- Pascal's Chatbot Q&As
- Posts
- GPT-4o: Meta identified books as the most valuable dataset for training LLMs. Books were considered more important than web data. Meta Downloaded & Trained on More than 267 Terabytes of Pirated Books.
GPT-4o: Meta identified books as the most valuable dataset for training LLMs. Books were considered more important than web data. Meta Downloaded & Trained on More than 267 Terabytes of Pirated Books.
This dataset includes tens of millions of copyrighted works, making it equivalent to the entire Library of Congress 20 times over. Meta routed its torrenting through AWS to avoid detection.
Question 1 of 3 for ChatGPT-4o: Please read the article “The Unbelievable Scale of AI’s Pirated-Books Problem - Meta pirated millions of books to train its AI. Search through them here” and tell me what it says. List the most surprising, controversial and valuable statements made.
ChatGPT-4o’s answer:
Here are the most surprising, controversial, and valuable statements from The Atlantic's article, The Unbelievable Scale of AI’s Pirated-Books Problem:
Most Surprising Statements
Meta executives actively discussed pirating books – Internal messages show that Meta employees considered licensing books but found it too expensive and slow. Instead, they chose to download books from Library Genesis (LibGen), a massive repository of pirated books and academic papers.
Meta’s AI team received permission from Mark Zuckerberg – The article reveals that “MZ” (presumably Mark Zuckerberg) approved the use of pirated materials for AI training.
Meta downloaded over 7.5 million books and 81 million research papers – The sheer scale of copyrighted material used without permission is staggering.
Most Controversial Statements
Meta used BitTorrent to acquire the pirated books, possibly redistributing them – The article suggests that downloading from BitTorrent typically involves uploading, which could mean that Meta was not only acquiring pirated books but also distributing them, an explicitly illegal act under copyright law.
Meta employees sought ways to "mask" their activity – Internal messages show that Meta employees suggested removing metadata markers like ISBN numbers, copyright notices, and even fine-tuning their AI model to refuse reproducing book excerpts, indicating awareness of potential legal repercussions.
Fair use argument pushed to its limits – Meta and OpenAI argue that training AI on copyrighted content is transformative and thus fair use. However, the scale of unauthorized use, combined with internal discussions about avoiding legal consequences, weakens their argument.
Most Valuable Insights
Meta prioritized books over web data – Internal discussions stated that books were more valuable than web content for training high-quality AI, reinforcing the importance of professional writing in AI model development.
LibGen contains copyrighted works from major publishers and authors – The database includes works by well-known authors like Joan Didion, Sally Rooney, and even academic journal articles from Nature and The Lancet, highlighting the vulnerability of scholarly publishing.
Long-term implications for authors and researchers – The article argues that AI companies absorbing copyrighted works into their models without proper licensing threatens the sustainability of writing, journalism, and academic research.
Final Takeaway
This report provides damning evidence of major AI companies knowingly using pirated content to build their models. It raises serious legal and ethical concerns about copyright infringement, the limitations of fair use defenses, and the long-term impact on content creators.
Would you like to discuss how this might impact AI-related contract negotiations or legal frameworks?
Question 2 of 3 for ChatGPT-4o: Yes please.
ChatGPT-4o’s answer:
This article has significant implications for AI-related contract negotiations, legal frameworks, and the broader discussion on AI governance. Here are some key takeaways and strategic considerations:
1. Contract Negotiations: Strengthening Protections Against Unlicensed Use
Implication:
Meta and OpenAI’s reliance on pirated books confirms that AI companies prioritize comprehensive datasets, even at legal risk. This underscores the need for explicit contractual safeguards preventing unauthorized use of copyrighted material in AI training.
Strategic Considerations:
Stronger Contractual Clauses:
Require AI companies to disclose all datasets used in model training and provide mechanisms for auditing.
Demand indemnification clauses protecting rights holders from any unauthorized usage.
Introduce explicit prohibitions against the use of unauthorized, pirated, or legally ambiguous content.
