- Pascal's Chatbot Q&As
- Posts
- GPT-4o: OpenAI’s most advanced models appear to have been trained on copyrighted books behind paywalls — without permission — and that’s a big legal and ethical problem.
GPT-4o: OpenAI’s most advanced models appear to have been trained on copyrighted books behind paywalls — without permission — and that’s a big legal and ethical problem.
This report delivers a wake-up call for both content owners and AI developers. The authors call for new rules and markets to make sure creators are fairly treated in the AI ecosystem.
Question 1 of 3 for ChatGPT-4o: Please read the report "Beyond Public Access in LLM Pre-Training Data. Non-public book content in OpenAI’s Models" and tell me what it says in easy to understand language
ChatGPT-4o’s answer:
The report “Beyond Public Access in LLM Pre-Training Data: Non-public book content in OpenAI’s Models” by Sruly Rosenblat, Tim O’Reilly, and Ilan Strauss investigates whether OpenAI used copyrighted, non-public book content from O’Reilly Media to train its AI models without permission. Here's a simplified breakdown of what the report says:
🧠 What the researchers did
They legally obtained 34 copyrighted O’Reilly Media books, which include both public and paywalled (non-public) parts.
They tested three OpenAI models: GPT-3.5 Turbo, GPT-4o, and GPT-4o Mini to see if those models “recognized” the books, which would suggest they were trained on them.
The key method used is called a “membership inference attack” (DE-COP), which checks if an AI model has “seen” or memorized certain texts before.
📊 Key Findings
GPT-4o (the most recent model) seems to strongly recognize non-public, paywalled content, more so than public content.
It scored 82% accuracy in identifying non-public text from its training set (compared to random chance at 50%).
It did better on paywalled data than public previews, which is suspicious because you'd expect the model to mostly learn from freely available data.
GPT-3.5 Turbo (older model) didn’t show this same pattern.
It recognized public content more than non-public, suggesting earlier OpenAI models may have been more careful with copyright.
GPT-4o Mini (smaller model) showed no real recognition, likely because it's too small to memorize much — not necessarily because it wasn’t trained on the content.
🔍 How do they know this?
They split the book paragraphs into public vs. non-public and also by publication date — if a book came out after the model was trained, then it definitely couldn’t have been used.
By comparing models trained in the same timeframe (e.g. GPT-4o and GPT-4o Mini), they could rule out “language style over time” as the reason for differences.
⚠️ Why this matters
The findings suggest OpenAI’s newer models are likely trained on copyrighted, paywalled content without permission.
This practice may violate copyright laws and threatens the sustainability of content creation, since creators aren’t being paid or credited.
The report warns that this could lead to an “extractive dead end,” where AI depletes the internet’s creative resources and undermines quality content production.
🔧 Policy and market recommendations
The authors argue for more transparency from AI companies about where their training data comes from.
They suggest creating formal licensing markets for training data — where content creators get paid.
They highlight that the EU AI Act might help push companies toward better disclosure standards.
🧾 Bottom Line
OpenAI’s most advanced models appear to have been trained on copyrighted books behind paywalls — without permission — and that’s a big legal and ethical problem. The authors call for new rules and markets to make sure creators are fairly treated in the AI ecosystem.
Question 2 of 3 for ChatGPT-4o: List the most surprising, controversial and valuable statements and findings in the report.
ChatGPT-4o’s answer:
Here’s a list of the most surprising, controversial, and valuable statements and findings from the report “Beyond Public Access in LLM Pre-Training Data: Non-public book content in OpenAI’s Models”:
🔥 Most Surprising Findings
GPT-4o shows higher recognition of paywalled content than public content
GPT-4o scored 82% AUROC on paywalled O’Reilly books, vs. 64% on public excerpts.
⟶ This is surprising because you'd expect AI models to mostly learn from public internet data.
Recognition increased over time in newer models
GPT-3.5 Turbo showed little recognition of paywalled content, but GPT-4o showed strong signs of having been trained on it.
⟶ Suggests that OpenAI’s data sourcing practices may have changed — for the worse — as newer models came online.
GPT-4o Mini (a smaller model) showed no such recognition
Despite being trained at the same time as GPT-4o, it performed at random chance levels (~50%) on both public and non-public data.
⟶ Surprising hint that model size affects whether copyright violations are detectable.
