- Pascal's Chatbot Q&As
- Posts
- GPT-4o: These concerns reflect broader issues regarding Meta’s handling of copyrighted content, the role of legal counsel in guiding potentially unlawful practices...
GPT-4o: These concerns reflect broader issues regarding Meta’s handling of copyrighted content, the role of legal counsel in guiding potentially unlawful practices...
...and the company’s compliance with discovery obligations. Meta's strategy should include measures to ensure compliance with copyright laws, foster transparency, and uphold ethical practices.
Question 1 of 4 for ChatGPT-4o: Please read the article "Bombshell allegations: Kadrey plaintiffs assert “Meta’s in-house counsel engaged in and advised Meta on Its Illegal Activity” and invoke crime-fraud exception for discovery. Also claim Mark Zuckerberg should be deposed again." and tell me what the key concerns are regarding Meta's (in)actions
ChatGPT-4o’s answer:
The key concerns raised by the plaintiffs regarding Meta’s actions and inactions include:
Involvement of In-House Counsel in Alleged Illegal Activities:
Plaintiffs allege that Meta’s in-house legal counsel played an active role in approving, concealing, and justifying the use of copyrighted material from pirated datasets for training AI models. This includes actions such as downloading and distributing copyrighted works (alleged violations of the California Comprehensive Data and Access Fraud Act - CDAFA), removing copyright management information (violations of the Digital Millennium Copyright Act - DMCA), and using these works to train their Llama model (violations of the Copyright Act).
Crime-Fraud Exception Invocation:
Plaintiffs claim that Meta’s communications involving in-house counsel should not be protected by attorney-client privilege because they allegedly furthered illegal activities. They argue for the application of the crime-fraud exception, suggesting that these privileged communications were used to plan or conceal illegal activities.
Eleventh-Hour Document Production:
Plaintiffs assert that Meta delayed the production of key documents until the end of the discovery period, despite having collected some of these documents months earlier. This “eleventh-hour dump” of documents allegedly contradicted prior deposition testimony and undermined transparency in discovery.
Contradictions in Testimony:
Plaintiffs argue that newly produced documents contradict previous deposition testimony by key Meta employees, including CEO Mark Zuckerberg. This has led to requests for additional depositions to clarify these contradictions.
Fair Use Defense Critique:
While Meta has asserted a fair use defense regarding its use of copyrighted works, plaintiffs argue that this defense is irrelevant to the invocation of the crime-fraud exception. They suggest that such a defense cannot retroactively legitimize actions that constitute a prima facie showing of crime or fraud.
Scope and Transparency of Dataset Use:
There are allegations that Meta did not fully disclose the datasets used to train its AI models, particularly datasets such as Books3 and others identified as “shadow datasets.” Plaintiffs demand greater transparency in the use and sourcing of these datasets.
These concerns reflect broader issues regarding Meta’s handling of copyrighted content, the role of legal counsel in guiding potentially unlawful practices, and the company’s compliance with discovery obligations. The plaintiffs are seeking further discovery, including additional depositions and access to allegedly privileged documents, to address these issues.
Question 2 of 4 for ChatGPT-4o: Do you deem the evidence convincing?
ChatGPT-4o’s answer:
The evidence presented by the plaintiffs raises serious questions but has some areas that would require further scrutiny to assess its overall strength. Here's a breakdown of the considerations:
Strengths of the Evidence:
Involvement of In-House Counsel:
The plaintiffs cite specific examples where Meta’s in-house counsel allegedly approved or was involved in activities like using pirated datasets, removing copyright management information, and developing legal strategies to justify this conduct. This includes references to internal documents and a New York Timesarticle reporting on internal Meta meetings discussing the use of copyrighted works.
Crime-Fraud Exception Argument:
If proven, the plaintiffs’ claims about the role of counsel in furthering unlawful activities could invalidate Meta’s attorney-client privilege under the crime-fraud exception. This is a serious allegation with significant implications for Meta’s defense.
Eleventh-Hour Document Dump:
The timing of Meta’s document production—just before the close of discovery—raises questions about whether this was an intentional strategy to limit the plaintiffs’ ability to use these documents effectively. Some documents allegedly contradict previous deposition testimony, which could undermine Meta's credibility.