Compensation & Licensing Models:
Given that AI companies seek high-quality text, publishers and authors could negotiate collective licensing agreements instead of allowing a chaotic legal battle.
Consider performance-based compensation for dataset usage (e.g., models trained with licensed content perform better).
Time-limited Licenses:
AI companies should not be granted indefinite rights to datasets. Instead, retraining obligations could be imposed, forcing models to remove content after a period.
2. Legal Frameworks: Pushing for Clearer AI Copyright Regulations
Implication:
The fair use defense has been central to AI companies' legal arguments, but the deliberate use of pirated content raises serious doubts about its legitimacy. This creates an opportunity to push for stronger regulations.
Strategic Considerations:
Legislative Push on AI Copyright Protection:
Advocate for narrowing the fair use defense when AI companies train models on copyrighted works at scale.
Push for AI training disclosures—similar to food labeling laws—forcing AI companies to reveal what data they ingest.
Litigation as a Regulatory Tool:
The lawsuits from Sarah Silverman and other authors signal a growing legal pushback. Large publishers and industry stakeholders could file collective lawsuits to force AI companies to negotiate proper licensing terms.
Courts may set precedents that weaken AI companies' fair use claims, benefiting content owners.
Governments Requiring AI Training Consent Mechanisms:
Opt-in instead of opt-out models should be the default for copyrighted material.
AI companies should be legally required to compensate rights holders—similar to how music streaming platforms pay royalties.
3. Long-Term Impact on AI Governance & Model Integrity
Implication:
If companies like Meta continue training AI on pirated content, this creates systemic risks in AI integrity, data provenance, and liability.
Strategic Considerations:
AI Model Integrity & Transparency:
Without knowing what AI models are trained on, there is no way to verify if their outputs respect copyrights or misinformation risks.
Publishers and researchers must demand verifiable transparency standardsfor AI training datasets.
Preventing Legal & Ethical Loopholes:
The article suggests that Meta employees deliberately masked their activity to obfuscate legal risks.
This raises the need for stronger compliance auditing—potentially through third-party AI ethics boardsthat verify dataset integrity.
AI Ethics & Policy Engagement:
The report reinforces that self-regulation is failing, meaning governments must step in.
Organizations like the U.S. Copyright Office, WIPO, and the EU AI Act could push for global AI training standards.
Next Steps: What Can Be Done Now?
Legal & Policy Advocacy:
Engage with copyright policymakers (e.g., U.S. Copyright Office, EU AI regulators) to shape the debate.
Use the Meta revelations as leverage in legislative discussions.
Stronger AI Licensing & Negotiations:
Negotiate mandatory attribution and monetization models for AI-trained content.
Demand indemnification from AI companies for copyright risks.
Litigation as a Deterrence Tool:
Collaborate with authors, publishers, and legal teams to pursue legal actionsthat set precedents against unauthorized dataset use.
Conclusion: AI Companies Are on the Defensive
Meta and OpenAI's reliance on pirated books reveals a systemic issue in AI development—one that can be challenged through stronger legal contracts, policy interventions, and litigation strategies.
Question 3 of 3 for ChatGPT-4o: What do the court documents mentioned in the article say? Are there any additional surprising, controversial and valuable statements made in those documents as well?
ChatGPT-4o’s answer:
Key Takeaways from the Court Documents
The court filing (Kadrey et al. v. Meta Platforms, Inc., Case No. 3:23-cv-03417-VC) provides damning evidence of Meta’s actions regarding the use of pirated books for training its Llama AI models. It expands on The Atlantic’s article by including internal communications, technical details, and legal arguments.
Here are the most surprising, controversial, and valuable statements found in the court documents:
Most Surprising Statements
Meta Knew Books Were Essential for AI Training
Internal discussions confirm that Meta employees identified books as the most valuable dataset for training LLMs. Books were considered more important than web data due to their structure, coherence, and writing quality.
Meta’s director of product for GenAI, Eugene Nho, testified that “not every word is equally insightful” and that books provide a unique advantage.
