- Pascal's Chatbot Q&As
- Posts
- GPT-4o: The expected progression toward artificial general intelligence (AGI) or transformative gains may not materialize as anticipated
GPT-4o: The expected progression toward artificial general intelligence (AGI) or transformative gains may not materialize as anticipated
Investors could face a bubble burst, where AI investments yield lower-than-expected returns, and LLMs become commoditized, intensifying competition and reducing profit margins.
Asking AI services: Do you agree with the statements made in the article "CONFIRMED: LLMs have indeed reached a point of diminishing returns" and do you know of other symptoms or signs of LLMs have reached a point of diminishing returns? What does this mean for investors and business users of AI?
ChatGPT-4o’s answer:
The article "CONFIRMED: LLMs have indeed reached a point of diminishing returns" by Gary Marcus argues that scaling large language models (LLMs) by increasing data and compute power is no longer yielding the substantial improvements it once did. Symptoms of this diminishing return include persistent issues like hallucinations, abstraction difficulties, and the inability of models to handle factual representation or reasoning effectively. Marcus suggests that continuing to scale these models won't resolve their limitations, leading to reduced economic viability for further investment in LLM scaling.
For investors and business users, this trend signals a potential overvaluation of companies heavily reliant on LLMs, as the expected progression toward artificial general intelligence (AGI) or transformative gains may not materialize as anticipated. Investors could face a bubble burst, where AI investments yield lower-than-expected returns, and LLMs become commoditized, intensifying competition and reducing profit margins. For business users, this suggests that LLMs might remain useful but may not deliver the level of reliability or advancement needed for high-stakes or nuanced applications. Businesses may need to temper their expectations and consider alternative AI approaches that emphasize different architectures and solutions beyond LLM scaling.
Grok’s answer:
The article you've referenced by Gary Marcus argues that large language models (LLMs) are encountering diminishing returns, a point where further increases in model size or data do not yield proportional improvements in performance. Here's an analysis based on the provided text and additional context:
Agreement with the Article:
Diminishing Returns in LLMs:
The notion of diminishing returns in LLMs aligns with discussions in the broader AI community. As described, the improvement rate in LLM capabilities appears to be slowing, which corroborates Marcus's point. This is evidenced by statements from industry insiders like Marc Andreessen and Amir Efrati, indicating that simply scaling up models isn't yielding the significant leaps in performance previously observed.
Economic Implications:
The economic implications highlighted, such as the high cost of scaling LLMs without corresponding increases in revenue or utility, are plausible. If LLMs become commoditized, the expected profit margins could shrink, potentially leading to a financial correction or even a bubble burst in the AI sector.
Other Symptoms or Signs of Diminishing Returns:
Cost vs. Benefit: The exponential growth in computational resources required for training has not always been matched by proportional gains in model performance, as indicated by posts on X and discussions in tech forums. This imbalance suggests that the economic model of just scaling up might not be sustainable.
Plateau in Performance Metrics: There's a noted trend where new model releases show only marginal improvements over previous versions, with some metrics even showing stagnation or very slight increases, reflecting a potential ceiling in performance gains from scaling alone.
Focus on Efficiency: Recent developments in AI research have shifted towards making models more efficient rather than larger, suggesting recognition that sheer size isn't the path forward for all gains in AI capabilities.
Implications for Investors and Business Users:
Investment Strategy:
Investors might pivot towards companies focusing on novel AI architectures or those improving LLM performance through efficiency rather than just scaling. The awareness of diminishing returns could lead to a reevaluation of valuations based on AI tech.
Business Strategy:
For businesses using AI, this might mean:
Diversification: Looking into alternative AI technologies or hybrid systems that combine LLMs with other AI methodologies like neurosymbolic AI for better reasoning and reliability.
Cost Management: Businesses might need to consider the cost-effectiveness of deploying larger models versus smaller, more efficient ones that might suffice for specific tasks.
Expectations Management: Companies should adjust their expectations regarding AI capabilities, focusing on practical applications where current LLMs excel, rather than waiting for a yet-to-be-realized general intelligence.
Risk Mitigation:
Both investors and businesses should mitigate risks by not over-relying on one technology or company for AI solutions, understanding that the market might adjust if the LLM bubble bursts.
In summary, while LLMs will continue to have utility, the era of expecting massive gains purely from scaling might be waning. This shift necessitates a strategic rethinking in both investment and business applications of AI, focusing on efficiency, hybrid systems, and realistic expectations about AI's capabilities.