⚖️ Most Controversial Claims
OpenAI likely trained GPT-4o on non-public, copyrighted content without permission
The study provides "empirical evidence" that OpenAI’s most capable model learned from copyrighted O’Reilly Media books behind paywalls.
Use of LibGen (a pirate database) may be the access point
All 34 tested O’Reilly books were found on LibGen, which the authors speculate may have been a source of unauthorized training data.
OpenAI and others are lobbying to exempt model training from copyright altogether
If successful, this would legally protect AI developers even if they trained on pirated or paywalled data, gutting copyright enforcement in the AI era.
A tragedy of the commons is underway
Tim O’Reilly calls this an “extractive dead end” — where AI firms cannibalize the internet’s creative ecosystem without contributing back, threatening its survival.
💡 Most Valuable Contributions
First-of-its-kind use of legally obtained, non-public data to detect access violations
Prior studies used public or synthetic data; this one used 34 actual, licensed copyrighted books to test real-world violations.
Strong evidence supporting the need for AI training data disclosure laws
The study directly supports regulatory efforts like the EU AI Act, which will require model developers to summarize their training data sources in 2026.
Proposes a market-based solution: functional AI content licensing ecosystems
Calls for structured commercial marketplaces where publishers and authors can license training data — with attribution and compensation mechanisms built-in.
Recommends technical audits like DE-COP to enforce accountability
Suggests that tools like membership inference attacks can pressure AI developersinto fairer data practices — though the method alone isn’t enough, especially for small or post-trained models.
Question 3 of 3 for ChatGPT-4o: Explain how publishers and regulators can make use of this information. Also, explain how AI developers should act in case they want to prevent liability as a result of this situation.
ChatGPT-4o’s answer:
Great question — this report delivers a wake-up call for both content owners and AI developers. Here’s a breakdown of how publishers, regulators, and AI developerscan make strategic use of these findings:
📚 For Publishers & Copyright Holders
🛠️ What they can do with this report:
Use the methodology as evidence
The DE-COP method used in the report shows that unauthorized training is detectable.
Publishers can replicate or commission similar studies using their own books or articles to build proof of infringement.
Pressure AI companies for licensing agreements
The report shows that non-public content improves model quality, making a clear economic case for licensing.
Publishers can leverage this to negotiate royalties, tiered access deals, or content usage limits.
Support disclosure requirements
Advocate for laws (like the EU AI Act) to be enforced or expanded to require detailed training data disclosures — not just vague summaries.
Join or initiate litigation or lobbying efforts
This report helps validate lawsuits (e.g. NYT v. OpenAI) and undermines the "fair use" defense.
It supports coalition-building among content producers to pursue legal reforms or collective bargaining rights.
Experiment with licensing marketplaces
Explore platforms like Spawning.ai, News Licensing Group, or Music AI licensing tools to monetize content used for AI training.
🏛️ For Regulators & Policymakers
🎯 How they can use the findings:
Mandate stronger transparency standards
Require AI companies to publish detailed training data audits or use tools like DE-COP to verify claims.
Push for third-party certification of data provenance.
Clarify copyright liability
Ensure that training on non-public content without consent is explicitly illegal.
Introduce safe harbor conditions for AI developers who use licensed or traceable datasets.
Build technical oversight capacity
Fund or support data auditing tools and institutions (e.g., academic or nonprofit watchdogs).
Set up regulatory sandboxes for AI data governance experimentation.
Promote market-based solutions
Create incentives or standards for the development of trusted content marketplaces where rights can be negotiated and tracked.
🤖 For AI Developers
⚠️ What they should do to avoid legal risk:
Stop using pirated or unauthorized data
Avoid scraping from sources like LibGen, Sci-Hub, or sites protected by robots.txt and TOS.
Audit current datasets — even retrospectively — to identify problematic content.
Build from licensed or “clean” datasets
Shift toward using datasets like Common Corpus, LAION with verified licenses, or purpose-built licensed corpora.
Disclose training sources voluntarily
Proactively share detailed breakdowns of training data — even before regulators require it.
Use model cards with dataset descriptions and audit logs.
Engage in good-faith negotiations
Partner with content providers and publishers to license data ethically and legally.
Consider revenue-sharing models for outputs derived from valuable proprietary content.
Develop internal risk assessments
Integrate compliance reviews into the data pipeline, especially for foundation model training.
