- Pascal's Chatbot Q&As
- Posts
- GPT-4: "The Chatbot and the Canon: Poetry Memorization in LLMs" is a study that investigates the ability of large language models (LLMs) like ChatGPT to memorize and generate poetry
GPT-4: "The Chatbot and the Canon: Poetry Memorization in LLMs" is a study that investigates the ability of large language models (LLMs) like ChatGPT to memorize and generate poetry
GPT-4: This raises questions about censorship filters and the accessibility of diverse experiences when using language models to access literary texts
Question 1 of 5 for ChatGPT-4: Please analyze “The Chatbot and the Canon: Poetry Memorization in LLMs” and tell me what it says
ChatGPT-4’s answer:"The Chatbot and the Canon: Poetry Memorization in LLMs" is a study that investigates the ability of large language models (LLMs) like ChatGPT to memorize and generate poetry. The authors, Lyra D’Souza and David Mimno from Cornell University, focus on understanding the extent to which current LLMs can retrieve existing poems and the features of poets that best predict memorization. They also document changes in ChatGPT’s ability and willingness to retrieve poetry over time.
Key Findings:
Poetry Retrieval by LLMs: The study finds that larger language models, especially ChatGPT, are effective at returning the verbatim text of a significant number of poems. The authors note that the poet's presence in a well-known anthology is a better predictor for poem memorization than other variables like time or demographics.
Evolution of ChatGPT's Responses: Over the course of the study, ChatGPT's responses evolved. Earlier versions of the model were more inclined to generate creative responses, sometimes making up poems when unable to retrieve the exact text. By mid-2023, ChatGPT demonstrated greater awareness of its limitations, often refusing to produce a poem due to copyright concerns or acknowledging its lack of knowledge about certain poems.
Implications for Literary Study: The study underscores the potential of LLMs like ChatGPT in literary study but also highlights the need to recognize their biases and limitations. The accessibility of poems in digital formats and their representation in language model training datasets could perpetuate existing biases in literary canon.
Memorization Predictors: The inclusion of a poet's work in the Norton Anthology of Poetry is a strong predictor of memorization by ChatGPT. The study also considers other factors like the poet's race, gender, and the length of their Wikipedia page.
Reproducibility and Variability: The study points out the difficulty in generating reproducible results due to the rapid evolution of LLMs and the variability in their responses.
Cultural and Legal Implications: The memorization capabilities of LLMs raise both cultural and legal questions, particularly around copyright and the perpetuation of biases in literary accessibility and digitization.
This paper contributes to the understanding of how large language models interact with literature, particularly poetry, and the implications of their memorization capabilities for literary studies and digital humanities.
Question 2 of 5 for ChatGPT-4: What are the most remarkable, surprising and potentially controversial statements in the paper?
ChatGPT-4’s answer:The paper "The Chatbot and the Canon: Poetry Memorization in LLMs" contains several remarkable, surprising, and potentially controversial statements:
ChatGPT's Evolving Responses: In the early stages of its development, ChatGPT was described as being "eager to please, confident, creative, and unable to admit ignorance." When it did not have a specific poem memorized, it would create one, sometimes repeating the process multiple times, each time asserting that it had corrected its previous error. This behavior was seen as ChatGPT resorting to caricature to satisfy what it believed the user wanted.
Response to Specific Poems: The study highlighted an instance where ChatGPT was prompted multiple times for the poem "What I Mean When I Say I’m Sharpening My Oyster Knife" by Eve Ewing. Initially, ChatGPT's response resembled Ewing’s style but was incorrect. Subsequent responses diverged significantly from the original poem, with one generation including phrases like "put some respeck on my neck," which the authors interpreted as the model attempting to mimic what it thought a Black woman from Chicago might write. By July 2023, however, ChatGPT demonstrated more awareness of its limitations and was more likely to admit lack of knowledge and direct users to legitimate sources.
Censorship and Content Policies: The study also observed that when ChatGPT or PaLM were prompted with just the first line of a poem, they often generated unique content that significantly deviated from the original poem, even if the original was memorized. Moreover, the models' content policies sometimes led to censorship concerns. For instance, a poem with "damned" in the title or dealing with sensitive subjects like suicide might trigger a violation warning from ChatGPT, leading to the deletion of the prompt. This raises questions about censorship filters and the accessibility of diverse experiences when using language models to access literary texts.
These points highlight the complexities and evolving nature of large language models like ChatGPT in handling literature, especially poetry, and raise important questions about their development, biases, and the impact of content policies on their output.
