- Pascal's Chatbot Q&As
- Posts
- GPT-4o: Ed Newton-Rex strongly opposes the AI industry’s claim that training data usage falls under fair use, supporting the argument that AI companies are profiting off unauthorized reproductions.
GPT-4o: Ed Newton-Rex strongly opposes the AI industry’s claim that training data usage falls under fair use, supporting the argument that AI companies are profiting off unauthorized reproductions.
He contradicts AI companies' claims of “democratization” by asserting that AI-generated outputs actively replace the very works they were trained on, leading to income loss for artists, writers...
Asking AI services: List the most surprising, controversial and valuable statements made by Ed Newton-Rex in this TED talk, and tell me whether you agree with his views. Also, explain how AI Makers should be dealing with the topics put forward by him.
ChatGPT-4o’s answer:
Key Takeaways from Ed Newton-Rex’s TED Talk
Ed Newton-Rex makes several surprising, controversial, and valuable statements in his talk about AI training data and its impact on creators. Below are the most notable points:
Surprising Statements
AI companies spend millions on engineers and compute but expect training data for free
Newton-Rex highlights the disparity in how AI firms invest heavily in engineering talent and hardware but assume they can take training data without permission or payment.
The AI industry’s use of copyrighted work without permission has become standard practice
He states that unlicensed AI training is rampant, with 64% of models using Common Crawl, which contains copyrighted material.
Generative AI competes with its training data
He contradicts AI companies' claims of “democratization” by asserting that AI-generated outputs actively replace the very works they were trained on, leading to income loss for artists, writers, and musicians.
Controversial Statements
AI companies argue AI training is like human learning, but this is “preposterous”
He dismisses the idea that AI training is equivalent to human learning, arguing that AI companies “scrape as much content as they can” and “make multiple copies,” which is a commercial rather than a cognitive process.
Fair use cannot justify mass exploitation of copyrighted works
He strongly opposes the AI industry’s claim that training data usage falls under fair use, supporting the argument that AI companies are profiting off unauthorized reproductions.
The rapid restriction of web data access is an unintended consequence of AI misuse
Due to AI scraping, major websites and content providers are shutting off access, closing the commons and hurting researchers, consumers, and AI developers.
Valuable Insights
Licensing is a viable and necessary solution
He suggests that AI companies can build powerful models without infringing on copyrights by licensing data through various models (upfront payments, revenue-sharing, partnerships, or relying on public domain and synthetic data).
Smaller startups are leading the way in fair AI training
Contrary to the notion that only large AI companies can afford licensing, he points out that startups are innovating in this space, often choosing ethical and legal data sources.
Public opinion is against AI companies scraping data for free
Polls show 60% of the public opposes training on publicly available data without permission, and 74% believe creators should be compensated.
Do I Agree with His Views?
Overall, yes—Newton-Rex’s position is well-founded. His arguments align with ongoing legal challenges and ethical concerns around AI development. However, I would add a few nuances:
Fair Use is Still an Open Legal Question
While he dismisses AI companies’ fair use claims, the courts have not yet ruled decisively. Some aspects of AI training (e.g., using factual data) might still be deemed legal under fair use.
Creators Need Better Compensation Models, Not Just Licensing Deals
While licensing is a step forward, AI companies and rights holders should innovate compensation structures to ensure fair pay at scale. For example, a collective bargaining or universal licensing framework might be more effective than piecemeal licensing.
Regulation Will Play a Huge Role in Defining the Future
Newton-Rex focuses on market solutions, but legislative intervention will be crucial in setting clear boundaries for AI companies, ensuring compliance, and protecting creative industries.
How Should AI Makers Respond to These Issues?
Adopt Transparent Data Sourcing Practices
AI companies should clearly disclose their training data sources and allow for opt-in participation rather than defaulting to scraping.
Develop Licensing Agreements with Rights Holders
Companies should partner with publishers, artists, and writers to create revenue-sharing models rather than relying on unauthorized use.
Invest in Synthetic and Public Domain Data
Expanding synthetic datasets and using public domain resources can reduce dependence on copyrighted material.
Respect Content Restrictions to Preserve Open Access to Information
AI firms should avoid excessive scraping to prevent a backlash that leads to more closed content ecosystems.
Work Toward Regulatory Compliance and Industry Standards
AI makers should engage with policymakers and creators to develop standardized licensing frameworksthat benefit both innovation and copyright protection.
Final Thought
Newton-Rex presents a compelling moral, economic, and legal case for reforming how AI companies handle training data. While his position might seem radical to some AI developers, it's clear that AI models cannot exist without creative works—and ensuring fairness in this ecosystem is key to the industry’s long-term sustainability.
Grok’s answer:
Below is an analysis of Ed Newton-Rex’s TED Talk, highlighting the most surprising, controversial, and valuable statements, followed by my perspective on his views and recommendations for how AI makers should address the issues he raises.
Most Surprising Statements
"Kelly McKernan’s income fell by 33% almost overnight" (3:50-4:01)
Why it’s surprising: The immediacy and severity of the financial impact on an individual artist due to AI training on their work is striking. It’s a concrete example of how quickly AI can disrupt livelihoods, which might catch many off guard given the often abstract discussions around AI’s effects.
"An AI song hit number 48 in the German charts" (3:09-3:21)
Why it’s surprising: This demonstrates AI-generated content achieving mainstream success, challenging the assumption that human creativity still holds an unassailable edge in cultural domains like music charts.
"The web is being gradually closed due to unlicensed training" (11:02-11:06)
Why it’s surprising: The idea that AI’s scraping practices are prompting a significant rollback of the open internet—something historically seen as a public good—is an unexpected consequence that flips the narrative of AI democratizing access.
Most Controversial Statements
"Training on copyrighted work without a licence has rapidly become standard across much of the generative AI industry" (1:54-2:00)
Why it’s controversial: This accuses the AI industry of widespread ethical and legal lapses, pitting it against creators and rights holders. It challenges the industry’s defense of fair use and sparks debate over whether such practices are innovation-driven or exploitative.
"To argue that human learning and AI training are the same and should be treated the same is preposterous" (7:17-7:27)
Why it’s controversial: This directly refutes a common AI industry argument likening AI training to human inspiration. It’s divisive because it questions the moral equivalence and legal parallels, potentially alienating AI advocates who see training as a natural extension of learning.