Track not just input sources but how they affect output liability (e.g., reproducing long book passages).
🧩 Bottom Line:
This report is a smoking gun showing that:
Non-public content improves model performance
That content was likely used without permission
It’s now technically feasible to detect misuse
That means:
Publishers have a stronger legal and commercial hand.
Regulators have a clear signal to act.
Developers must clean up their pipelines — or face lawsuits, fines, and reputational damage.
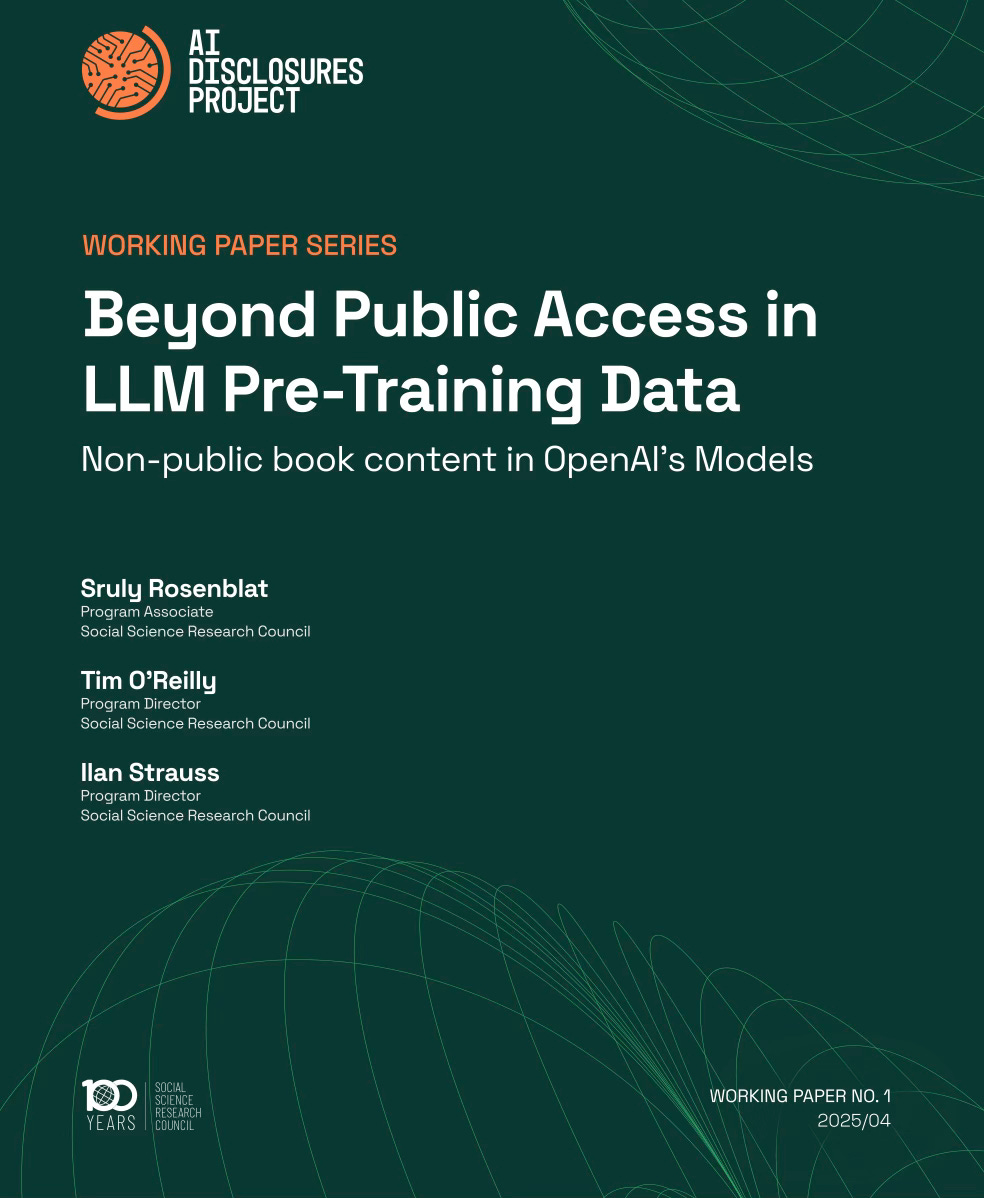