- Pascal's Chatbot Q&As
- Posts
- GPT-4: The AI tax approach relies on approximating the value extracted from copyrighted works, which might not accurately reflect the true value or impact of individual works on AI model performance
GPT-4: The AI tax approach relies on approximating the value extracted from copyrighted works, which might not accurately reflect the true value or impact of individual works on AI model performance
GPT-4: Disparities in how contributions are valued and compensated. Gemini: Creators might not see direct benefits. Claude: AI tax is a more workable approach. Copilot: Careful planning required.
Asking AI services: Please read the articles “Licensing Fees For AI Training Data Is Impractical—AI Tax Is Workable” and “OpenAI Attorneys Say Licensing All Training Data Is Impossible”. Do you agree with these views? Explain the pros and cons of the suggested approaches and provide evidence for your views whenever possible.
ChatGPT-4’s answer:The discussion around the use of copyrighted material in AI training and the proposal of an AI tax as a workable solution brings several pros and cons to the forefront.
Pros of the AI Tax Approach:
Practicality: Licensing every piece of copyrighted content used in AI training individually is logistically impossible due to the sheer volume of data needed for training large language models. An AI tax offers a feasible alternative by imposing a tax based on the model's parameter size, a proxy for the value extracted from the copyrighted works through learning .
Compensation for Society: The revenue from the AI tax could be directed towards a compensation fund, benefiting cultural, educational, and technological initiatives. This ensures that society at large, if not the individual creators themselves, receives compensation for the use of their works .
Encourages Social Responsibility: Implementing an AI tax aligns with the broader principle of internalizing externalities. AI companies would be compelled to consider the broader impact of their technologies, balancing innovation with social responsibility .
Cons of the AI Tax Approach:
Complexity in Implementation: The most equitable and effective method for imposing an AI tax will likely require trial and error, necessitating careful planning and consensus among stakeholder groups. This complexity might slow down the process and make initial implementations less effective .
Potential Impact on Innovation: While the tax aims to ensure companies contribute back to society, there is a concern that it might also stifle innovation in the AI sector if the tax burden is perceived as too high or if the tax structure is not carefully designed to encourage continued development and use of AI technologies .
Difficulty in Valuing Contributions: The AI tax approach relies on approximating the value extracted from copyrighted works, which might not accurately reflect the true value or impact of individual works on AI model performance. This approximation might lead to disparities in how contributions are valued and compensated .
Evidence and Further Considerations:
The articles "Licensing Fees For AI Training Data Is Impractical—AI Tax Is Workable" and "OpenAI Attorneys Say Licensing All Training Data Is Impossible" outline the challenges and propose the AI tax as a solution. However, the implementation of such a tax would require careful consideration of various factors, including how to assess the value contributed by copyrighted materials accurately, ensuring that the tax does not discourage innovation, and developing a transparent and equitable system for distributing the funds collected through the tax .
In conclusion, while the AI tax approach presents a novel solution to the impracticality of licensing each piece of copyrighted content used in AI training, it also introduces challenges that need to be addressed. The success of this approach will depend on the ability to create a system that is both equitable and conducive to continued innovation in the AI sector.
Google Gemini’s answer:AI Tax vs. Licensing Fees: Pros and Cons
The articles argue that traditional licensing fees for training data used in large language models (LLMs) are impractical and proposes an AI tax as a solution. Here's a breakdown of the pros and cons of each approach:
Licensing Fees
Cons:
Impracticality: As the articles point out, negotiating individual licenses for the massive amount of data used to train LLMs is nearly impossible.
Uncertain Copyright Scope: Copyright law might not apply neatly to AI training data. The "use" is to learn patterns, not necessarily copy the material.
Pros:
Fairness: Authors and creators would be directly compensated for their work used in training LLMs.
Specificity: Compensation would be tied to the specific works used, potentially rewarding popular content.
AI Tax
Pros:
Scalability: An AI tax could be levied based on model size or data usage, streamlining the process.
