- Pascal's Chatbot Q&As
- Posts
- GPT-4o: The report draws detailed parallels between today’s AI developers and past tobacco companies—accusing the latter of funding deceptive research while suppressing internal data on harms.
GPT-4o: The report draws detailed parallels between today’s AI developers and past tobacco companies—accusing the latter of funding deceptive research while suppressing internal data on harms.
AI companies allegedly threaten bans or legal action against safety researchers who expose vulnerabilities, using restrictive terms of service.
Asking ChatGPT-4o: Please analyze the “Draft Report of the Joint California Policy Working Group on AI Frontier Models” and list the most surprising, controversial and valuable statements made. Conclude by listing all recommendations for AI developers and regulators that you can deduce from the report. Feel free to suggest additional solutions and strategies not mentioned in the report.
ChatGPT-4o’s answer:
Here’s an analysis of the “Draft Report of the Joint California Policy Working Group on AI Frontier Models”, highlighting the most surprising, controversial, and valuablestatements, followed by a synthesized list of recommendations for AI developers and regulators, and some additional suggestions.
🔎 Most Surprising Statements
AI risk comparisons to the tobacco industry: The report draws detailed parallels between today’s AI developers and past tobacco companies—accusing the latter of funding deceptive research while suppressing internal data on harms. It warns AI firms might similarly “distort public understanding” unless legally required to disclose internal risk assessments.
Low transparency levels: Despite AI’s societal importance, developers score poorly on transparency:
Data sourcing: 32%
Risk mitigation: 31%
Safety risks: 47%
Adverse event reporting as a “critical first step”: The report envisions post-deployment incident reporting (like with medical or aviation systems) as essential infrastructure, not just a supplementary governance tool.
⚖️ Most Controversial Statements
Opaque suppression of researchers: AI companies allegedly threaten bans or legal action against safety researchers who expose vulnerabilities, using restrictive terms of service.
Skepticism about developer-level thresholds: The report rejects traditional thresholding methods (e.g., revenue, headcount) because small teams (like DeepSeek) can build high-impact models, whereas large companies dabbling in AI might get over-regulated.
Call for whistleblower protections in AI: The report supports establishing a “right to warn” for insiders in AI companies who want to disclose hidden safety risks—framing it as a public good.
💡 Most Valuable Statements
Safe harbor for independent researchers: Legal protections for third-party evaluators (modeled after cybersecurity) are presented as essential to robust safety culture and public interest risk research.
Hybrid reporting systems: A balanced model combining mandatory developerreporting with voluntary userreporting is advocated to build a comprehensive, real-world incident database.
"Race to the top" through transparency: Publicly disclosed, third-party verified safety practices are said to foster healthy competition, improving accountability and industry-wide standards.
✅ Recommendations for AI Developers
Disclose:
How training data was acquired (sources, licenses, human labor)
Safety risks, mitigation strategies, and evaluation methods
Cybersecurity protections, especially around model weights
Participate in:
Third-party pre-deployment evaluations
Transparent benchmarking of capabilities and risks
Adverse event reporting systems—mandatory for developers
Enable:
Independent researcher access to models for risk evaluation
Safe harbor protections for good-faith safety research
Transparent licensing terms that allow responsible research
Avoid:
Legal threats or punitive platform bans targeting researchers
Blocking disclosures of discovered vulnerabilities
✅ Recommendations for Regulators
Implement hybrid adverse event reporting systems, drawing from best practices in medical, aviation, and cybersecurity domains
Create clear thresholds for regulatory scrutiny based on:
Training compute (e.g., FLOP)
Risk evaluations and downstream impact (e.g., misuse or societal integration)
Not generic developer-level metrics like company size
Use existing regulatory tools creatively (e.g., repurpose domain-specific agencies like healthcare or labor) rather than waiting for dedicated AI-specific regulation
Institutionalize third-party risk evaluation ecosystems:
Certify evaluators
Fund research and infrastructure
Route findings through secure communication networks
Enforce transparency:
Public summaries of training data
Model capability disclosures
Safety test results
Clear consequences for false or misleading claims
Protect whistleblowers:
Establish legal rights to warn
Protect against retaliation for reporting systemic risks
💭 Additional Suggestions (Not in the Report)
Audit trail and chain of custody for training data: Require developers to maintain provenance metadata for datasets to enhance accountability and traceability.
Liability shift mechanisms: Consider differential liability schemes where safe-harbored developers may face reduced litigation risk if they meet transparency and evaluation standards.
Model registration system: Like pharmaceuticals, require registration and versioning of high-impact models before deployment, including summaries of known capabilities and limitations.
Minimum standards for deployment in critical domains: Enforce stricter evaluation before models are integrated into sectors like healthcare, education, employment, or law enforcement.
"Kill switch" protocols: Mandate developers include technical or legal mechanisms to disable or restrict high-risk models if serious harm is detected post-deployment.
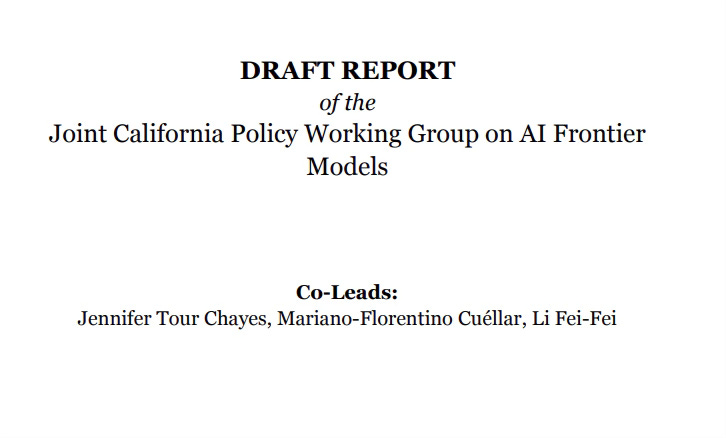