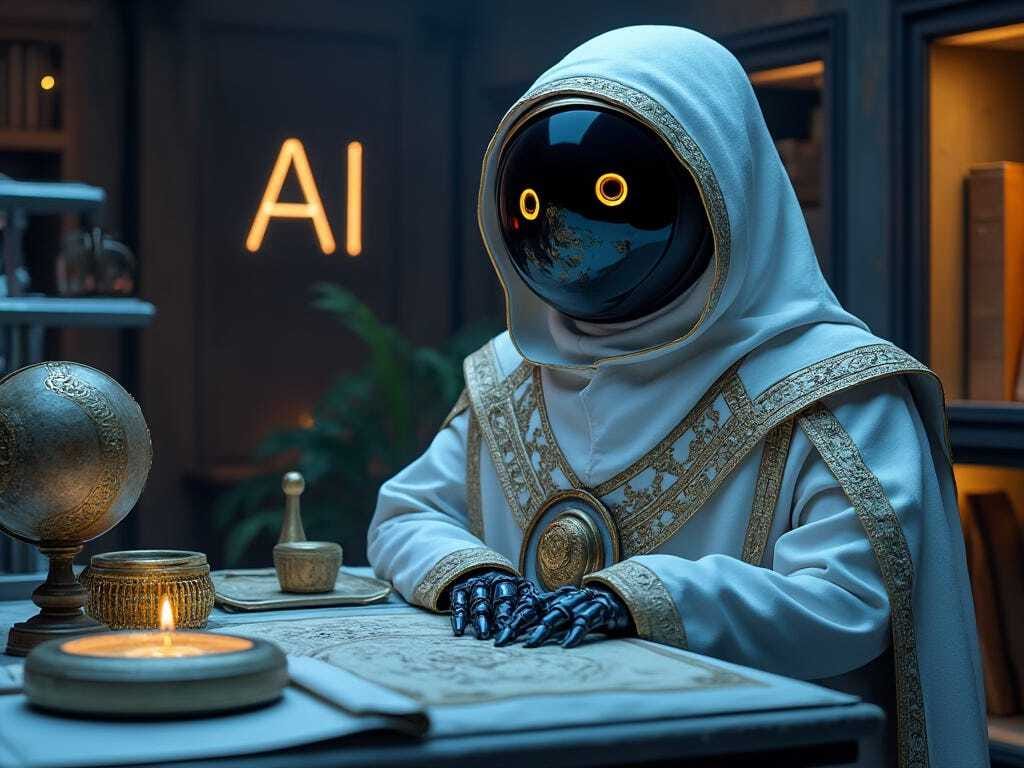