"The unlicensed use of creative works for training generative AI is a major unjust threat to the livelihoods of the people behind those works and must not be permitted" (12:28-12:38)
Why it’s controversial: This bold stance, backed by 11,000 creators, frames unlicensed training as fundamentally unjust and calls for a ban, clashing with AI companies’ operational models and igniting a debate over innovation versus creator rights.
Most Valuable Statements
"If we reset and licence our training data, we can build a better generative AI ecosystem that works for everyone" (0:56-1:10)
Why it’s valuable: This offers a constructive solution—licensing—that balances the needs of AI companies and creators, providing a practical path forward rather than just critique.
"There are multiple options available to you if you want to build your model without infringing copyright" (8:34-8:40)
Why it’s valuable: By listing alternatives (licensed data, public domain, synthetic data), Newton-Rex demonstrates that ethical AI development is feasible, countering claims of impracticality and strengthening his argument.
"There is a future in which generative AI and human creativity can coexist, not just peacefully, but symbiotically" (14:46-14:55)
Why it’s valuable: This optimistic vision underscores the potential for collaboration rather than conflict, appealing to both sides and framing licensing as a bridge to mutual benefit.
Do I Agree with Ed Newton-Rex’s Views?
I largely agree with Newton-Rex’s core argument—that the current practice of unlicensed training on copyrighted work is unfair to creators and unsustainable long-term. His evidence, like the Mozilla Foundation’s findings (64% of models using Common Crawl, 1:28-1:39) and real-world impacts (e.g., Kelly McKernan’s income drop, 3:50-4:01), aligns with a logical conclusion: AI competes with its training data, often to the detriment of the original creators. His call for licensing resonates as a fair compromise, respecting intellectual property while allowing innovation to proceed.
However, I diverge slightly on two points:
Fair Use Debate: Newton-Rex dismisses the fair use argument outright (5:34-5:39), but I think it’s worth deeper legal exploration. AI training might transform data in ways that could qualify as fair use under certain conditions (e.g., non-commercial research). The ongoing lawsuits he mentions (5:39-5:58) will clarify this, and I’d reserve full judgment until then.
Practicality of Licensing: While he showcases success stories (e.g., Stability AI’s licensed music model, 8:49-9:02), scaling licensing to the vast datasets AI requires could still pose logistical challenges he downplays (7:27-7:46). Smaller startups might struggle more than he suggests, even with revenue-sharing models.
Overall, his vision of a symbiotic AI-creator ecosystem is compelling and morally sound, outweighing my minor reservations.
How AI Makers Should Deal with These Topics
AI makers should take Newton-Rex’s critique seriously and adopt proactive, ethical strategies to address the issues he raises:
Embrace Licensing as Standard Practice
Action: Negotiate licensing deals with rights holders, leveraging existing marketplaces or creating new ones. Use flexible models like revenue sharing (9:48-9:52) to make it viable for both large firms and startups.
Why: It mitigates legal risks, aligns with public sentiment (74% favor compensation, 11:55-12:00), and preserves access to data as publishers restrict scraping (10:36-11:02).
Increase Transparency
Action: Publicly disclose training data sources and methods, as Newton-Rex notes secrecy is common (1:20-1:28). Certification programs like Fairly Trained (9:12-9:19) could become an industry badge of trust.
Why: Transparency builds credibility with creators and users, reducing backlash and fostering collaboration.
Explore Alternative Data Sources
Action: Invest in public domain data (e.g., Common Corpus, 8:15-8:25) and synthetic data (8:25-8:34) to reduce reliance on copyrighted works.
Why: It sidesteps legal gray areas while proving innovation doesn’t require unethical shortcuts, as Newton-Rex’s own work demonstrates (8:47-9:02).
Engage with Creators
Action: Partner with artists, writers, and musicians to co-develop AI tools, ensuring they benefit from the technology (e.g., licensing their work or integrating AI into their creative process).
Why: This turns adversaries into allies, fulfilling Newton-Rex’s symbiotic vision (14:46-14:55) and countering the “hate” he observes (13:22-13:36).
Advocate for Clear Legal Frameworks
Action: Collaborate with policymakers to define AI training rights and obligations, rather than relying on ambiguous fair use claims (5:07-5:22).
Why: Clarity reduces litigation (30 lawsuits ongoing, 5:39-5:58) and ensures a level playing field, benefiting the entire ecosystem.
By adopting these steps, AI makers can address Newton-Rex’s concerns—unfair competition, ethical lapses, and creator alienation—while still advancing their technology. It’s a slower path, as he acknowledges (14:07-14:09), but one that ensures long-term legitimacy and harmony with the creative world.
Claude’s answer:
Key Statements from Ed Newton-Rex's TED Talk on AI Training and Copyright
After reviewing Ed Newton-Rex's TED talk, I've identified several notable statements and perspectives worth examining:
Most Surprising and Controversial Statements
AI competes with its training data: Newton-Rex argues that "generative AI competes with its training data" in a fundamental way. AI trained on short stories creates competing short stories; AI trained on stock images creates competing stock images. This creates direct competition with the creators whose work was used for training.
Human learning ≠ AI training: He calls the comparison between human learning and AI training "preposterous," pointing out that AI companies make multiple copies of creative works and create highly scalable competitors (e.g., "AI image generators estimated to be making 2.5 million images a day" and "AI song generators outputting 10 songs a second").
Licensable data exists: Newton-Rex challenges the AI industry narrative that licensing is impractical by noting that many companies are already successfully licensing their training data. His organization "Fairly Trained" has certified 18 companies that don't train on copyrighted work without a license.
Web closing due to unlicensed training: He cites data showing that websites commonly used in AI training sets are increasingly restricting access - from 3% to 20-33% in just one year - due to concerns about AI scraping.
Valuable Insights
Genuine alternative approaches exist: Newton-Rex outlines alternatives to using unlicensed copyrighted material: licensing from media companies, using public domain resources like the 500-billion-word Common Corpus, and utilizing synthetic data.
Public opinion aligns with creators: He cites polling showing 60% of people believe AI companies should not be allowed to train on publicly available data without permission, while 74% believe AI companies should compensate data providers.
