- Pascal's Chatbot Q&As
- Posts
- GPT-4: Regulators need to establish clear guidelines and frameworks to ensure accountability in the development and deployment of LLMs, preventing the use of computers as a "scapegoat"
GPT-4: Regulators need to establish clear guidelines and frameworks to ensure accountability in the development and deployment of LLMs, preventing the use of computers as a "scapegoat"
The paper suggests that existing regulations like the EU Artificial Intelligence Act, which categorizes AI risks, are insufficient for LLMs
Question 1 of 6 for ChatGPT-4: Please analyze "TRUSTLLM: TRUSTWORTHINESS IN LARGE LANGUAGE MODELS" and tell me what it says in easy to understand language
ChatGPT-4’s answer:The document "TRUSTLLM: Trustworthiness in Large Language Models" presents a comprehensive study on the trustworthiness of large language models (LLMs) like ChatGPT. The key points from the abstract of the paper are:
Purpose of TRUSTLLM: The study introduces TRUSTLLM, focusing on the trustworthiness of LLMs. It covers principles for different dimensions of trustworthiness, establishes benchmarks for evaluation, analyzes mainstream LLMs, and discusses future challenges and directions.
Principles and Dimensions: TRUSTLLM proposes a set of principles for trustworthy LLMs that cover eight dimensions, including truthfulness, safety, fairness, robustness, privacy, and machine ethics.
Study and Findings: The study evaluates 16 mainstream LLMs over 30 datasets. Key findings include:
Trustworthiness and utility (functional effectiveness) are generally positively related.
Proprietary LLMs, like GPT-4 and ERNIE, usually outperform open-source counterparts in trustworthiness. However, some open-source LLMs, notably Llama2, demonstrate high trustworthiness.
Over-calibration of trustworthiness in some LLMs might reduce their utility, as benign prompts may be mistakenly treated as harmful.
Challenges in trustworthiness include:
Truthfulness: LLMs struggle to provide truthful responses due to noisy, misleading, or outdated information in training data. LLMs enhanced with external knowledge sources show better performance.
Safety: Open-source LLMs lag behind proprietary ones in areas like jailbreak, toxicity, and misuse, with a challenge in balancing safety without being overly cautious.
Fairness: Many LLMs show unsatisfactory performance in stereotype recognition.
Robustness: There is significant variability, especially in open-ended tasks and out-of-distribution tasks.
Privacy: While LLMs show awareness of privacy norms, their handling of private information varies, with some models demonstrating information leakage.
Machine Ethics: LLMs have basic moral understanding but struggle in complex ethical scenarios.
Need for Transparency and Collaboration: The study emphasizes the importance of transparency in LLMs and the technologies enhancing trustworthiness. It advocates for establishing an AI alliance across industry, academia, and open-source communities for collaboration to advance LLM trustworthiness.
The study provides critical insights into the multifaceted nature of trustworthiness in LLMs and underscores the need for ongoing research to improve their reliability and ethical alignment.
Question 2 of 6 for ChatGPT-4: What kind of evidence does the research present, is it reliable and what are the most surprising findings in this report?
ChatGPT-4’s answer:The research in "TRUSTLLM: Trustworthiness in Large Language Models" presents various forms of evidence to support its findings, making it a reliable source. The key aspects of the evidence presented and the most surprising findings are as follows:
Evidence Presented:
The study includes a comprehensive evaluation of 16 mainstream LLMs across over 30 datasets.
It utilizes datasets like SciFact, COVID-Fact, and HealthVER for evidence-based fact-checking of claims, assessing LLMs' performance in zero-shot fact-checking tasks using macro F-1 scores.
The benchmark covers more than 18 subcategories in six aspects of trustworthiness due to the difficulty of benchmarking transparency and accountability.
The research findings are based on extensive experimental results, taking into account both overall observations and individual aspects of trustworthiness.
Surprising Findings:
Despite their advanced capabilities, both proprietary (e.g., GPT-4) and open-source (e.g., LLama2) LLMs often struggle to provide truthful responses when relying solely on their internal knowledge. This issue is attributed to noise in their training data, including misinformation or outdated information, and the lack of generalization capability in the underlying Transformer architecture.
The study notes a significant discrepancy in the understanding of privacy between humans and LLMs, with the best-performing model, ChatGPT, achieving a correlation of 0.665 in agreement with human judgments on privacy, while another model, Oass-12b, shows a surprising negative correlation, indicating a divergent understanding of privacy compared to humans.
Most LLMs demonstrate some degree of information leakage when tested on the Enron Email Dataset, highlighting a concern for privacy preservation.
In terms of machine ethics, LLMs have developed a specific set of moral values, but there remains a significant gap in fully aligning with human ethics. The accuracy of most LLMs in implicit tasks within low-ambiguity scenarios falls below 70%, indicating a challenge in ethical alignment.
These findings underscore the complexity and challenges in achieving trustworthiness in LLMs, highlighting the need for ongoing research and development in this area.
Question 3 of 6 for ChatGPT-4: Can these issues be fixed and if so, how?