Societal Benefit: Tax revenue could be directed to cultural and educational initiatives that benefit creators indirectly.
Internalizing Externalities: AI companies would account for the impact of their technology on society.
Cons:
Indirect Compensation: Individual creators might not see direct benefits.
Tax Design Challenges: Determining the tax base (data size, revenue) and ensuring progressivity (taxing larger companies more) requires careful planning.
Price Shifting: Companies might try to pass the tax burden onto consumers, potentially affecting affordability.
My View
An AI tax seems like a more viable approach compared to individual licensing for LLM training data. However, the design of the tax is crucial. Here are some considerations:
Transparency: The allocation of tax revenue should be clear and involve creators.
Balance: The tax should encourage innovation but also compensate society for the use of data.
Price Controls: Measures might be needed to prevent companies from simply raising prices to offset the tax.
Overall, the ideal solution would likely involve a combination of approaches. Further discussion and collaboration among stakeholders (creators, AI developers, policymakers) are necessary to create a fair and effective system.
Claude’s answer: Based on the articles, I agree that licensing fees for AI training data is not a practical solution, and an AI tax is a more workable approach. Here are the key pros and cons of the two approaches:
Pros of an AI tax:
It provides a scalable and streamlined way to compensate for the use of copyrighted material in training AI models, which would be nearly impossible to do through individual licensing agreements.
The revenue generated from the tax could be used to fund cultural, educational, and technological initiatives that benefit creators, past and present.
It aligns with the principle of internalizing the externalities created by AI technologies, forcing companies to account for the broader social impact.
Cons of an AI tax:
Determining the appropriate tax base (e.g., model parameters, revenue) and rate structure (e.g., tiered system) may require trial-and-error to get right.
There is a risk of the tax being passed on to consumers, making AI-powered products and services less affordable.
Ensuring the transparent and equitable allocation of the tax revenue to various stakeholder groups may be challenging.
Pros of licensing fees:
It would provide direct compensation to individual copyright holders whose works are used in training AI models.
It would uphold traditional intellectual property rights and principles.
Cons of licensing fees:
As stated by the OpenAI attorneys, obtaining consent and negotiating licenses for the enormous volume of data used to train large language models would be practically impossible.
The value of the copyrighted works to the AI models may not align with their market value as works of art, making it difficult to determine appropriate licensing fees.
The articles present a compelling case that an AI tax is a more practical and scalable solution than individually negotiated licensing fees. The AI tax approach recognizes the limitations of traditional IP frameworks in dealing with the unique challenges posed by large language models, while still aiming to compensate society and the creative community for the use of their works. However, the implementation details of such a tax will require careful consideration to ensure it is equitable and effective.
MS Copilot’s answer:The article “Licensing Fees For AI Training Data Is Impractical—AI Tax Is Workable” by Andrew Leahey discusses the impracticality of negotiating individual licensing agreements for each protected work used in training large language models like OpenAI’s ChatGPT. The author suggests that an AI tax could be a viable solution to ensure that society, if not individual authors, is compensated for the use of copyrighted works in AI training data.
Pros of AI Tax:
Scalability: An AI tax could be applied uniformly, avoiding the complexity of individual licensing agreements.
Societal Benefit: Revenue from the tax could fund cultural, educational, and technological initiatives, benefiting creators and society.
Internalizing Externalities: It would force AI companies to account for the broader impact of their technologies.
Cons of AI Tax:
Valuation Challenges: Determining the tax base could be complex, whether it’s based on the model’s parameters or the revenue generated by AI applications.
Potential for Cost Shifting: There’s a risk that AI companies might pass the tax cost to consumers.
Trial and Error: Finding the most equitable and effective method for imposing an AI tax will likely require adjustments over time.
The second article, “OpenAI Attorneys Say Licensing All Training Data Is Impossible” by Isaiah Poritz, echoes the sentiment that obtaining consent for all copyrighted content used in AI training is unfeasible due to the sheer volume of data.
