- Pascal's Chatbot Q&As
- Posts
- GPT-4: For AI developers aiming for successful adoption of their innovations in the healthcare space, particularly in precision oncology, they should consider the following strategies
GPT-4: For AI developers aiming for successful adoption of their innovations in the healthcare space, particularly in precision oncology, they should consider the following strategies
Robustness and Accuracy, Bias and Fairness, Transparency and Explainability, Data Security and Privacy, Engage with Stakeholders, Focus on Integration, Ethical Practices, Clinical and Economic Value
Question 1 of 7 for ChatGPT-4: Please read "Incorporating Integrated Diagnostics into Precision Oncology Care - Proceedings of a Workshop" and tell me what it says about Artificial Intelligence in easy to understand language
ChatGPT-4’s answer:The workshop proceedings discuss the expanding role of Artificial Intelligence (AI) in precision oncology, emphasizing how AI can enhance integrated diagnostics and overall cancer care. Here are the key points simplified:
Digitization and Machine Learning: Over 5 million pathology slides have been digitized to train machine learning algorithms to identify cancer more accurately than humans in some cases. This use of AI improves the ability to integrate data from various studies, aiding in better patient stratification and response prediction for treatments like immunotherapy.
Challenges and Limitations: Despite these advances, the effectiveness of AI is limited by the quality of the data used. Problems can arise from biases within the data, potential errors in how data are used, and the algorithms' lack of contextual understanding. This calls for better methods to assemble and integrate diverse datasets.
Ethical and Technical Concerns: The ethical design of AI is crucial. It needs to be transparent, inclusive, responsible, unbiased, trustworthy, and secure. Moreover, there are technical challenges, like the possibility of data poisoning and adversarial attacks that aim to manipulate or compromise the AI models.
Trust and Adoption in Clinical Settings: While AI offers substantial benefits by streamlining diagnostics and improving patient care quality, many clinicians are hesitant to adopt it fully. Trust issues, concerns about replacing human judgment, and the need for more transparent, understandable AI systems are notable hurdles.
Future Visions and Research Needs: Looking forward, the integration of AI in healthcare is seen as transformative, with initiatives like the "health digital twin" providing a personalized model of a patient's health that can be updated and used to make better treatment decisions. Research is ongoing to create more robust, ethical, and effective AI systems that clinicians can rely on.
Overall, while AI in precision oncology offers significant potential to enhance patient outcomes and streamline care processes, careful attention must be given to data quality, ethical considerations, and the technology's integration into clinical practice to maximize its benefits and mitigate risks.
Question 2 of 7 for ChatGPT-4: Are there any other remarkable or controversial statements being made in the document?
ChatGPT-4’s answer:The document includes several remarkable or controversial statements, particularly concerning health care disparities and challenges associated with the implementation of new technologies:
Health Care Disparities: Otis Brawley pointed out that many Americans, especially racial minorities and those with low socioeconomic status, receive "less than optimal cancer care". This suboptimal care manifests in multiple ways, such as less access to the newest cancer care tools and technologies. Furthermore, there's an indication that Black Americans and poorer Americans who need radiation therapy are more likely to receive treatment from older, less effective radiation therapy machines compared to their middle- and upper-middle-class counterparts.
Challenges with New Technologies: Implementing new technologies in health care can paradoxically worsen disparities by creating "data deserts" where some populations, especially those in rural or underfunded areas, lack access to these advancements. This concern is accentuated with technologies that require high infrastructure costs or specialized training that may not be available in all health care settings.
These statements highlight significant issues in equity and access within the health care system, especially as it pertains to the integration of new diagnostic technologies and precision oncology care.
Question 3 of 7 for ChatGPT-4: What does the document say about equitable AI algorithms?
ChatGPT-4’s answer:The document discusses the challenges and solutions involved in developing equitable AI algorithms, particularly in the context of health care and diagnostic accuracy. Here are some key points:
Data Hungry Models: AI models, especially deep learning models, require large datasets ("data hungry") and are not adept at handling shifts in the types of data they analyze. This can lead to decreased performance when the data deviates from the training set's characteristics. For instance, a model trained on images with white backgrounds performed poorly on colored backgrounds, showing the models' limitations in adapting to new types of data.
Bias in AI: The models can inadvertently learn and perpetuate the biases present in their training datasets. An example given was an image recognition model that misclassified a monkey holding a guitar as a human, presumably because guitars were associated with humans in the training data. This highlights the critical issue of inherent biases within AI training datasets.