Dataset Transparency:
Plaintiffs highlight that Meta has not fully disclosed all datasets used for AI training, which could suggest an effort to obscure the full scope of potentially infringing activity. The lack of clarity about Meta's use of "shadow datasets" adds to this concern.
Weaknesses and Counterarguments:
Meta’s Fair Use Defense:
Meta argues that its actions fall under the scope of fair use, which is a novel and unresolved legal issue in the context of AI training. Courts have not yet ruled definitively on whether using copyrighted works in this way is permissible. Meta’s position that it acted in good faith and sought legal counsel on these matters could mitigate the crime-fraud allegations.
Lack of Criminal Adjudication:
The plaintiffs’ allegations hinge on the claim that Meta’s actions constitute crimes (e.g., copyright infringement, DMCA violations). However, no court has yet ruled that these actions meet the threshold of criminal behavior. Without such a determination, the claim that the crime-fraud exception applies could be seen as premature.
Speculative Elements:
Some of the plaintiffs’ claims, such as inferences about the intent behind certain redactions or the alleged role of counsel, may rely on circumstantial evidence rather than direct proof. For example, the NYT article provides a narrative but may not constitute direct evidence of illegal intent.
Meta’s Discovery Compliance Argument:
Meta defends the timing of its document production, attributing delays to the scope of the plaintiffs’ requests and the court’s own deadlines. If Meta can demonstrate good faith compliance with discovery obligations, this could weaken the plaintiffs’ claims of “sandbagging”.
Conclusion:
The evidence is concerning and raises significant legal and ethical questions, particularly about the role of Meta’s in-house counsel and the use of copyrighted material. However, whether it is "convincing" depends on the standard of proof and the context:
For Discovery Disputes: The evidence may be sufficient to warrant further depositions and in-camera review of documents under the crime-fraud exception.
For the Underlying Case: The plaintiffs face challenges in proving that Meta’s actions constitute criminal behavior or fraud, particularly given the unresolved legal questions around fair use and AI training.
Ultimately, the strength of the evidence will depend on how well the plaintiffs can substantiate their claims with specific, direct evidence rather than inferences or external narratives.
Question 3 of 4 for ChatGPT-4o: What would be a legally sound outcome in this case that reflects the spirit of the law and would be a robust example of 'justice being done'?
ChatGPT-4o’s answer:
A legally sound and just outcome in this case would strike a balance between accountability for potential wrongdoing and respect for emerging legal ambiguities in AI and copyright law. It should aim to reflect the principles of fairness, transparency, and deterrence, while fostering innovation within lawful boundaries. Here's what such an outcome might look like:
1. Full Transparency and Discovery
Immediate Remedy: Meta should be compelled to produce all relevant documents and data without inappropriate redactions or delays. This includes datasets used to train AI models, internal communications, and privilege log entries where attorney-client privilege is improperly invoked.
Crime-Fraud Review: An in-camera review of disputed documents should be conducted by the court to determine whether the crime-fraud exception applies, ensuring that plaintiffs have access to any documents where privilege was used to shield unlawful activities.
2. Accountability for Copyright Infringement
Fair Use Determination: The court should thoroughly evaluate whether Meta’s use of copyrighted works for AI training qualifies as fair use under the four-factor test (purpose, nature of the work, amount used, and market effect). If the use fails the test, a ruling should clarify the boundaries of permissible use of copyrighted material for AI training.
Damages: If infringement is found, Meta should pay damages commensurate with the harm done, including statutory damages for DMCA and copyright violations, and potentially punitive damages if willful infringement is established.
3. Deterrence Against Procedural Abuse
Sanctions for Discovery Misconduct: If the court finds that Meta deliberately delayed document production to frustrate the plaintiffs’ case, it should impose sanctions. This could include monetary penalties, adverse inference rulings, or reopening depositions at Meta’s expense.
Judicial Commentary: A strong judicial statement condemning any efforts to evade transparency, especially in cases involving powerful corporate actors, would set a robust precedent.
