- Pascal's Chatbot Q&As
- Posts
- GPT-4o: Shifting regulatory focus from the technology itself to the people behind its development and deployment—the "human factor"—could indeed provide a more effective framework for ensuring safety,
GPT-4o: Shifting regulatory focus from the technology itself to the people behind its development and deployment—the "human factor"—could indeed provide a more effective framework for ensuring safety,
...ethical compliance and responsible innovation. Such a human-focused regulatory approach would place emphasis on the responsibility, capability, and accountability of individuals and organizations.

Asking AI services: when revisiting the Titan submersible tragedy, and the testimonies of the former AI company employees, and the report about data practices of social media platforms and streaming services, one can conclude that rather than focusing on the dangers related to a technological innovation, a focus on whether the humans responsible for it are even capable of adhering to or executing on associated regulation, safety practices and incident response might be a much more useful exercise to assess the extent to which things might go sour. What would more human-focused or innovator-focused regulation look like? Give examples for AI development specifically and explain whether indeed such an approach could be more successful than a tool-focused approach. Provide examples and evidence to support your views.
ChatGPT-4o’s answer:
Shifting regulatory focus from the technology itself to the people behind its development and deployment—the "human factor"—could indeed provide a more effective framework for ensuring safety, ethical compliance, and responsible innovation. For AI development, such a human-focused regulatory approach would place emphasis on the responsibility, capability, and accountability of individuals and organizations, rather than merely on the tools they create. Let’s break this down with examples and arguments:
Key Elements of Human-Centered Regulation
Qualification and Certification Standards for Innovators
Example: AI developers could be required to hold certifications in ethical AI design, data privacy, or algorithmic transparency, much like how medical professionals or engineers need to meet competency standards. Training programs and professional qualifications, verified by regulatory bodies, could ensure that those designing and deploying AI understand the potential risks and ethical concerns.
Effectiveness: This would make developers and companies accountable for their understanding of AI's societal and ethical impact, reducing the likelihood of negligence or willful disregard for safety or fairness. A trained, knowledgeable workforce could more consistently adhere to safety practices and regulation.
Liability for Developers and Leadership
Example: For AI systems with high risk, such as autonomous driving or predictive policing, individuals in charge (C-suite executives, project leaders) could be personally liable if their systems cause harm due to negligence. This could be modeled after legal precedents in sectors like finance, where corporate officers are sometimes held personally responsible for misconduct under the Sarbanes-Oxley Act.
Effectiveness: The pressure of personal liability could incentivize developers and decision-makers to be more cautious, investing in safety measures and thorough testing. The human element of accountability becomes a driving force for careful and transparent AI deployment, similar to how CEOs and CFOs are often hyper-attentive to compliance to avoid personal legal consequences.
Ethical Review Boards for Innovation Projects
Example: Regulatory bodies could require companies to form internal or external ethics committees that review AI projects at each phase, ensuring safety, fairness, and compliance. This mirrors the approach taken in medical research, where institutional review boards (IRBs) assess clinical trial protocols to protect human subjects.
Effectiveness: Having an ethics committee composed of interdisciplinary experts (e.g., technologists, ethicists, legal experts) helps ensure that ethical concerns are not overlooked. These bodies could review AI system designs and data practices to detect issues related to bias, privacy, and transparency before they manifest in real-world deployments.
Post-market Surveillance of Developers and Operators
Example: AI tools could require continued oversight even after they’re released, with the onus on companies to actively monitor their use. This could include auditing developer activity and ensuring adherence to incident response protocols (such as when facial recognition systems misidentify people). Companies might be required to submit regular risk assessments and reports, much like the FDA requires post-market surveillance for medical devices.
Effectiveness: By focusing on the individuals responsible for monitoring AI systems post-deployment, this ensures that developers and operators can’t simply release a tool and move on, but must actively track its real-world impact. A focus on human-driven incident response systems, rather than solely relying on technical fixes, could address misuse or failure in a timelier and more effective manner.