Novel Algorithmic Solutions: The document outlines novel solutions to develop more equitable AI models. For instance, "Mirai" and "Sybil" are two risk prediction models that use imaging data to predict the likelihood of developing cancer. These models were designed to be robust across different populations by using diverse data during the training phase. This approach aims to ensure that the models perform equally well across various demographic groups.
Anti-Bias Training Solution: One proposed solution to address biases is forcing the algorithm to treat data from majority and minority populations equally by learning invariant representations. This method focuses on eliminating unnecessary differences between these groups, aiming for improved accuracy across all populations.
Distributional Shift Detection: Another approach involves the algorithm learning to detect biases automatically by splitting data in a way that highlights distributional shifts. This helps identify outliers and data points that may not conform to the general patterns seen in the training dataset.
Calibrated Selective Classification: A novel method where the algorithm learns to abstain from making predictions when there is uncertainty. This is crucial for clinical applications where making an incorrect prediction could have serious consequences. The algorithm instead reports when its predictions may not be reliable.
These points illustrate ongoing efforts to address and mitigate biases in AI applications within healthcare, aiming to ensure that AI tools are equitable, safe, and effective across diverse populations.
Question 4 of 7 for ChatGPT-4: What does the document say about ethical AI design?
ChatGPT-4’s answer:The document discusses several essential aspects of ethical AI design, focusing on the need for AI models to be both ethical and practical. Here are the main points highlighted:
Transparency and Understandability: AI models should be designed to be transparent and easy to understand. This allows users to comprehend how decisions are made, which is crucial for trust and accountability.
Inclusiveness: AI should be designed to be inclusive, meaning it should be usable and accessible by people with diverse backgrounds and needs. This includes ensuring that training data are representative of various populations to prevent biases.
Responsibility and Impartiality: Ethical AI must be responsible and unbiased. This involves careful design to avoid perpetuating or amplifying biases that might exist in the training data. There is an ongoing concern about how biases in datasets can be encoded and then amplified during training, leading to biased outputs that can perpetuate cycles of bias.
Trustworthiness and Reliability: The design of AI systems should focus on building trust and ensuring that the models are reliable. This includes addressing concerns related to adversarial attacks and data security.
Data Security and Privacy: Ensuring the security of data and protecting user privacy are paramount. The design should incorporate strong measures to secure data and guard against unauthorized access or breaches.
Detection and Addressing Bias: The document also touches on advanced methods like automatic detection of distributional shifts in data, which helps in identifying biases or anomalies that could affect the performance and fairness of AI models.
Ethical Considerations Across Disciplines: The ethical design of AI is not solely the domain of computer scientists but requires a multidisciplinary approach that includes insights from various fields to ensure that the models are ethically sound and practically useful.
These principles aim to create AI systems that are not only technologically advanced but also ethically designed to serve all users fairly and securely.
Question 5 of 7 for ChatGPT-4: If AI makers want to be successful in this space, ensuring the adoption of their innovations, what would they need to do next?
ChatGPT-4’s answer:For AI developers aiming for successful adoption of their innovations in the healthcare space, particularly in precision oncology, they should consider the following strategies:
Ensure Robustness and Accuracy: Prioritize the development of AI models that are highly accurate and robust across different clinical settings and patient populations. This involves extensive validation and testing on diverse datasets to confirm that the models perform well universally, not just under specific conditions or with certain demographic groups.
Address Bias and Fairness: Actively work to detect and mitigate biases in AI models. This can be achieved by using diverse training datasets and incorporating techniques like distributional shift detection and calibrated selective classification. Ensuring fairness will not only improve the model's performance across various demographics but also enhance trust among users.
Enhance Transparency and Explainability: Develop AI systems that are transparent and provide explainable outputs. Clinicians and patients are more likely to trust and rely on AI tools when they understand how decisions are made. This could involve creating interfaces that clearly explain the reasoning behind AI-generated recommendations or diagnoses.
Strengthen Data Security and Privacy: Implement stringent data security measures to protect patient information. Adhering to health data regulations, such as HIPAA in the United States, and ensuring that data is encrypted and securely stored is crucial for maintaining trust and complying with legal standards.