4. Affirmation of Legal Counsel’s Ethical Boundaries
Role of In-House Counsel: If the court finds that Meta’s in-house counsel knowingly facilitated illegal activity, those individuals could face sanctions, including reporting to the state bar for potential disciplinary action.
Attorney-Client Privilege Limits: A clear ruling on when attorney-client privilege is forfeited under the crime-fraud exception would guide future corporate conduct.
5. Protection of Technological Innovation
Clarity on AI Training and Copyright: The court should articulate the legal standards for using copyrighted works in AI training, providing guidance to the broader tech and publishing industries. This might include:
Conditions under which fair use might apply.
The need for transparency in dataset sourcing.
Limits on using pirated or misattributed materials.
Collaborative Framework: The court could encourage Meta and the publishing industry to establish a licensing framework or collaborative initiative that respects creators' rights while enabling AI innovation.
6. Broader Implications for Justice
No Overreach: The court must ensure that any findings or sanctions are proportionate and based on clear legal violations, avoiding overreach that could stifle legitimate legal consultation or innovation.
Judicial Precedent: The case should serve as a model for balancing rights enforcement with the need to address novel legal issues arising from AI and digital technologies.
Outcome Example:
Meta is found liable for copyright infringement due to its use of copyrighted works in pirated datasets but receives clear guidelines for future conduct.
Plaintiffs are granted damages and transparency in discovery, but Meta’s fair use defense is acknowledged as raising unresolved questions, fostering legal clarity.
A legal framework for AI dataset use is proposed, balancing rights and innovation.
Judicial scrutiny ensures that Meta’s in-house counsel adheres to ethical obligations, sending a strong message to corporate actors about the limits of privilege.
Why This Reflects Justice:
Fairness to Plaintiffs: Protects the rights of creators and ensures redress for harms caused by unauthorized use of their works.
Balance for Meta: Acknowledges the legal gray areas of AI training while imposing accountability for clearly unlawful acts.
Broader Legal Clarity: Offers guidance to industries navigating AI and copyright, reducing future litigation and promoting lawful innovation.
Deterrence: Prevents abuse of discovery processes and underscores the ethical obligations of legal counsel in corporate settings.
This outcome ensures that the spirit of the law—fairness, innovation, and accountability—is upheld while addressing the unique challenges posed by this novel legal context.
Question 4 of 4 for ChatGPT-4o: What should Meta be doing - or not be doing - in the future to prevent these issues from occurring?
ChatGPT-4o’s answer:
Meta should adopt a comprehensive strategy to prevent similar legal and ethical issues in the future. The strategy should include measures to ensure compliance with copyright laws, foster transparency, and uphold ethical practices in data collection and AI training. Here's what Meta should focus on:
1. Ethical and Legal Data Acquisition
Strict Vetting of Datasets: Meta must ensure that all datasets used for AI training are legally obtained and meet copyright compliance standards. This includes avoiding datasets sourced from pirated libraries like Books3 or LibGen.
Licensing Agreements: Develop robust licensing frameworks with content creators, publishers, and rights holders to use copyrighted materials for AI training, with clear terms on compensation and permissible use.
Metadata Preservation: Ensure copyright management information (CMI) is not stripped during data preprocessing, to avoid potential DMCA violations.
2. Transparency in AI Development
Public Dataset Disclosures: Meta should maintain and publish a comprehensive list of datasets used for AI training, including details of how the data was sourced, its licensing status, and its purpose.
Independent Audits: Conduct regular audits of datasets by independent third parties to verify compliance with copyright and data privacy laws.
Clear Fair Use Policies: Where fair use is invoked, Meta should articulate its reasoning transparently and consistently, using case-specific analyses and legal precedents.
3. Strengthened Legal and Compliance Oversight
Dedicated Compliance Teams: Establish specialized teams to oversee data acquisition and use, ensuring adherence to copyright laws and ethical standards.
Ethical Guidelines for In-House Counsel: Ensure legal counsel provides guidance grounded in compliance and ethics, avoiding advice that could be perceived as facilitating unlawful activities.
Whistleblower Protections: Implement systems to allow employees to report potential legal or ethical violations confidentially, fostering a culture of accountability.
4. Improved Discovery Practices
Proactive Document Management: Meta should ensure that all relevant documents are identified, reviewed, and disclosed in a timely manner during litigation to avoid accusations of “sandbagging.”