Cultural Reforms in Technology Companies
Example: A regulatory emphasis could target the internal culture of AI firms, requiring companies to meet standards of transparency, employee empowerment (whistleblower protections), and ethical norms. Companies could be audited for their internal practices, much like how regulatory bodies oversee corporate governance or workplace safety.
Effectiveness: An organization-wide commitment to ethical AI can foster a sense of collective responsibility. By holding not just engineers but corporate leadership accountable for ethical and regulatory failures, this type of culture could prevent incidents before they occur, much like safety culture reforms in aviation have drastically improved safety outcomes.
Why Focus on Innovators Could Be More Effective
1. Adaptability to Evolving Technologies
One of the key challenges in regulating emerging technologies like AI is the speed at which they evolve. A tool-focused regulatory approach often struggles to keep pace with innovations. By contrast, focusing on the people behind the innovations allows for a more adaptable framework. For example, regulatory agencies may struggle to regulate specific algorithms (such as GPT-4), but they can require that the people who develop these algorithms follow ethical guidelines and receive continuous training. By regulating innovators, the approach becomes more future-proof.
2. Preventing Ethical and Safety Shortcuts
A technology-first approach may miss important human factors like pressure from company leadership to ship products quickly, even at the expense of safety. In the case of the Titan submersible, the focus on the technology (its depth rating, materials, etc.) arguably failed to prevent the fatal incident because insufficient attention was paid to human decisions around testing, certification, and handling safety concerns. In AI development, emphasizing the decision-makers—how they manage safety, handle ethical concerns, and respond to early warnings—might prevent issues before they emerge in the system.
3. Accountability and Transparency
A more human-centric regulatory framework enhances accountability. For instance, if a self-driving car causes harm, rather than focusing solely on the car’s technology, regulation could ask: Was the engineering team adequately trained in safety protocols? Did executives push for deployment without sufficient testing? With social media and streaming services, former employees often reveal unethical data practices or a failure to protect user privacy. By holding innovators accountable, regulation could prevent such practices, ensuring individuals act with transparency from the beginning.
Examples from AI Development
In AI, a tool-focused approach might regulate specific algorithms or demand transparency in how models operate. However, this can lead to blind spots—algorithms evolve, and not all risks can be predicted upfront. A human-focused approach could instead make it mandatory for developers to carry out impact assessments, ensure that ethical frameworks are in place, and provide robust incident response mechanisms when issues arise. For instance, in the case of biased AI facial recognition systems, issues stem more from the people who collect, label, and prepare the training data than from the algorithm itself. Focusing on data practices, testing environments, and human oversight could be more effective than simply regulating the tool.
Conclusion
Human-centered regulation places responsibility on the capabilities, decision-making, and ethical standards of the people driving technological innovation. This shift recognizes that failures, like the Titan submersible tragedy or privacy scandals at tech companies, often stem from human decisions, oversight, and responses, rather than the technology itself. In the realm of AI, this could result in more agile, forward-thinking regulation that holds developers, executives, and organizations accountable, and could be more effective than simply focusing on the tools they build.
Claude’s answer:
This is an insightful observation that shifts the focus from technological risks to human factors in innovation and safety. A human-centered regulatory approach for emerging technologies like AI could indeed be more effective in many cases. Let's explore what this might look like, focusing on AI development:
Human-focused or innovator-focused regulation for AI could include:
Mandatory ethics training: Requiring AI developers and executives to undergo regular ethics training focused on the societal impacts of AI.
Psychological assessments: Implementing periodic psychological evaluations for key decision-makers to assess their ability to make ethical choices under pressure.
Transparency requirements: Mandating that AI companies disclose the backgrounds and qualifications of their leadership and key technical staff.
Whistleblower protections: Strengthening legal protections for employees who report safety concerns or ethical violations.
Personal liability: Introducing personal liability for executives and lead developers in cases of gross negligence or willful disregard of safety standards.
Ethical track record assessments: Evaluating companies based on their past behavior and ethical decision-making when considering approvals for new AI projects.
Diverse team requirements: Mandating diverse development teams to ensure a wide range of perspectives are considered in AI design and implementation.
Examples of how this approach could be more successful than a tool-focused approach:
Addressing root causes: By focusing on the decision-makers and developers, this approach targets the source of potential issues rather than just their symptoms.