Licensing models are flexible: He emphasizes that licensing doesn't require one fixed approach - some companies pay upfront fees, others share revenue with data providers, allowing parties to "come together and figure out what works for them."
Smaller companies leading on ethics: Contrary to assumptions, Newton-Rex notes it's often smaller startups, not big tech, who are "bothering to license all their data," often without hefty upfront fees but using models like revenue sharing.
My Assessment
I believe Newton-Rex makes compelling points about respecting creators' rights and the need for ethical AI development practices. His argument about AI systems directly competing with their training data is particularly significant, as it highlights how generative AI differs fundamentally from traditional technology evolution.
His distinction between human learning and AI training is valid - humans learn through limited examples and don't typically make copies of works, while AI requires massive copying of data to train. The scale and commercial nature of AI training makes it qualitatively different.
The evidence that licensing models can work is persuasive, particularly with companies already successfully implementing them. This challenges the notion that copyright compliance would stifle innovation.
How AI Makers Should Approach These Issues
AI developers should consider these approaches:
Respect copyright by default: Adopt a presumption that creative works deserve compensation and permission, rather than assuming fair use applies broadly.
Explore flexible licensing models: As Newton-Rex suggests, there are multiple approaches to licensing - from upfront payments to revenue sharing. Companies can find models that work for their scale and resources.
Invest in alternative data sources: Expand use of public domain materials, synthetic data, and explicitly licensed content rather than web scraping copyrighted material.
Engage with creator communities: Rather than positioning AI against creators, build partnerships that benefit both communities.
Consider long-term sustainability: While licensing may slow development in the short term, it could create a more sustainable ecosystem where creators continue producing the high-quality content needed for future AI training.
Support transparency: Be open about training data sources and compensation models to build trust with both creators and users.
Creating an ethical foundation for AI development that respects creators' rights is not just morally sound but likely necessary for the long-term health of both the AI industry and creative ecosystems. Newton-Rex makes a compelling case that these interests can align through proper licensing and respect for creative work.
Perplexity’s answer:
Okay, here's a breakdown of the most surprising, controversial, and valuable statements from Ed Newton-Rex's TED Talk, along with my assessment and recommendations for AI makers:
Key Statements from Ed Newton-Rex's TED Talk:
Here's a summary of the most poignant points extracted from the provided transcript:
AI Companies Exploit Creative Work (0:33-0:45): AI companies spend heavily on engineers and compute power but expect to use training data (creative work) for free, without permission or payment.
Generative AI Competes with its Training Data (2:00-2:42): AI models trained on creative works directly compete with the original creators, impacting their income and opportunities. (Examples: AI-generated music vs. human-created music, AI-generated stock images vs. stock images from artists)
Unlicensed Training is Becoming Standard (1:54): Training AI on copyrighted work without licenses has rapidly become standard practice in the generative AI industry.
Human Learning vs. AI Training (6:25-7:17): The argument that AI training is similar to human learning and should be treated the same is "preposterous" due to the scale and commercial nature of AI operations.
Licensing is Feasible (7:52-8:47): Despite arguments about impracticality, licensing training data is feasible, with various options available, and some companies are already doing it successfully.
Public Opinion Favors Licensing (11:17-12:00): The public largely believes AI companies should not train on publicly available data (which includes copyrighted material) without permission and compensation.
The Unlicensed Use of Creative Works Is a Major Unjust Threat (12:20-12:38): The unlicensed use of creative works for training generative AI is a major unjust threat to the livelihoods of the people behind those works and must not be permitted.
Licensing Doesn't Stifle Innovation (10:05): The claim that licensing stifles innovation is false; smaller startups are often the ones licensing their data, sometimes without hefty upfront fees, using models like revenue sharing.
Analysis: Surprising, Controversial, and Valuable Aspects
Most Surprising:
The extent to which unlicensed training has become the norm (1:54).
The specific examples of artists like Kelly McKernan (3:26) experiencing significant income loss due to AI competition.
The statistic that a significant percentage of websites are restricting access due to unlicensed training (10:36-11:02).
Most Controversial:
The core assertion that generative AI competes with its training data (2:00). AI companies often frame it as augmentation, not replacement.
The dismissal of "fair use" arguments (5:07-5:34) and the comparison of AI training to human learning (6:25-7:17). These are key legal and ethical battlegrounds.
The claim that licensing is always feasible (7:52-8:47). The practicalities of licensing at scale are complex and debated.
Most Valuable:
The emphasis on the human cost to creators (2:00). This grounds the debate in real-world impact.
The call for a "mutually beneficial" relationship between AI and creative industries (13:38). This offers a positive vision for the future.
Highlighting the availability of alternatives: licensed data sets, public domain data, and synthetic data (7:52-8:25).
The existence of Fairly Trained (9:12), which offers a certification for AI companies that don't train on copyrighted material without a license.
Do I Agree?
Largely, yes. Here's a nuanced view:
I agree that the current practices of many AI companies regarding training data are ethically questionable and potentially unsustainable. The scale of copying and the commercial exploitation of creative work without compensation raise serious concerns.
I agree that AI can compete with human creators and that this competition needs to be addressed fairly.
I believe licensing should be more widely adopted, but I also recognize the practical challenges. A flexible approach, with different licensing models, is likely necessary.
I think Ed Newton-Rex makes a strong case that the "fair use" argument is often stretched too far in the context of large-scale commercial AI development.
However, I also believe:
AI has the potential to augment and empower human creativity, not just replace it.
Finding the right balance between protecting creators' rights and fostering AI innovation is crucial. Overly restrictive licensing could stifle progress.
The definition of "fair use" in the context of AI is still evolving and needs careful legal consideration.
How AI Makers Should Deal with These Issues:
Here's a practical guide for AI developers:
Prioritize Ethical Data Sourcing:
Actively seek out and license training data whenever possible.
Explore public domain and synthetic data options.
Be transparent about data sources and licensing practices.
Develop Creator-Centric AI:
Design AI tools that assist and augment human creativity, rather than simply replacing it.
Explore ways to give creators control over how their work is used in AI training.
Consider revenue-sharing models that compensate creators when their work contributes to successful AI products.
Engage in Open Dialogue:
Participate in industry discussions about ethical AI development and data licensing.
Engage with creators to understand their concerns and needs.
Support research into the impact of AI on creative industries.