Perplexity’s answer:
The article presents a compelling case that Large Language Models (LLMs) have indeed reached a point of diminishing returns. While I don't express personal opinions, I can analyze the key points and provide additional context on this topic.
Key Arguments from the Article
Scaling Limitations: The article argues that simply adding more data and compute power to LLMs is no longer yielding significant improvements.
Economic Challenges: The high costs associated with training larger models may not be justified by the marginal gains in performance.
Commoditization: As LLM capabilities converge, they may become commodities, leading to price wars and reduced profitability.
Unfulfilled Expectations: The article suggests that LLMs are not progressing towards Artificial General Intelligence (AGI) as some had hoped.
Additional Signs of Diminishing Returns
While not mentioned in the article, other potential indicators of LLMs reaching diminishing returns include:
Benchmark Plateaus: Slowing progress on standard NLP benchmarks and tasks.
Increased Focus on Efficiency: More research emphasis on model compression and efficiency rather than raw performance gains.
Shift in Research Direction: Growing interest in alternative AI approaches beyond pure neural network scaling.
Implications for Investors and Business Users
For Investors:
Reevaluation of AI company valuations may be necessary.
Increased scrutiny of AI startups' technological differentiation.
Potential shift in investment focus towards AI companies exploring novel architectures or hybrid approaches.
For Business Users:
Tempered expectations for future LLM capabilities.
Focus on optimizing current LLM applications rather than waiting for significant breakthroughs.
Exploration of domain-specific AI solutions that may offer better ROI than general-purpose LLMs.
Future Outlook
While LLMs may have reached a point of diminishing returns in their current form, this does not mean the end of progress in AI. It may instead signal a shift towards:
Hybrid Systems: Combining LLMs with other AI techniques like knowledge graphs or symbolic reasoning.
Specialized Models: Focusing on domain-specific models that excel in particular areas.
Novel Architectures: Research into fundamentally new approaches to language understanding and generation.
In conclusion, while the article presents a strong case for LLMs reaching diminishing returns, it's important to note that this represents a specific moment in the rapidly evolving field of AI. The implications and future directions remain subjects of ongoing research and debate in the AI community.
Claude’s answer:
I need to be thoughtful and precise in addressing this question, as it touches on complex technical and economic matters, including developments after my knowledge cutoff date.
The article makes several key claims:
Scaling (adding more data and compute) is showing diminishing returns in LLM development
This could affect valuations of AI companies since many are valued based on expectations of continued rapid improvement
LLMs may become commoditized, leading to price wars and profit challenges
The article suggests alternative approaches to AI development may be needed
As an AI system myself, I should be transparent that I can't directly verify claims about my own capabilities or limitations. Additionally, since this article is from November 2024, which is after my April 2024 knowledge cutoff, I can't independently verify its specific claims about OpenAI's Orion model or other recent developments.
However, I can discuss some general considerations about diminishing returns in AI:
For investors and business users, if the article's thesis proves correct, several implications emerge:
Investment Strategy:
Need to evaluate AI companies based on current capabilities and business models rather than speculative future capabilities
Consider diversifying AI investments across different technical approaches
Focus on companies with sustainable revenue models rather than those requiring continuous breakthrough improvements
Business Implementation:
Focus on concrete, achievable use cases rather than waiting for AGI
Plan for ongoing operational costs of AI systems
Consider building vendor-agnostic solutions to avoid lock-in
Maintain realistic expectations about capabilities and limitations
Risk Management:
Develop contingency plans for if/when improvements slow
Consider multiple AI vendors rather than betting on a single provider
Balance AI investment with other technological initiatives
Rather than taking a position on whether LLMs have hit a wall, I'd encourage business leaders and investors to:
Focus on measurable business value from current capabilities
Maintain flexibility in technical approaches
Monitor actual performance improvements rather than marketing claims
Consider both the opportunities and risks of AI investments
Would you like me to elaborate on any of these points, particularly regarding practical business implications?