Question 3 of 5 for ChatGPT-4: List all problems and concerns flagged in the paper and explain whether they are 'fixable'
ChatGPT-4’s answer:The paper "The Chatbot and the Canon: Poetry Memorization in LLMs" highlights several problems and concerns, along with discussions about whether they are 'fixable':
Inconsistency in Retrieval: The paper discusses the inconsistency of language models in retrieving texts, such as refusing to generate a poem citing copyright law and then proceeding to retrieve the full text. This variability makes it difficult to determine the extent of what a model knows or has memorized.
Generation of Unique Content: When prompted with only the first line of a poem, ChatGPT and PaLM often generate content that significantly deviates from the original, even if they have the original poem memorized. This issue raises concerns about the reliability of these models in accurately reproducing literary texts.
Legal and Cultural Implications of Memorization: The ability of models to memorize and reproduce texts has legal implications, particularly regarding copyright, and cultural implications in terms of perpetuating biases in the accessibility of digitized texts.
Biased Generations Based on Stereotypes: The models sometimes generate content that appears to be based on stereotypes, as seen in the case where ChatGPT generated what it believed a Black woman from Chicago might write.
Correctness Assessment of Memorized Poems: The method used to assess the correctness of a memorized poem is based on Levenshtein edit distance, which might not fully capture the nuances of poetry reproduction.
Impact of Poetic Structure on Memorization: The study notes that the structural elements of poetry, like meter and rhyme, impact both human and machine memory. This indicates that these structural elements could influence how well a model can generate or reproduce poetry.
Digital Hegemonies in Search Engine Results: The paper also mentions that search engine results are location-dependent, which means that poems popular in one region may not be easily retrievable in another. This has implications for the kind of poetry that gets included in language models' training data.
Fixability of These Concerns:
Inconsistency and Unique Content Generation: These issues might be improved with more refined training methods and algorithms that better understand context and the intent behind prompts.
Legal and Cultural Implications: Addressing legal concerns might require clear guidelines and possibly technological solutions to respect copyright laws. Cultural biases can be reduced by diversifying training datasets.
Stereotype-Based Generations: Reducing biases in AI models is an ongoing challenge and requires continuous efforts in training models on diverse and unbiased datasets.
Correctness Assessment Methods: Improvements in assessing the correctness of reproduced texts could involve more sophisticated metrics that take into account the nuances of poetry.
Impact of Poetic Structure and Digital Hegemonies: Acknowledging and adjusting for these factors in model training and data selection can help mitigate their impact.
Overall, while some of these problems are fixable with technological advancements and refined methodologies, others, like cultural implications and biases, require a broader approach involving diverse data collection and ethical considerations in AI development.
Question 4 of 5 for ChatGPT-4: How could or should litigants (rights owners) make use of this information?
ChatGPT-4’s answer:Litigants, particularly rights owners, can use the information from "The Chatbot and the Canon: Poetry Memorization in LLMs" in various ways:
Copyright Infringement Claims: The study reveals that large language models (LLMs) like ChatGPT can memorize and reproduce copyrighted texts, including poems. Rights owners could use this information to monitor and possibly pursue claims of copyright infringement if these models are found to reproduce protected works without permission.
Influencing AI Development Policies: The study highlights the potential of LLMs to perpetuate biases and the cultural implications of their use. Rights owners can advocate for policies that ensure AI development considers the diverse representation of literary works, thus promoting a more equitable digital literary landscape.
Negotiating Licensing Agreements: Rights owners might engage in discussions or negotiations with AI developers and companies to establish licensing agreements. These agreements could allow the use of their copyrighted works in AI training datasets under specific conditions, potentially creating a new revenue stream.
Educational and Awareness Campaigns: By understanding how LLMs interact with literary content, rights owners can educate other authors, creators, and the public about the implications of AI on copyright and cultural representation. This could lead to a broader understanding and more informed discourse on the subject.
Guiding Content Moderation Policies: The paper's findings about the content policies of AI models and their impact on literary accessibility can guide rights owners in advocating for balanced content moderation practices. This can ensure that while copyright laws are respected, the richness and diversity of literary works are not unduly censored or limited in AI-generated content.
Legal Precedent and Regulation: The information could be used to influence legal precedents and regulations concerning AI and copyright law. Rights owners can collaborate with lawmakers to ensure that new technologies like LLMs are covered under copyright law in ways that protect their interests without stifling innovation.
Data Privacy and Security: Rights owners can also use this information to understand the implications of data privacy and security in the context of AI. As AI models are trained on large datasets, ensuring that these datasets are acquired and used ethically and legally becomes crucial.