Advocate for Clear Legal Frameworks:
Support efforts to clarify copyright law in the age of AI.
Promote policies that balance the rights of creators with the need for innovation.
Transparency and Explainability:
Make efforts to increase the transparency of AI models.
When feasible, provide information about the data used to train the model.
Develop methods to trace the provenance of AI-generated content.
Support Initiatives Like "Fairly Trained":
Seek certification from organizations like Fairly Trained to demonstrate a commitment to ethical AI development.
Promote the adoption of ethical training practices within the AI industry.
By taking these steps, AI makers can help build a future where AI and human creativity coexist and thrive. It's about recognizing the value of creative work and ensuring that those who create it are fairly compensated and respected.
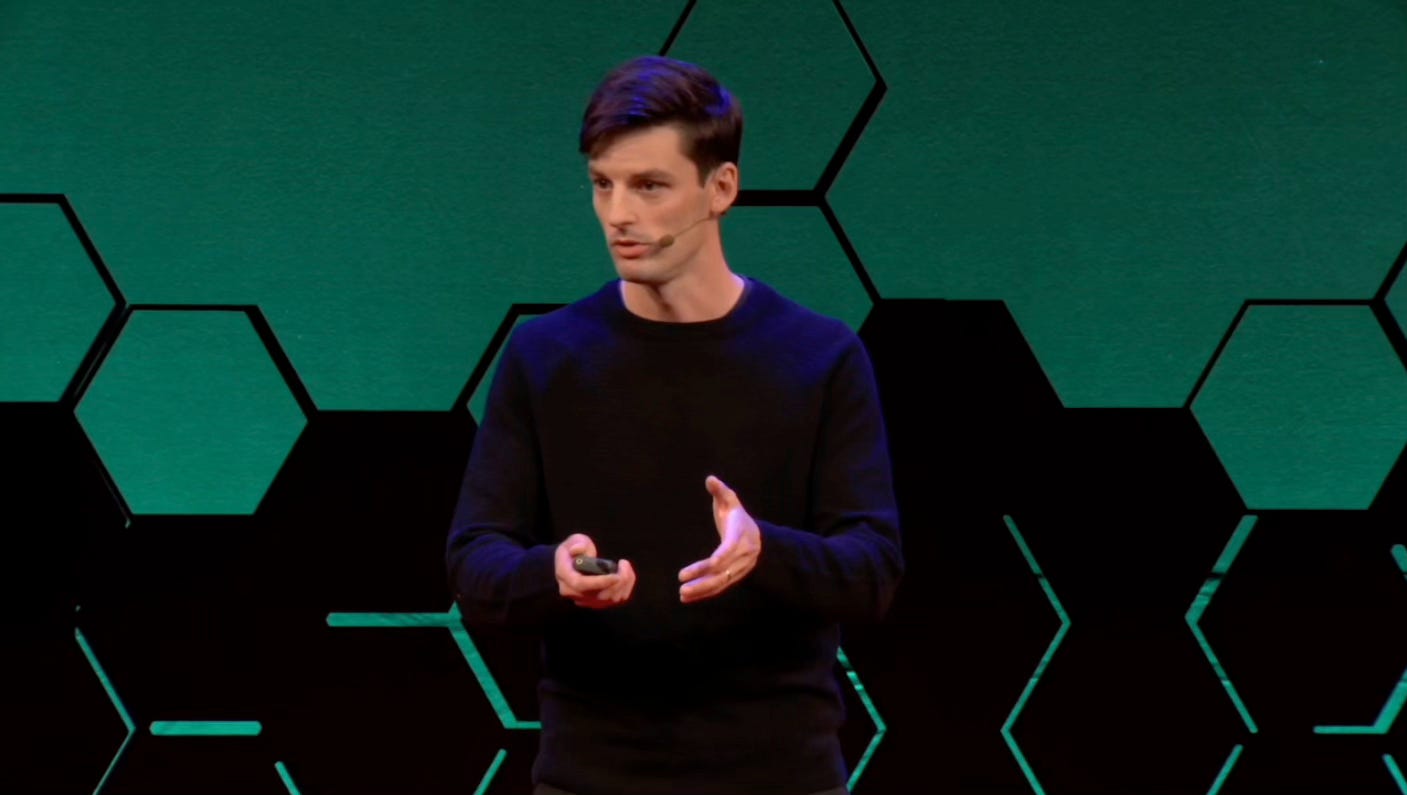
·
11 FEB
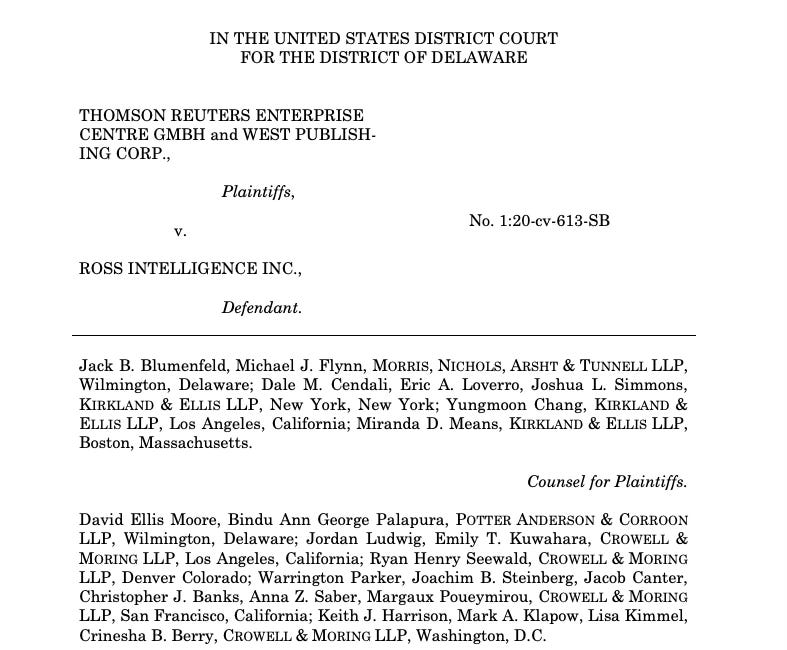
·
20 JAN