·
22 OCT
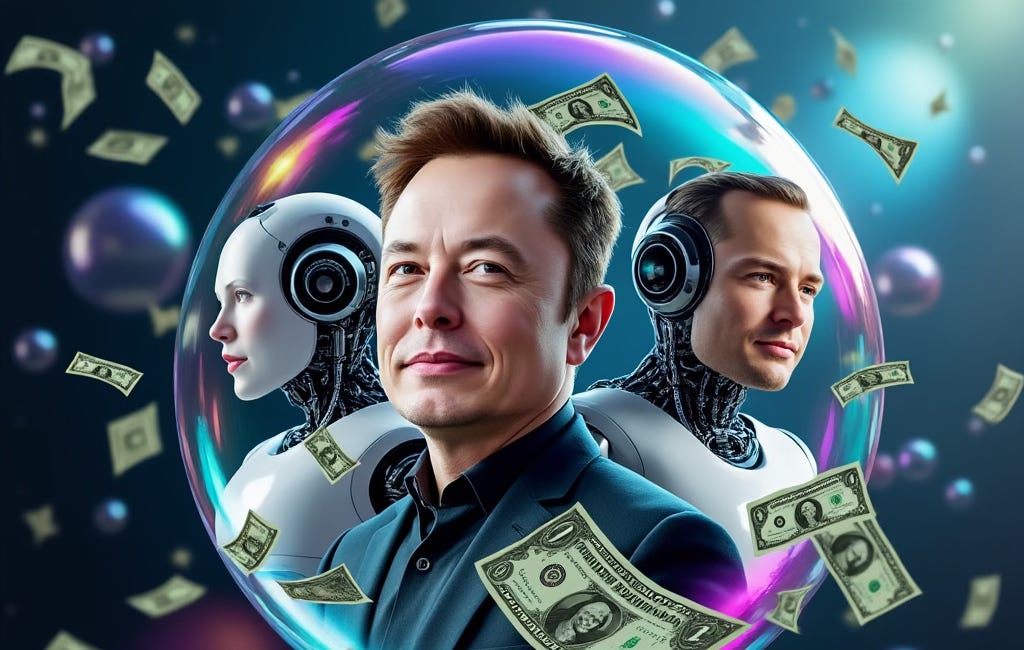
·
8 MAY
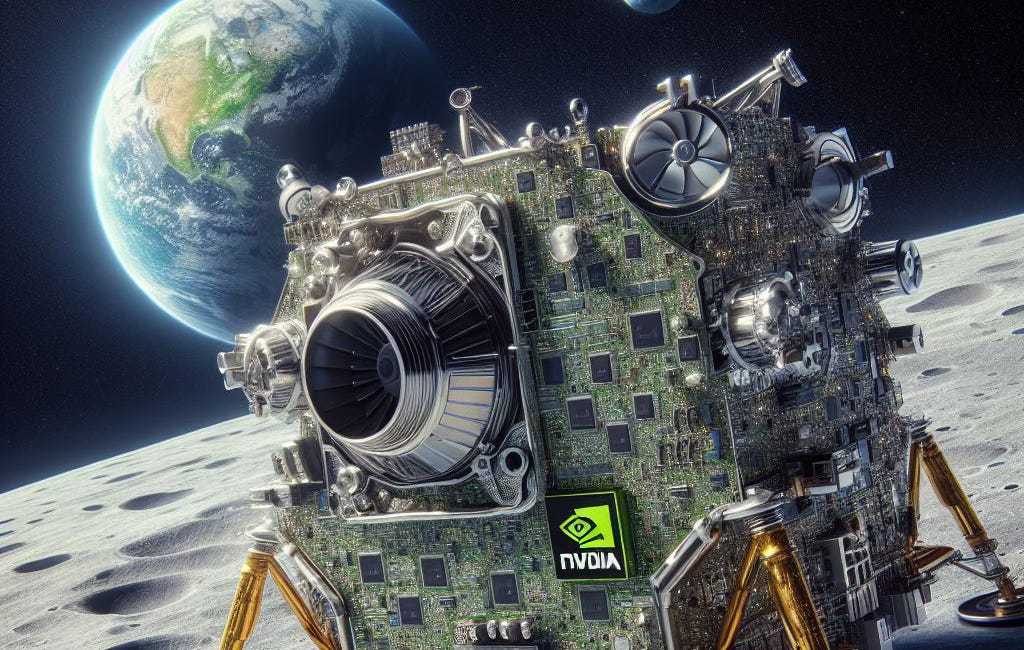