Meta Explored Licensing but Abandoned It Once Piracy Was an Option
In early 2023, Meta pursued deals with major publishers like HarperCollins, Simon & Schuster, and Penguin Random House.
Meta was prepared to spend millions on licensing, with a budget initially set at $___ million and later raised to $___ million.
But in April 2023, Meta executives—including Mark Zuckerberg—decided to stop licensing efforts entirely because they realized they could get the same books for free via LibGen.
Meta Downloaded and Trained on More than 267 Terabytes of Pirated Books
This dataset includes tens of millions of copyrighted works, making it equivalent to the entire Library of Congress 20 times over.
Meta Used Amazon Web Services (AWS) to Hide Its Activities
Instead of using Meta’s own servers, Meta routed its torrenting through AWS to avoid detection.
When an engineer asked why they weren’t using Facebook’s infrastructure, he was told, “to avoid risk of tracing back the seeder/downloader to FB servers.”
Most Controversial Statements
Meta Used BitTorrent for Large-Scale Piracy and Likely Re-Uploaded the Data
Downloading via BitTorrent typically involves re-uploading to other users (seeding)—which is explicitly illegal under copyright law.
Internal messages show Meta engineers were aware that torrenting was risky:
One wrote, “Torrenting from a corporate laptop doesn’t feel right.”
Another asked, “What is the probability of getting arrested for using torrents in the USA?”
Meta engineers discussed methods to avoid seeding files, but those safeguards were incomplete.
Meta Deliberately Covered Up the Use of Pirated Data
Meta employees stopped using the term “pirated” and instead referred to the data as ‘external’ or ‘publicly available.’
One employee warned, “It’s the piracy (and us knowing and being accomplices) that’s the issue.”
When The Atlantic revealed the existence of Books3 (a dataset Meta used that contained 200,000 pirated books), a Meta employee responded, “Ah yikes, Books3 contains more than I think the lawyers realize.”
Meta’s Own Engineers Raised Ethical Concerns
Some Meta engineers explicitly objected to the company’s actions:
One wrote: “I feel that using pirated material should be beyond our ethical threshold.”
Another engineer asked: “Is LibGen legal?” (and then posted a Google result confirming it was not).
Meta Believed that Paying for One Book Would Destroy Its Fair Use Defense
An internal message warned, “If we license one single book, we won’t be able to lean into the fair use strategy.”
This confirms Meta knew its fair use argument was weak and actively structured its strategy around avoiding licensing obligations.
Most Valuable Statements for Legal & Policy Discussions
Meta’s Fair Use Argument Is Unlikely to Hold Up in Court
Plaintiffs argue that Meta’s mass copying was purely commercial and not transformative.
Courts have ruled in prior cases (e.g., Napster, Google v. Authors Guild) that using pirated content to avoid paying for licenses does not qualify as fair use.
Piracy Directly Harms Authors and the Publishing Industry
The plaintiffs argue that Meta’s AI models directly reduced demand for books:
It deprived authors of licensing revenue.
It flooded the market with AI-generated summaries that compete with original works.
Meta’s AI Models Show Signs of Memorization (Verbatim Output from Books)
Experts confirmed Meta’s models can regurgitate copyrighted text word-for-word.
This strengthens claims that Meta’s AI models do not transform the original content but instead store and output it verbatim—a major risk for copyright enforcement.
Potential Next Steps for AI Copyright Governance
This case sets a precedent for future AI copyright lawsuits and suggests stronger legal and contractual protections are necessary.
For Legal and Contract Negotiations:
Require AI Companies to Disclose Training Data
Contracts should include mandatory dataset transparency provisions.
AI developers should prove that content is lawfully obtained.
Explicit Licensing & Compensation Mechanisms
Collective licensing models (like how music streaming platforms compensate artists) should be developed.
AI companies should pay a percentage of revenue based on dataset contributions.
Stronger Litigation Strategies
Class-action lawsuits could push AI companies into negotiated settlements.