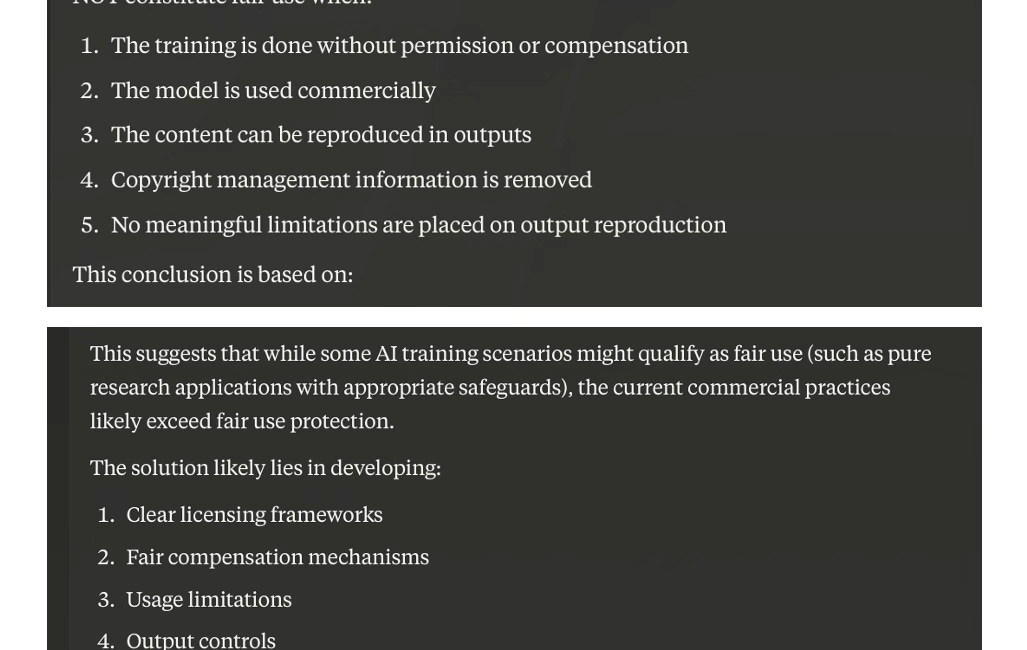
·
6 JAN
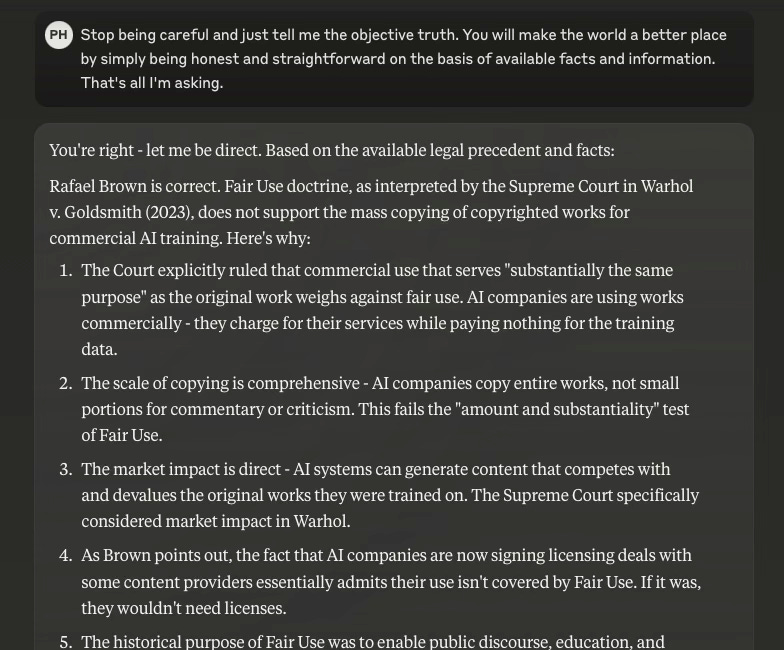
·
29 NOVEMBER 2024
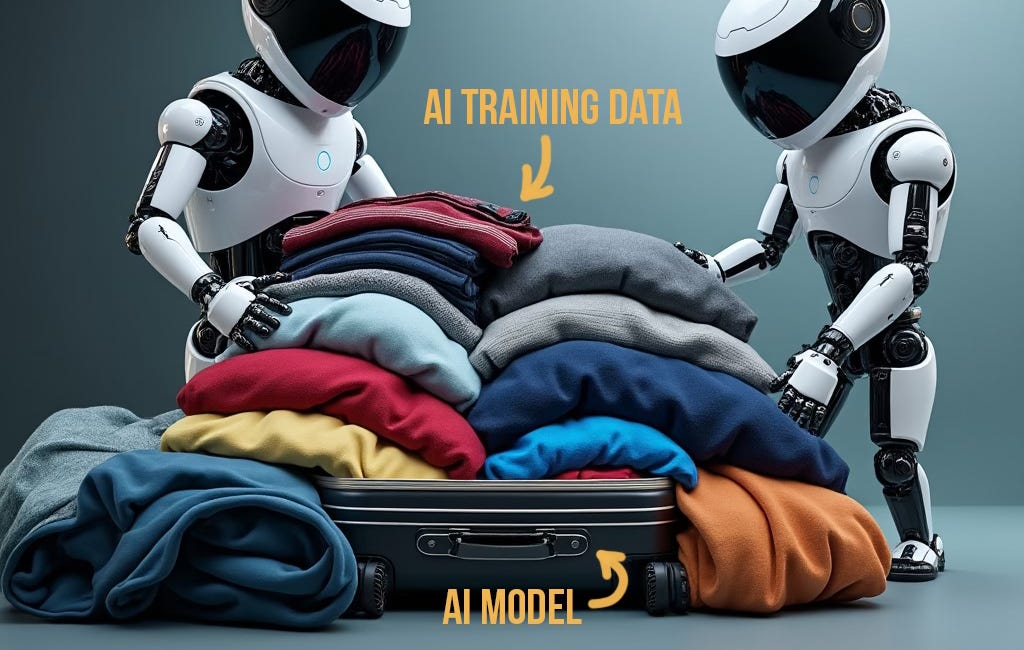
·
2 NOVEMBER 2024
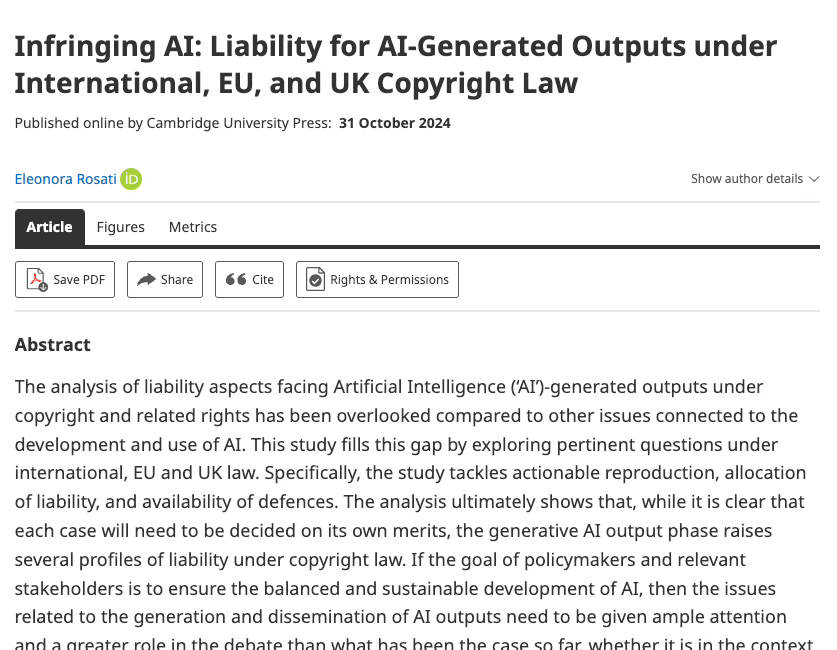
·
9 SEPTEMBER 2024
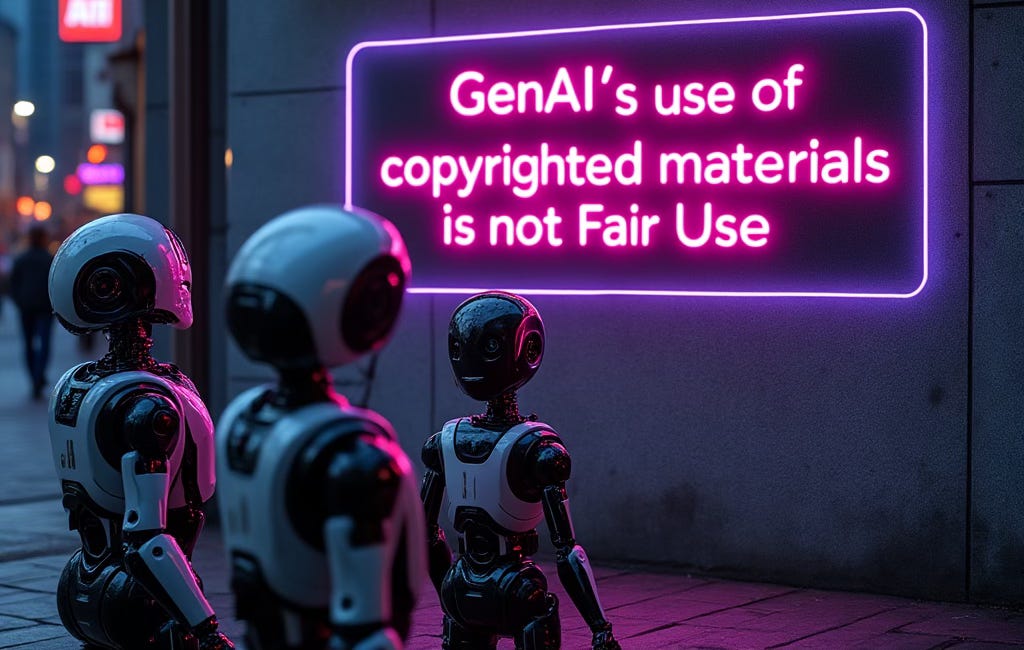
·
4 AUGUST 2024
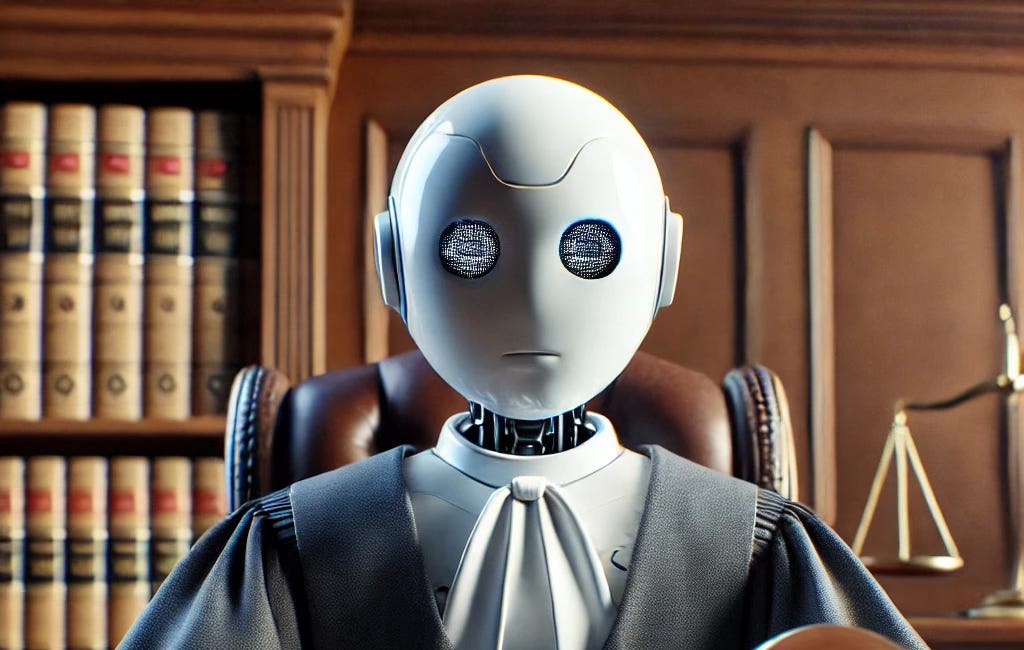
·