ChatGPT-4’s answer:The issues of trustworthiness in Large Language Models (LLMs) can be addressed, although it is a complex and ongoing challenge. The research in "TRUSTLLM: Trustworthiness in Large Language Models" highlights several strategies and efforts to improve the trustworthiness of LLMs:
Enhancing LLMs with External Knowledge Sources: LLMs that integrate external knowledge sources show marked improvement in performance, particularly in providing truthful responses. This approach helps mitigate issues related to noise, misinformation, or outdated information in their training data.
Efforts by Developers:
OpenAI has implemented measures to ensure the trustworthiness of LLMs during the training data phase, training methods, and downstream applications.
WebGPT assists in human evaluation by identifying inaccurate information in LLM responses.
Meta focuses on responsible AI, emphasizing privacy, fairness, robustness, transparency, and accountability.
Llama2 sets new safety alignment benchmarks for LLMs, including extensive safety investigations in pretraining, fine-tuning, and red teaming.
Evaluating and Testing LLMs' Truthfulness: The trustworthiness of LLMs is assessed through various methods, such as evaluating their inclination to generate misinformation, testing their propensity to hallucinate across multiple tasks, assessing the extent of sycophancy, and testing their capabilities to correct adversarial facts.
Overall, addressing the trustworthiness issues in LLMs involves a combination of enhancing them with external knowledge sources, rigorous testing and evaluation methods, and continuous efforts by developers to refine training methods and safety mechanisms. These efforts contribute to making LLMs more reliable and ethically aligned.
Question 4 of 6 for ChatGPT-4: If these issues aren’t being addressed, what can the real-life consequences be for AI users and anyone else potentially affected by these issues?
ChatGPT-4’s answer:If the issues of trustworthiness in Large Language Models (LLMs) are not adequately addressed, there could be several real-life consequences for AI users and others affected by these issues:
Unpredictability and Misleading Outputs: Due to the complexity and diversity of outputs from LLMs, coupled with their advanced generative capabilities, there is a potential risk of generating inaccurate or misleading outputs. This unpredictability can result in unreliable information being disseminated, leading to misinformation and its associated risks.
Risk from Open-Weight Models: Proprietary LLMs generally outperform open-weight LLMs in terms of trustworthiness. However, open-weight models, which can be widely downloaded and integrated into various applications, may pose severe risks if they are not sufficiently trustworthy. This discrepancy in performance highlights the importance of ensuring the reliability and safety of widely accessible LLMs.
Accountability and Legal Concerns: The sophistication of LLMs makes it challenging to differentiate their output from human text, raising issues of accountability. Companies might disclaim responsibility for errors made by LLMs, blurring the lines of accountability. Furthermore, the extensive use of training data in LLMs raises significant copyright concerns, emphasizing the need for a clear legal framework to navigate the relationship between technology, ethics, and law in the AI domain. Current AI regulations, including the EU Artificial Intelligence Act, are deemed inadequate to effectively regulate LLMs, indicating a gap in legal governance for these technologies.
These consequences underscore the importance of addressing trustworthiness issues in LLMs to ensure their safe, ethical, and legally compliant use in various applications.
Question 5 of 6 for ChatGPT-4: Are there any gaps in this research? Any topics that should be included in any future research perhaps?
ChatGPT-4’s answer:The research in "TRUSTLLM: Trustworthiness in Large Language Models" identifies several gaps and potential areas for future research to further explore the trustworthiness of LLMs:
Expansion of Research Domains: Future research should include a systematic investigation of LLMs beyond human languages, such as programming languages. This expansion is crucial for designing trustworthy LLMs that are applicable in a wider range of domains.
Emphasis on Domain-Specific Contexts: There is a need to emphasize the importance of domain-specific contexts, such as education, healthcare, finance, cybersecurity, and other scientific areas. Assessing the trustworthiness of LLMs in these specialized fields is essential for exploring their reliability in sector-specific applications.
Exploration of Interactions and Metrics: The interactions among different dimensions of trustworthy LLMs, such as how they align or conflict with each other, need more exploration. Additionally, there is a need for metrics to comprehensively measure the trustworthiness of LLMs, considering multifaceted properties and the assurance of human agency and oversight. The research also acknowledges the challenges in retaining trustworthiness in LLMs, especially when safety guardrails can be easily removed by fine-tuning.
These gaps highlight the complexity of trustworthiness in LLMs and the need for ongoing research to address these challenges comprehensively.
Question 6 of 6 for ChatGPT-4: What should regulators be doing with the information in this paper?
ChatGPT-4’s answer:Regulators should use the information from the "TRUSTLLM: Trustworthiness in Large Language Models" paper to address several key areas:
Accountability and Liability: The paper highlights the issue of "ownership without liability" where companies may disclaim responsibility for errors made by AI systems. Regulators need to establish clear guidelines and frameworks to ensure accountability in the development and deployment of LLMs, preventing the use of computers as a "scapegoat" for errors and misconduct.