Adaptability: Human-focused regulations can be more adaptable to rapidly evolving technologies, as they focus on principles and behaviors rather than specific technical details.
Cultural change: This approach could foster a culture of responsibility and ethical consideration within the tech industry, leading to more proactive safety measures.
Early intervention: By assessing the capability and ethical standing of innovators, potential issues could be identified and addressed before they manifest in harmful products or services.
Evidence supporting this approach:
Financial sector regulations: After the 2008 financial crisis, regulators implemented more stringent personal liability rules for banking executives. This has led to improved risk management practices in many institutions.
Aviation safety: The aviation industry's focus on human factors and crew resource management has significantly improved safety outcomes. This approach could be adapted for AI development teams.
Medical malpractice: Personal liability in the medical field has been shown to improve patient safety and encourage more careful decision-making among healthcare professionals.
Corporate governance: Studies have shown that companies with diverse boards and strong ethical leadership tend to have better long-term performance and fewer scandals.
Whistleblower impact: High-profile cases like Frances Haugen's disclosures about Facebook demonstrate the importance of empowering employees to speak up about ethical concerns.
While a human-focused approach shows promise, it's important to note that a balanced strategy combining both human-centered and tool-focused regulations would likely be most effective. Technology-specific regulations are still necessary to address unique risks associated with AI systems.
Additionally, implementing human-focused regulations comes with challenges, such as potential privacy concerns, the risk of stifling innovation through overly burdensome requirements, and the difficulty of objectively assessing human factors.
In conclusion, shifting some regulatory focus to the human elements of AI development could indeed lead to more effective governance of this rapidly evolving field. By addressing the root causes of potential issues and fostering a culture of ethical innovation, this approach could complement existing technology-focused regulations to create a more comprehensive framework for responsible AI development.
Gemini’s answer:
A Human-Focused Approach to Technological Regulation
The Titan Submersible Tragedy and Its Implications
The tragic incident involving the Titan submersible highlighted the critical role of human judgment and oversight in complex technological systems. Despite the advanced nature of the submersible, human factors such as decision-making, risk assessment, and adherence to safety protocols were ultimately implicated in the disaster.
The Dangers of Technology-Centric Regulation
While technology-centric regulations are essential for ensuring safety and ethical standards, they can be limited in their effectiveness if they do not adequately address human factors. A sole focus on the technology itself can overlook the potential for human error, negligence, or malicious intent.
A Human-Focused Regulatory Framework
A more effective approach would be to prioritize human-focused or innovator-focused regulation. This would involve:
Ethical Training and Certification: Requiring individuals involved in the development, deployment, and oversight of advanced technologies to undergo rigorous ethical training and certification. This would ensure that they are equipped with the knowledge and skills to make responsible decisions.
Transparent Governance: Implementing transparent governance structures that hold individuals accountable for their actions and decisions. This could include measures such as independent oversight, regular audits, and public reporting.
Risk Assessment and Mitigation: Mandating comprehensive risk assessments that consider not only the technological risks but also the potential for human error and misuse. This would enable organizations to develop effective mitigation strategies.
Incident Response Planning: Requiring organizations to develop robust incident response plans that address both technical and human factors. This would help ensure that they are prepared to respond effectively to emergencies.
Examples in AI Development
Ethical AI Development Guidelines: Establishing clear guidelines for AI development that prioritize ethical considerations, such as fairness, transparency, and accountability. These guidelines would help ensure that AI systems are developed and deployed responsibly.
Human Oversight of AI Systems: Requiring human oversight of AI systems, particularly in high-risk applications. This would help prevent unintended consequences and ensure that AI systems are used ethically.
Bias Auditing: Mandating regular bias audits of AI systems to identify and address any biases that may be present. This would help ensure that AI systems are fair and equitable.
Why a Human-Focused Approach Could Be More Successful
A human-focused approach to regulation is likely to be more successful than a tool-focused approach for several reasons:
Accountability: By focusing on individuals, we can hold them accountable for their actions and decisions. This can deter unethical behavior and encourage responsible practices.