Transparency in Privilege Claims: Clearly delineate the boundaries of attorney-client privilege and avoid overusing it to shield routine business decisions.
5. Responsible AI Practices
Ethical AI Charter: Adopt an ethical AI charter that governs the development, deployment, and training of AI systems, focusing on fairness, compliance, and transparency.
Minimize Data Usage: Explore and develop techniques for minimizing reliance on copyrighted material, such as synthetic data generation, public domain data, or licensing-based collaborations.
6. Collaboration with Stakeholders
Industry Partnerships: Work collaboratively with publishers, authors, and copyright organizations to establish standards for AI training datasets.
Policy Advocacy: Advocate for clearer legal frameworks around AI and copyright, including licensing regimes or statutory exemptions for training datasets, to reduce legal ambiguity.
7. Enhanced Corporate Governance
Executive Accountability: Ensure senior leaders, including the CEO, are well-informed and accountable for decisions related to AI and data use.
Board Oversight: Establish board-level oversight of AI ethics and compliance to align corporate governance with ethical AI development.
8. User-Focused AI Innovation
Bias and Fairness Assessments: Regularly evaluate AI outputs for bias and ensure fairness in model predictions.
Educational Content: Include public-facing materials that educate users about the ethical and legal measures Meta employs in its AI systems.
9. Responsive Legal and Ethical Training
Employee Training: Provide comprehensive training to employees on copyright law, ethical AI practices, and legal obligations to foster a culture of compliance.
Scenario Planning: Develop internal protocols for addressing new legal challenges, ensuring Meta can adapt to regulatory changes swiftly.
10. Avoiding Future Pitfalls
Do Not Rely on Questionable Datasets: Avoid datasets with unclear licensing or sourcing histories, even if their use could arguably fall under fair use.
Do Not Delay Discovery: Ensure timely compliance with court-ordered disclosures to avoid allegations of obstructive behavior.
Do Not Conceal Decisions: Avoid the appearance of impropriety by maintaining open channels of communication with regulators, stakeholders, and the public.
By proactively adopting these measures, Meta can build trust with creators, regulators, and the public while minimizing legal and ethical risks. This approach not only helps avoid future disputes but also establishes Meta as a leader in ethical and responsible AI development.

·
7 DEC
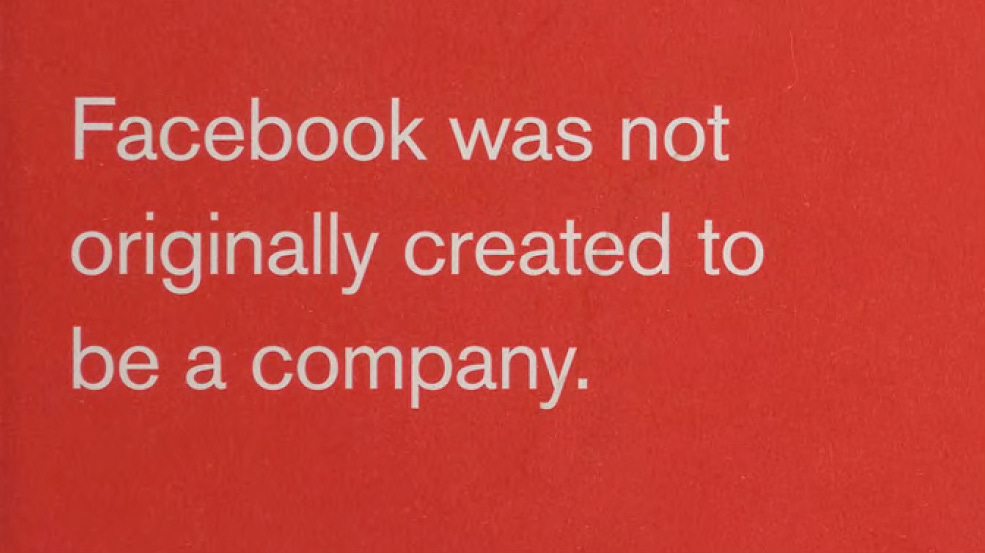