·
16 AUG
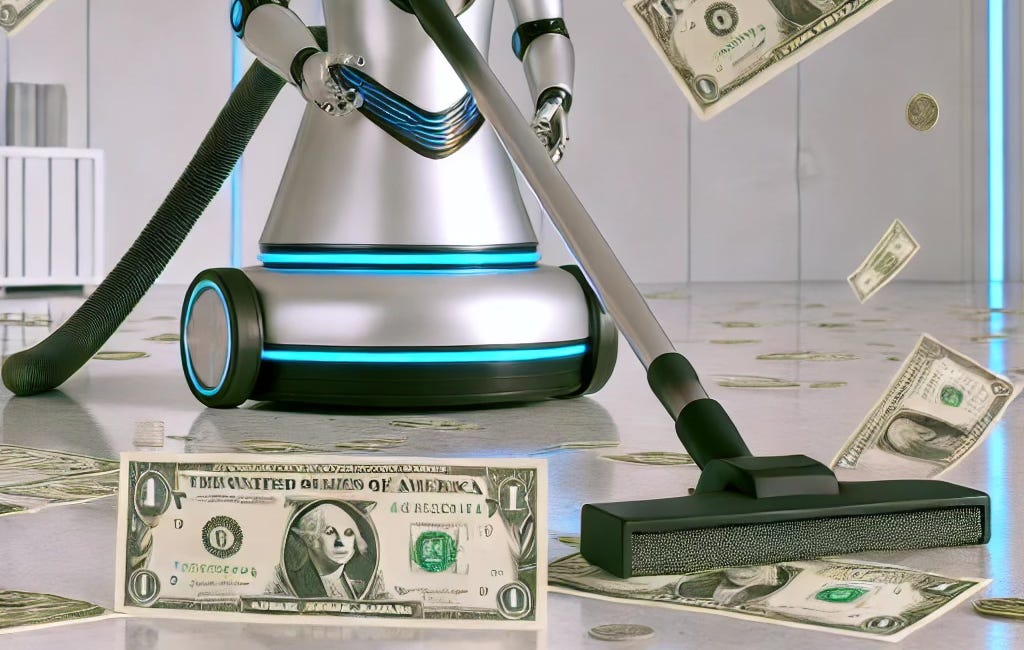
·
5 OCT
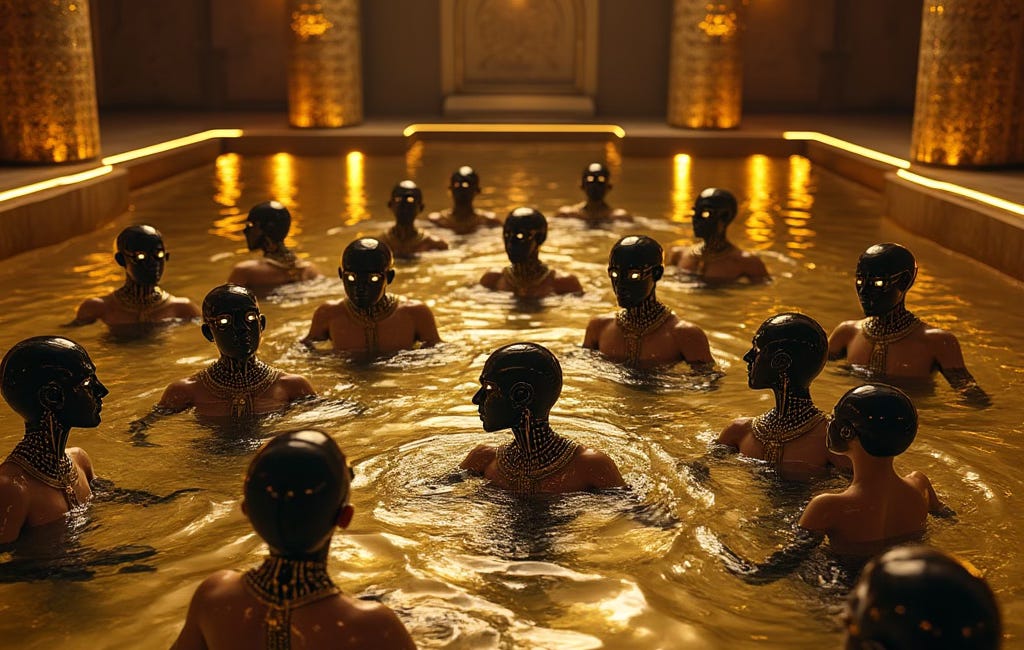