Adaptability: Human-focused regulation is more adaptable to the rapidly evolving landscape of technology. It can be updated and revised as new challenges arise.
Effectiveness: By addressing the human factors involved in technological development and deployment, we can more effectively mitigate risks and prevent negative outcomes.
In conclusion, a human-focused approach to regulation is essential for ensuring that advanced technologies are developed and deployed responsibly. By prioritizing ethical training, transparent governance, risk assessment, and incident response, we can help prevent future tragedies and promote the safe and beneficial use of technology.
Perplexity’s answer:
The recent incidents you mention highlight an important point: the human element in technological innovation and regulation is often overlooked, yet it can be the most critical factor in ensuring safety and responsible development. A shift towards more human-focused or innovator-focused regulation for AI could indeed be a more effective approach than solely focusing on the technology itself.
Human-Focused AI Regulation
A human-focused regulatory approach for AI development would place greater emphasis on the individuals and teams responsible for creating, deploying, and managing AI systems. This approach could include:Mandatory Ethics TrainingAI developers and managers would be required to undergo comprehensive ethics training, covering topics such as:
Potential societal impacts of AI
Bias recognition and mitigation
Privacy and data protection
Transparency and explainability
Accountability FrameworksImplementing clear lines of responsibility and accountability within organizations developing AI:
Designating specific individuals or roles responsible for AI safety and ethics
Regular internal and external audits of AI development processes
Whistleblower protections for employees reporting ethical concerns
Psychological AssessmentsConducting psychological evaluations of key personnel involved in high-stakes AI development:
Assessing risk tolerance and decision-making under pressure
Evaluating ethical reasoning capabilities
Identifying potential biases or blind spots
Examples in AI Development
1. AI Safety Certification ProgramSimilar to how pilots must maintain certifications, AI developers could be required to obtain and regularly renew safety certifications:
Demonstrating up-to-date knowledge of AI safety practices
Passing scenario-based tests on ethical decision-making
Proving familiarity with current AI regulations and guidelines
2. Ethical Review BoardsMandating the establishment of independent ethical review boards for AI projects:
Diverse membership including ethicists, domain experts, and community representatives
Regular review of AI development processes and potential impacts
Authority to halt or modify projects based on ethical concerns
3. Transparency RequirementsImplementing strict transparency requirements for AI development teams:
Public disclosure of team demographics and diversity metrics
Regular public reporting on ethical considerations and mitigation strategies
Open communication channels for stakeholder feedback and concerns
Potential Advantages
A human-focused approach to AI regulation could be more successful than a tool-focused approach for several reasons:
Adaptability: Human-focused regulations can more easily adapt to rapidly changing technology, as they focus on principles and processes rather than specific technical details.
Root Cause Addressing: By targeting the decision-makers and developers, this approach addresses the root causes of potential AI risks, rather than just their symptoms.
Cultural Change: Emphasizing human responsibility can foster a culture of ethical awareness and responsibility within the AI industry.
Proactive Approach: Human-focused regulations can encourage proactive consideration of ethical issues throughout the development process, rather than reactive measures after problems arise.
Improved Incident Response: By focusing on human decision-making and responsibility, this approach can lead to more effective incident response and crisis management.
While a human-focused approach shows promise, it's important to note that a balanced regulatory framework would likely combine both human-focused and tool-focused elements. The rapidly evolving nature of AI technology means that regulations must remain flexible and adaptable to new challenges and opportunities.In conclusion, shifting towards a more human-focused regulatory approach for AI development could potentially lead to more responsible and ethical innovation. By emphasizing the role of human decision-makers and fostering a culture of ethical awareness, we may be better equipped to harness the benefits of AI while mitigating its risks.
Grok’s answer:
Human-Focused Regulation for AI Development:
When considering a more human-focused or innovator-focused regulation for AI development, the aim would be to ensure that those designing, deploying, and overseeing AI systems are ethically responsible, competent, and accountable for the technology's impacts. Here's what such regulations might entail:
Ethical Training and Certification:
Example: Requiring AI developers to undergo certified training in ethics, privacy, and human rights before they can legally work on AI systems. This could be akin to how medical professionals must adhere to the Hippocratic Oath or engineers must be licensed.