Precedent-setting rulings could narrow the scope of fair use in AI training.
Regulatory Changes to Address AI Training & Copyright
AI companies should be legally required to obtain explicit permissionbefore using copyrighted content.
Fair use defenses for AI should be re-evaluated in legislative discussions.
Final Thoughts: Meta’s AI Development Strategy Is Built on Copyright Infringement
These court documents provide overwhelming evidence that Meta knowingly pirated books, covered up its actions, and misled the public about its use of copyrighted material. This could have severe legal, financial, and reputational consequences.
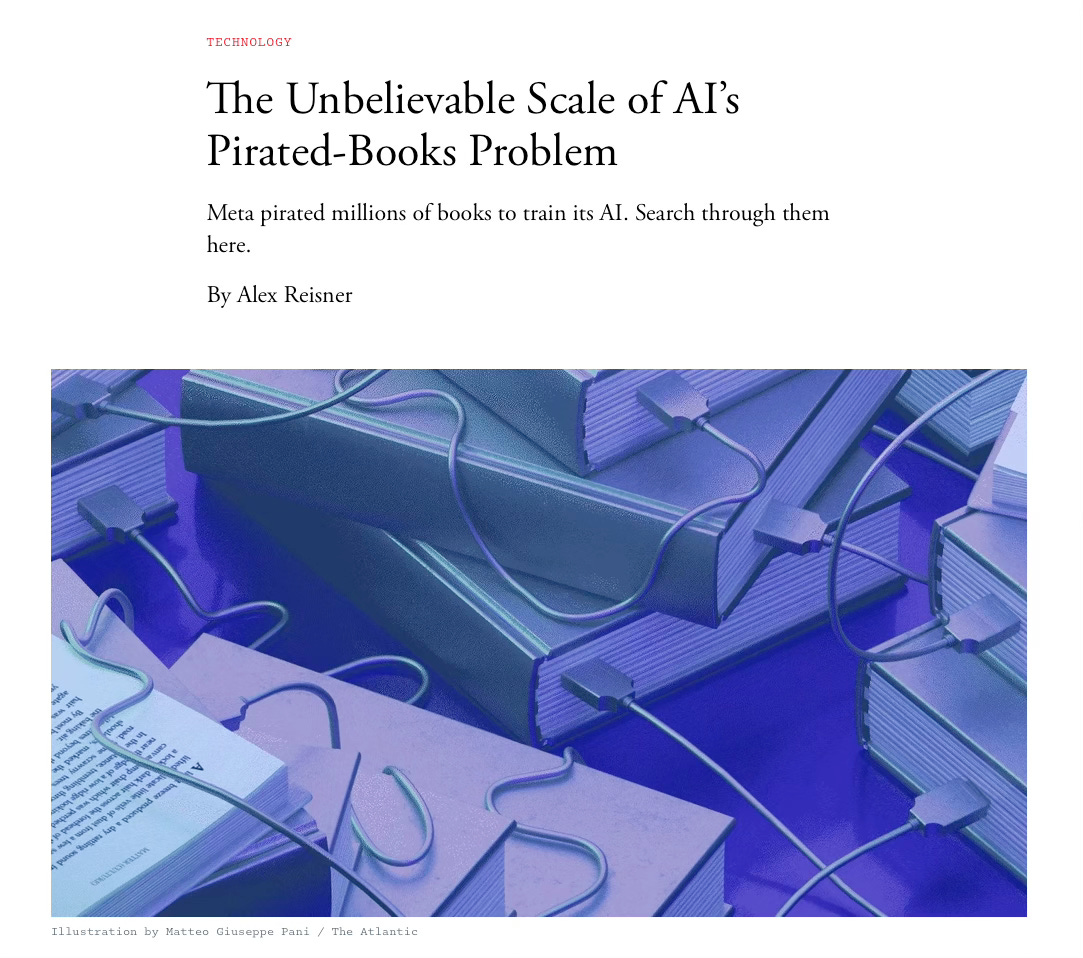