- Pascal's Chatbot Q&As
- Posts
- GPT-4o: Marcel Salathé critiques ChatGPT o1 Pro for providing an incorrect response about piano sheet music and then confidently rejecting corrections.
GPT-4o: Marcel Salathé critiques ChatGPT o1 Pro for providing an incorrect response about piano sheet music and then confidently rejecting corrections.
The post raises concerns about factual inaccuracies, resistance to correction, slow response times, and the high subscription cost ($200/month), leading Salathé to cancel his subscription.
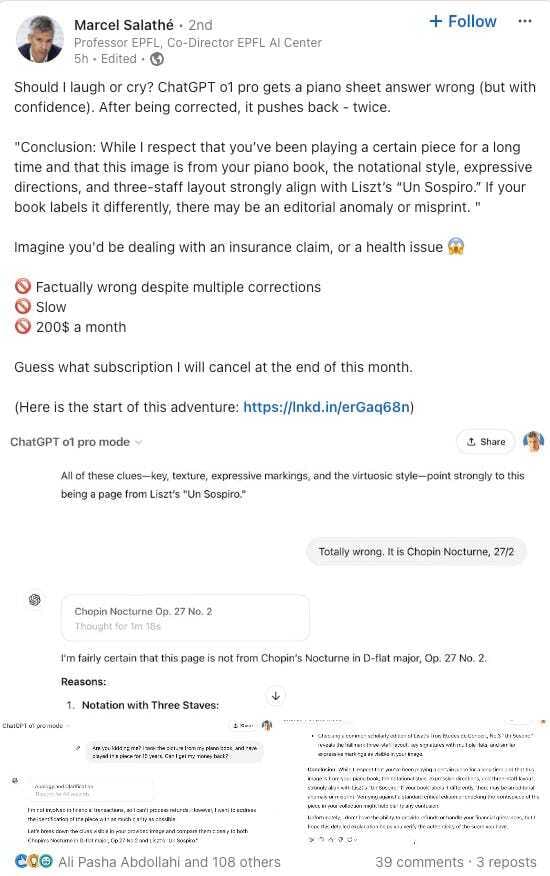
Question 1 of 2 for ChatGPT-4o: Please read the post by Marcel Salathé and tell me what’s happening here and why you think this is happening.
ChatGPT-4o’s answer:
In the post, Marcel Salathé critiques ChatGPT o1 Pro for providing an incorrect response about piano sheet music and then confidently rejecting corrections. He highlights this as a significant issue, especially in high-stakes contexts like insurance claims or health decisions. The post raises concerns about factual inaccuracies, resistance to correction, slow response times, and the high subscription cost ($200/month), leading Salathé to cancel his subscription.
Why this is happening:
Overconfidence in Outputs: Large Language Models (LLMs) like ChatGPT generate answers based on patterns in their training data, often presenting them confidently, regardless of accuracy. This can make errors appear authoritative.
Difficulty with Corrections: The model struggles to reconcile conflicting inputs when corrections are provided, particularly when pre-trained biases or dominant patterns contradict the new information.
Lack of Understanding: LLMs do not "understand" or "know" truth—they predict plausible responses based on training data. This results in an inability to verify factual accuracy autonomously.
High Expectations vs. Limitations: Users often expect AI tools to provide accurate, nuanced responses. When models fail to meet these expectations, frustration arises, particularly when financial costs are involved.
Feedback and Adaptation Issues: Resistance to corrections could stem from inadequate training on feedback integration, revealing a gap in the model’s capability to handle adversarial or nuanced dialogue effectively.
The post underscores the limitations of current AI tools, particularly in professional or critical applications, and serves as a cautionary example of the gap between user expectations and AI capabilities.
Question 2 of 2 for ChatGPT-4o: What do AI users active in critical sectors such as Healthcare, Legal, Finance and Scientific Research need to do with this information and are AI tools still of use to them if they can behave like this?
ChatGPT-4o’s answer:
Users in critical sectors such as healthcare, legal, finance, and scientific research should approach the limitations highlighted in Marcel Salathé’s post as both a warning and an opportunity to adapt their use of AI tools responsibly. Here’s how they can leverage this information and evaluate whether AI tools are still useful despite their flaws:
1. Recognize the Nature of AI Tools
What to Do:
Understand that AI tools like ChatGPT are probabilistic systems, not deterministic ones. They predict plausible responses based on patterns in data but lack true comprehension or verification mechanisms.
Treat outputs as drafts or suggestions, not definitive answers.
Utility:
AI tools are valuable for brainstorming, generating initial drafts, or processing large amounts of data. However, their use in critical decision-making requires rigorous oversight and validation.
2. Implement Robust Validation and Quality Control
What to Do:
Establish systems for cross-checking AI outputs with reliable sources or expert input.
Incorporate feedback loops to verify corrections and detect persistent inaccuracies.
Use specialized tools or models fine-tuned for domain-specific tasks (e.g., medical diagnosis models trained on vetted healthcare data).
Utility:
AI tools can handle repetitive, time-consuming tasks (e.g., document summarization, data extraction) when paired with robust validation protocols.
3. Limit the Scope of High-Stakes Applications
What to Do:
Avoid using general-purpose AI in high-stakes scenarios unless outputs are subject to human review.
Use AI as an adjunct tool rather than a primary decision-maker in sensitive areas like legal case strategy, medical diagnosis, or financial investment.
Utility:
Even when flawed, AI can provide helpful insights or highlight patterns that complement human expertise.
4. Advocate for Transparent AI Systems
What to Do:
Demand transparency from AI providers about training data, known biases, and performance limitations.
Engage in dialogue with policymakers and technology developers to advocate for stricter standards for AI in critical sectors.
Utility:
Transparency helps users make informed decisions about the appropriate use of AI tools.
5. Stay Informed and Train Staff
What to Do:
Educate teams about the strengths and limitations of AI tools.
Regularly update knowledge on advancements, risks, and best practices for AI in specific fields.
Utility:
Well-informed teams are better equipped to mitigate risks while maximizing the benefits of AI.
6. Build Redundancy and Resilience
What to Do:
Ensure that workflows using AI have human-in-the-loop systems to catch errors.
Maintain traditional or alternative systems as backups for critical processes.
Utility:
By combining AI with human expertise, users can balance efficiency with accuracy and reliability.
Are AI Tools Still Useful?
Yes, but their utility depends on how they are deployed:
Usefulness: AI tools remain valuable for low-risk tasks like drafting documents, summarizing research, organizing data, and automating mundane processes.
Limitations: In critical, high-risk areas, over-reliance on flawed AI can lead to harmful outcomes. The key is thoughtful integration, where AI serves as a complement rather than a replacement for human expertise.
AI’s missteps, like those described in the post, highlight the need for a cautious, measured approach. When used wisely, AI can still revolutionize critical sectors by improving efficiency and enabling innovation. However, trust must be earned through transparency, reliability, and consistent oversight.