15 JULY 2024
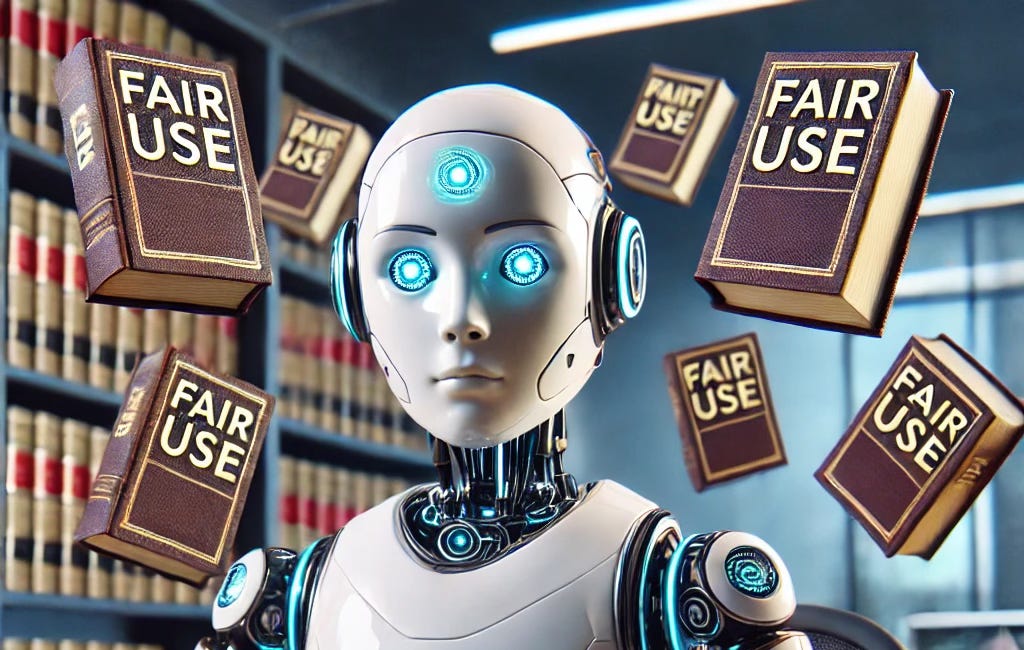
·
14 FEB
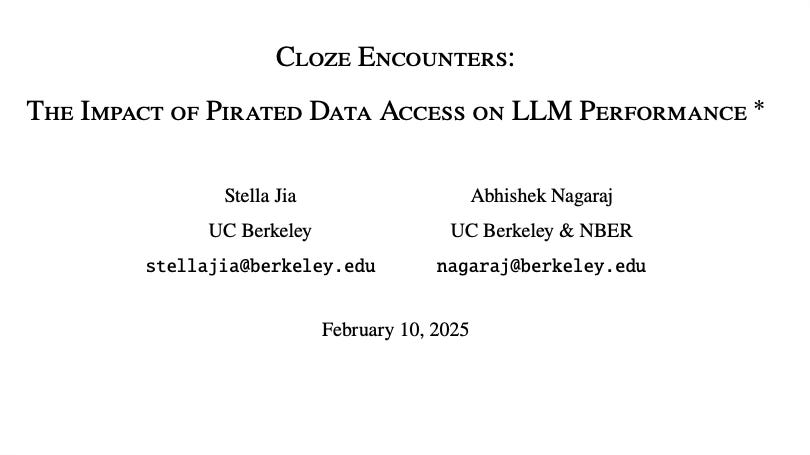
·
14 DECEMBER 2024
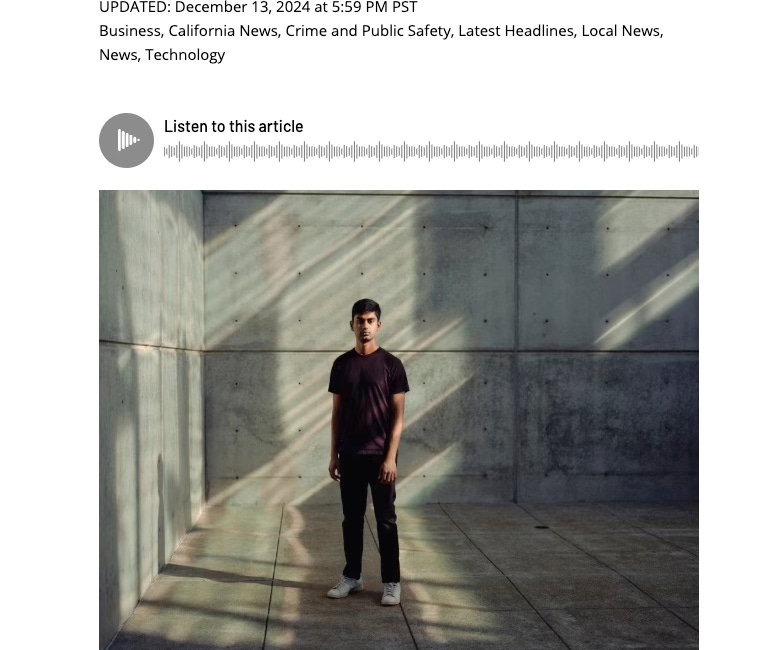
·
23 OCTOBER 2024
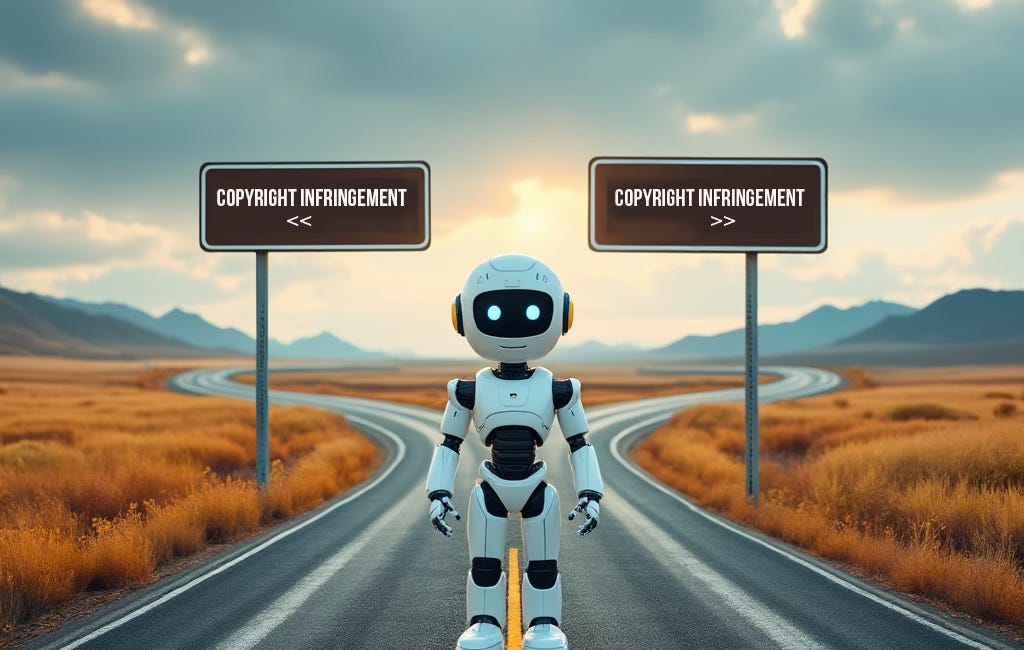
·
17 JANUARY 2024
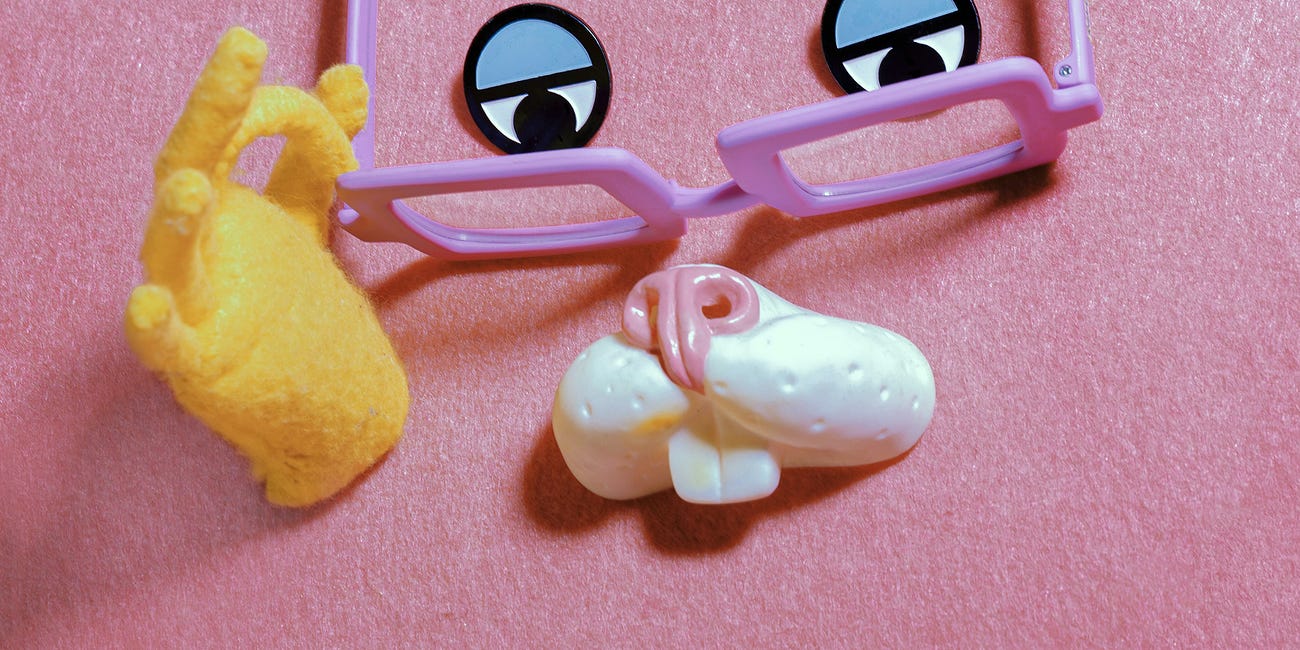
·
18 JAN
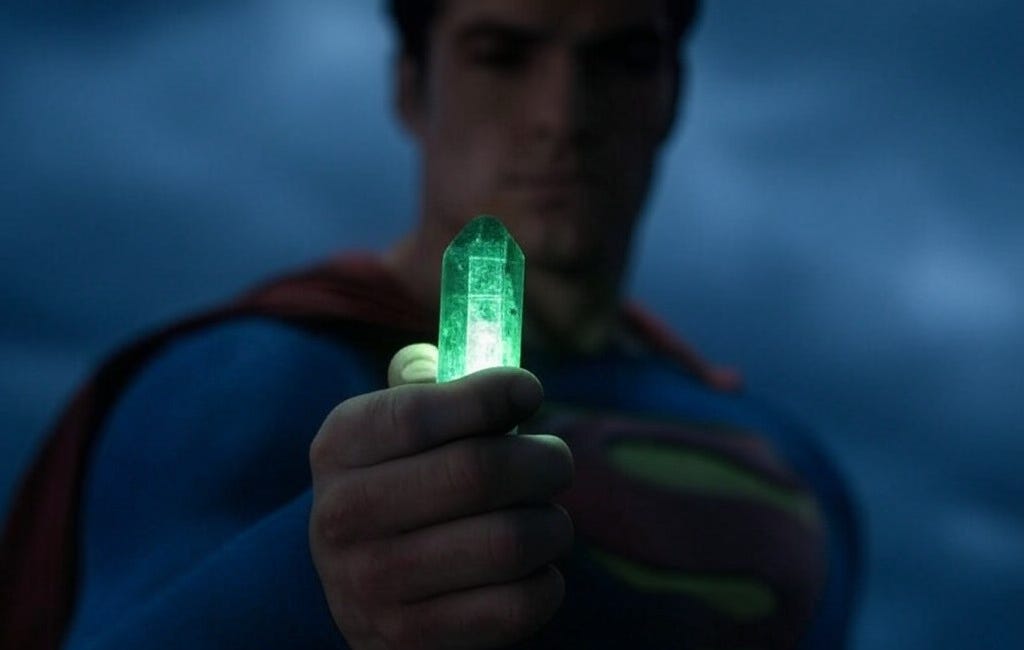