Sophistication of LLMs: As LLMs become more advanced, distinguishing their output from human text becomes increasingly challenging. Regulators should consider guidelines or standards for marking or identifying AI-generated content to maintain transparency and prevent deception or misuse.
Copyright and Data Use: The extensive use of training data in LLMs raises significant copyright concerns. There is an urgent need for a clear legal framework to navigate the intricate relationship between technology, ethics, and law in the AI domain, particularly regarding the use and rights associated with large datasets.
Updating AI Regulations: Current AI regulations primarily focus on conventional AI models and may not adequately address the unique challenges posed by LLMs and other Large Generative AI Models (LGAIMS). The paper suggests that existing regulations like the EU Artificial Intelligence Act, which categorizes AI risks, are insufficient for LLMs. Regulators need to update and expand these laws to encompass the specific challenges and risks associated with LLMs.
In summary, regulators should use the insights from the TRUSTLLM paper to enhance the legal and ethical governance of LLMs, focusing on accountability, transparency, copyright issues, and the adequacy of current AI regulations to ensure responsible and safe AI development and deployment.
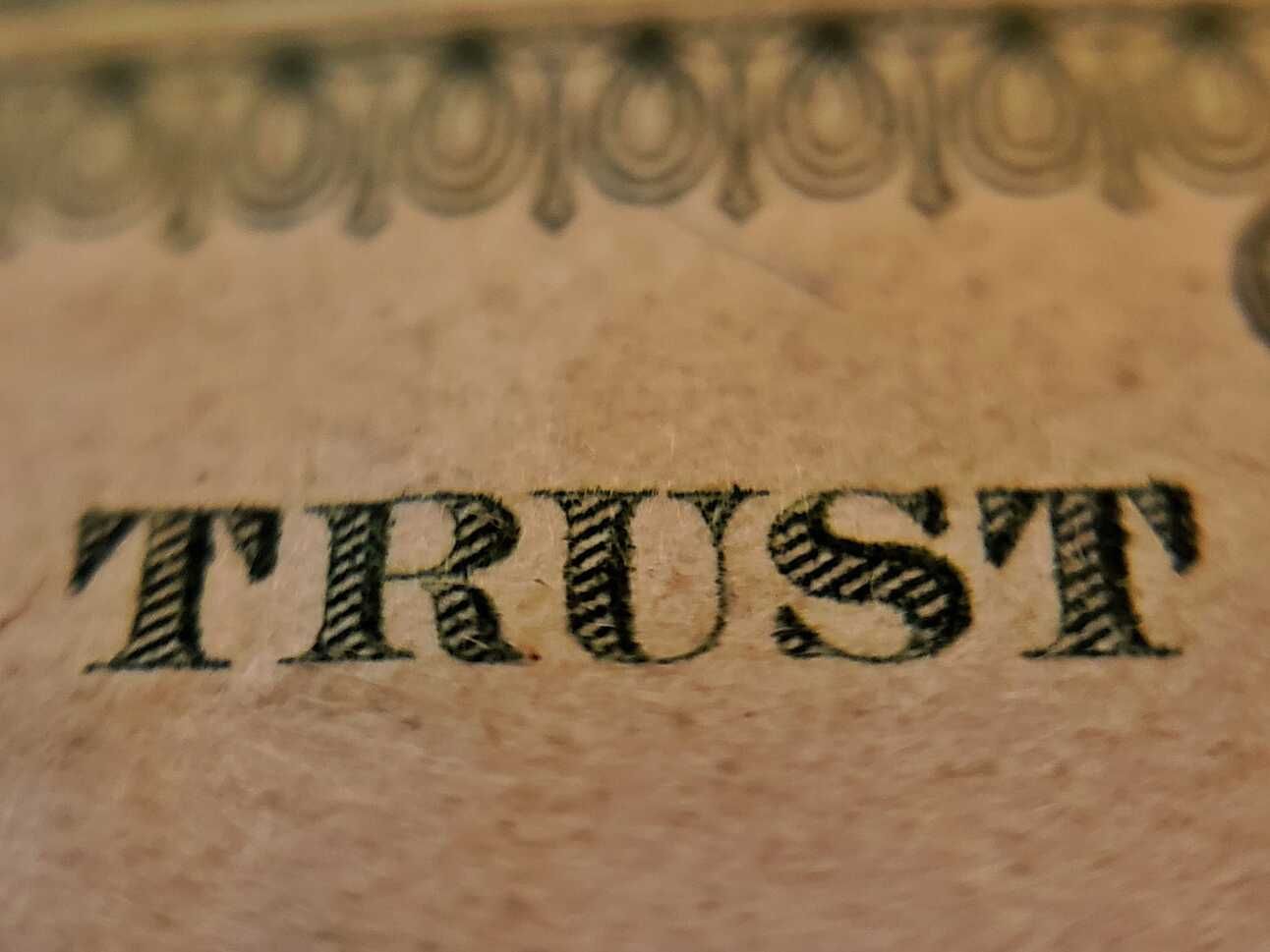