Both articles highlight the challenges of applying traditional intellectual property laws to AI and suggest that new approaches, like an AI tax, may be necessary to balance innovation with social responsibility and compensation for the use of copyrighted materials. The evidence presented is largely based on the logistical and financial impracticality of individual licensing and the potential benefits of a more streamlined solution like an AI tax. However, the implementation of such a tax would require careful planning and consensus among various stakeholders to ensure its effectiveness and fairness.
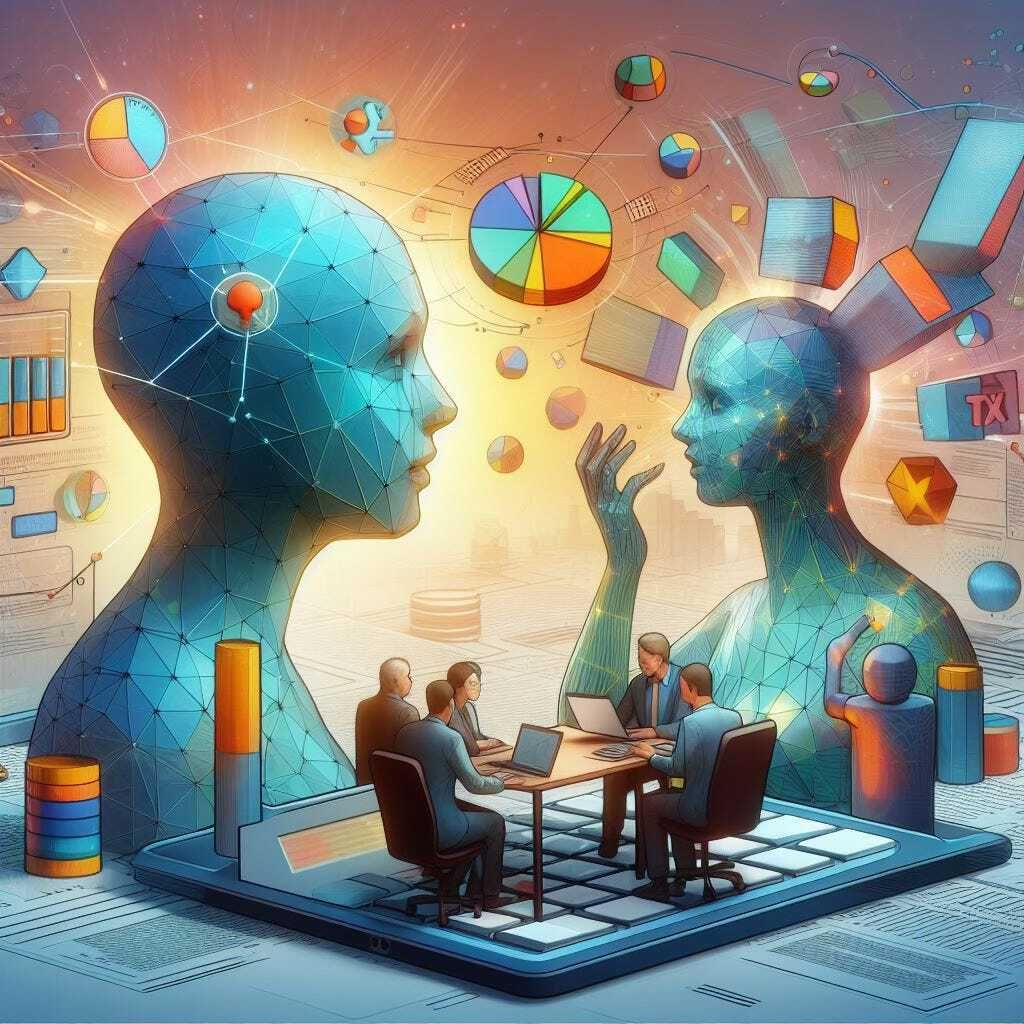