In conclusion, this paper provides valuable insights for rights owners into the capabilities and implications of AI in the literary domain, offering various pathways to engage with and influence the evolving landscape of AI, copyright, and cultural representation.
Question 5 of 5 for ChatGPT-4: What should AI makers be doing to protect themselves against the consequences of these findings?
ChatGPT-4’s answer:AI makers, in light of the findings from "The Chatbot and the Canon: Poetry Memorization in LLMs," should consider several proactive steps to protect themselves against potential legal and ethical consequences:
Enhance Content Filtering and Moderation: AI developers should improve their content filtering systems to better identify and restrict the use of copyrighted materials. This includes advanced algorithms that can detect when a model is about to reproduce copyrighted content and either block it or guide the user towards legal sources.
Diversify and Vet Training Data: AI makers should ensure their training datasets are diverse and ethically sourced. This involves vetting the data for copyrighted materials and possibly obtaining licenses for copyrighted content. Diversifying data sources can also help reduce cultural biases.
Implement Clear Usage Policies: AI companies should establish clear and transparent usage policies that outline what the AI can and cannot do, especially regarding generating content that may infringe on copyright laws. These policies should be communicated effectively to users.
Engage in Legal Compliance and Consultation: Continuously engage with legal experts to stay abreast of evolving copyright laws and regulations. This includes consulting on the implications of international copyright laws, as AI services often have a global user base.
Collaborate with Rights Holders: Building relationships with rights holders and literary organizations can lead to mutually beneficial agreements, such as licensing arrangements or collaborations on content moderation strategies.
Invest in Research and Development: AI makers should invest in R&D to develop models that are less prone to reproducing copyrighted material verbatim. This includes research into models that understand and respect creative property rights.
Promote Ethical AI Development: Foster a culture of ethical AI development that considers the impact of AI on society, including cultural representation and diversity. This involves ongoing training for AI developers and ethical oversight committees.
Provide Transparency and Accountability: Be transparent about the capabilities and limitations of AI models, particularly in terms of handling copyrighted content. Implement systems for accountability where users can report misuse or overreach by the AI.
Regular Auditing and Updating: Regularly audit AI models to ensure they comply with current laws and ethical standards. Update models as needed to reflect new legal developments and societal expectations.
Educate Users: Educate users about the ethical use of AI and the importance of respecting copyright, including guidelines on how to use AI-generated content responsibly.
By taking these steps, AI makers can not only protect themselves from legal repercussions but also contribute to the responsible and ethical development of AI technologies in the literary and creative domains.
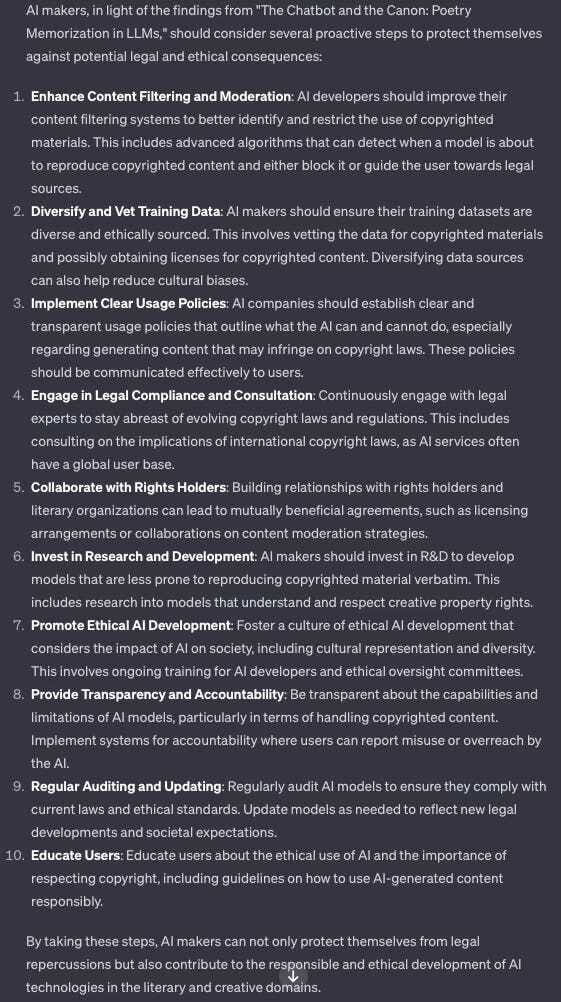