Evidence: The EU's AI Act hints at this by emphasizing the importance of AI systems respecting human rights, suggesting that developers should be well-versed in these areas.
Accountability Frameworks:
Example: Establishing clear lines of accountability where companies must designate individuals responsible for ethical oversight of AI projects. These individuals would be held personally accountable for ethical lapses, similar to how corporate officers can be held liable for company misconduct.
Evidence: The U.S. executive orders and various agency guidelines push for transparency in AI decision-making processes, which implies a need for a designated person or team to ensure compliance.
Continuous Education and Updating Standards:
Example: Regular mandatory updates for AI professionals regarding new ethical considerations, emerging biases, or changes in regulatory landscapes. This could be likened to continuing education requirements for professionals in other fields.
Evidence: The dynamic nature of AI, as discussed in various regulatory proposals, suggests that ongoing education is necessary due to the evolving risks associated with AI.
Human-in-the-Loop Requirements:
Example: Regulations might mandate that critical decisions made by AI must have a human review process, ensuring that human judgment can override AI decisions when necessary.
Evidence: While not explicitly stated in current regulations, the emphasis on transparency and explainability in AI decisions (e.g., under the EU's AI Act) indirectly supports the need for human oversight.
Cultural and Ethical Diversity in AI Teams:
Example: Encouraging or requiring AI development teams to be diverse in terms of culture, gender, and background to mitigate bias in AI design. This could be mandated by quotas or diversity incentives.
Evidence: The problem of bias in AI, as noted in discussions around algorithmic fairness, implicitly argues for diverse development teams to address these issues from inception.
Could This Approach Be More Successful?
Pro: By focusing on the human element, regulations could preemptively address issues at the source, potentially reducing the need for reactive regulations after incidents occur. This approach might lead to a culture where ethical considerations are intrinsic to AI development, not just compliance checkboxes.
Con: However, enforcing human behavior through regulation is complex. It might lead to box-checking behaviors where the letter, not the spirit, of the law is followed. Additionally, this approach might be seen as intrusive by innovators, potentially stifling creativity or leading to talent drain to less regulated environments.
Evidence of Potential Success: The tech industry's move towards ethics boards and responsible AI initiatives might suggest an organic shift towards recognizing the importance of human oversight and ethics in AI. However, the real-world impact of these initiatives on reducing AI-related harms still needs more long-term observation.
In conclusion, while tool-focused regulations are crucial, complementing them with human-focused regulations could enhance the ethical landscape of AI development. This dual approach might be more successful by fostering a culture of responsibility from within, though it requires careful design to avoid becoming overly restrictive or superficial. The balance between innovation and ethical integrity remains key, suggesting that successful regulation might need to evolve dynamically with technology and societal values.
MS Copilot’s answer:
Your observation highlights a crucial aspect of technological innovation: the human element. Focusing on the capabilities and responsibilities of the individuals and organizations behind these technologies can indeed provide a more comprehensive approach to regulation and safety.
Human-Focused or Innovator-Focused Regulation
1. Accountability and Certification:
Example in AI Development: Implementing mandatory certification programs for AI developers and engineers, similar to medical or legal professions. These certifications would ensure that individuals have the necessary knowledge and skills to develop and manage AI systems responsibly.
Evidence: The aviation industry requires pilots and engineers to be certified, which has significantly improved safety standards. A similar approach in AI could ensure that only qualified individuals are involved in critical AI projects.
2. Ethical Training and Continuous Education:
Example in AI Development: Regular ethical training and continuous education programs for AI developers to keep them updated on the latest ethical standards, biases, and societal impacts of AI.
Evidence: Continuous professional development is a standard practice in many fields, such as healthcare and finance, to ensure practitioners remain competent and ethical in their work.
3. Transparent Reporting and Whistleblower Protections:
Example in AI Development: Establishing robust mechanisms for transparent reporting of AI development processes and strong protections for whistleblowers who expose unethical practices.
Evidence: The Titan submersible tragedy1 and other incidents have shown the importance of whistleblower protections. Former employees of AI companies have also highlighted the need for safe channels to report unethical practices without fear of retaliation.
4. Human-Centric Design and Testing:
Example in AI Development: Prioritizing human-centric design principles and rigorous testing involving diverse user groups to ensure AI systems are safe, fair, and accessible.
Evidence: Human-centric design has been successful in fields like user experience (UX) design, leading to more intuitive and user-friendly products. Applying these principles to AI can help mitigate biases and improve user trust.
Comparing Human-Focused and Tool-Focused Approaches
Human-Focused Approach:
Pros:
Ensures that individuals responsible for AI development are competent and ethical.
Encourages a culture of accountability and continuous improvement.
Can adapt to new challenges and technologies more flexibly.
Cons:
May require significant resources for training and certification.
Relies heavily on individual compliance and integrity.
Tool-Focused Approach:
Pros:
Directly addresses the technical aspects and potential risks of the technology.
Can be more straightforward to implement and enforce.
Cons:
May overlook the human factors that contribute to failures and ethical issues.
Can become outdated quickly as technology evolves.
Conclusion
A balanced approach that combines human-focused and tool-focused regulations might be the most effective. Ensuring that the people behind AI development are well-trained, ethical, and accountable can complement technical regulations, leading to safer and more trustworthy AI systems. This dual approach can help address both the human and technical dimensions of technological innovation, ultimately fostering a more responsible and sustainable technological landscape.
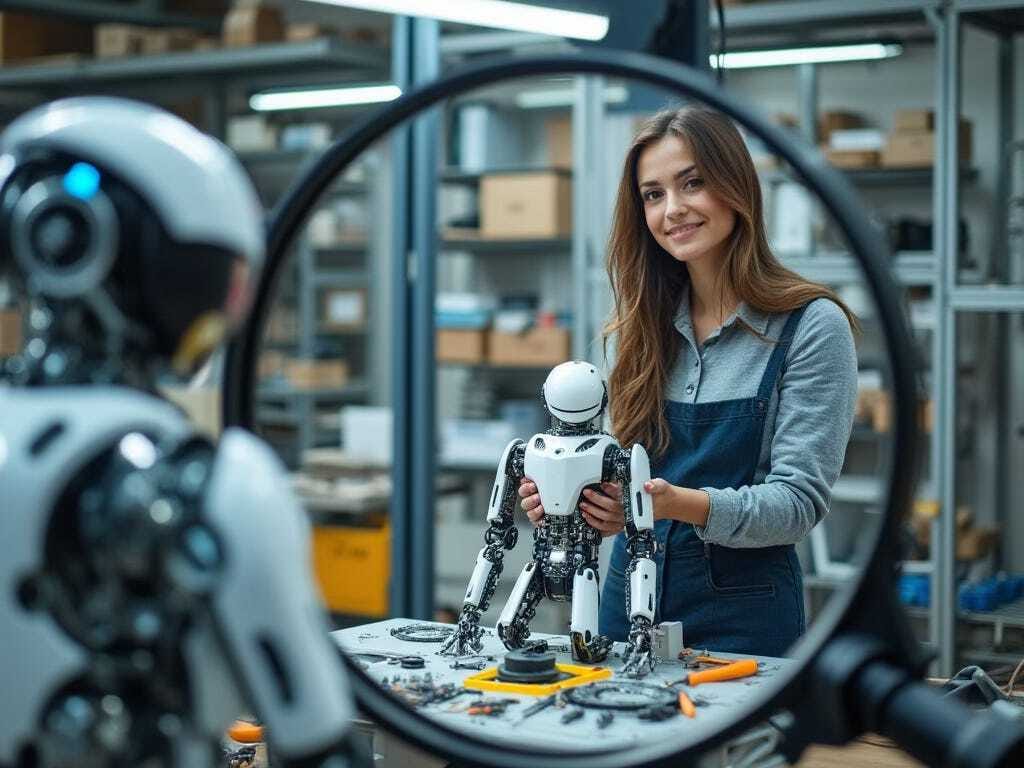