Engage with Stakeholders: Collaborate with healthcare providers, regulators, and patients to understand their needs and concerns. Regular engagement through workshops, pilot programs, and feedback sessions can help tailor AI tools to better fit clinical workflows and address specific challenges faced by healthcare professionals.
Regulatory Compliance: Navigate the complex regulatory landscape effectively. Ensuring that AI tools meet all regulatory requirements for medical devices is crucial for legal compliance and market access. This might involve working closely with regulatory bodies like the FDA to understand and meet necessary standards.
Focus on Integration: Design AI systems that can be easily integrated into existing healthcare infrastructures, such as electronic health records (EHRs) and diagnostic imaging systems. Seamless integration helps in minimizing disruptions to existing workflows and encourages wider adoption.
Provide Training and Support: Offer comprehensive training and ongoing support to healthcare providers. Educating users on how to effectively use and benefit from AI tools can facilitate smoother implementation and greater acceptance.
Demonstrate Clinical and Economic Value: Provide clear evidence of the clinical benefits and cost-effectiveness of AI tools. Demonstrations on how AI can improve diagnostic accuracy, reduce errors, and potentially lower costs can be compelling arguments for healthcare organizations to adopt such technologies.
Promote Ethical Practices: Adhere to ethical guidelines in AI development and application, ensuring that these tools do the least harm while maximizing benefits. Ethical considerations should be a core part of the development process, reflecting in every aspect from design to deployment.
By focusing on these strategies, AI developers can better position their innovations for successful adoption in the healthcare sector, especially in areas like precision oncology where the stakes and potential benefits are particularly high.
Question 6 of 7 for ChatGPT-4: If regulators want to make sure that no major issues arise in this space, what would they need to do next?
ChatGPT-4’s answer:For regulators aiming to ensure that AI in healthcare, particularly in precision oncology, is safely and effectively implemented without major issues, they could consider the following approaches:
Develop Specific Guidelines: Create detailed regulatory guidelines specifically tailored for AI applications in healthcare. These should address aspects like data quality, algorithm transparency, and fairness. Guidelines could provide a clear framework for both developers and healthcare providers.
Enhance Oversight: Establish a robust oversight mechanism to monitor the development, deployment, and ongoing use of AI systems. This could involve periodic audits, performance reporting, and compliance checks to ensure that AI tools remain safe and effective over time.
Promote Transparency: Require that AI developers disclose the methodologies, data sources, and training processes used in creating their algorithms. This transparency would enable better scrutiny and trust in the technologies being deployed.
Encourage Bias Testing and Mitigation: Mandate regular testing for bias and require the implementation of mitigation strategies before AI systems can be approved for clinical use. This could include requiring developers to test their systems across diverse populations and different clinical environments.
Strengthen Data Security Standards: Impose strict data security regulations to protect sensitive patient information. This includes setting high standards for data encryption, access controls, and breach notification protocols.
Support Interdisciplinary Collaboration: Foster collaboration between technologists, clinicians, ethicists, and legal experts to address the multifaceted challenges posed by AI in healthcare. This can help ensure that regulations are comprehensive and appropriately address all potential issues.
Facilitate Post-Market Surveillance: Implement post-market monitoring systems to continually assess the performance and impact of AI applications after they have been deployed. This could help in quickly identifying any unforeseen issues or declines in performance.
Invest in Regulatory Science: Support research in regulatory science to keep up with the rapid advancements in AI. This could involve partnerships with academic institutions and industry to develop new methods for evaluating and monitoring AI technologies.
Provide Clear Paths for Approval: Offer a streamlined yet rigorous pathway for the approval of AI applications in healthcare. This can help reduce the time to market for beneficial innovations while ensuring that they meet all necessary safety and effectiveness standards.
Educate and Engage the Public: Engage in public education campaigns about the benefits and risks of AI in healthcare. This can help manage expectations and promote an informed dialogue about the use of AI technologies in clinical settings.
International Cooperation: Collaborate with international regulatory bodies to create harmonized standards and approaches for AI in healthcare. This could help in managing the global aspects of AI technologies, which often cross national boundaries.
By focusing on these strategies, regulators can effectively manage the deployment of AI in healthcare, particularly in sensitive areas like oncology, ensuring that these powerful tools benefit patients safely and equitably.
Question 7 of 7 for ChatGPT-4: Is AI in its current state fit for purpose in the context of this discussion?
ChatGPT-4’s answer:In the context of precision oncology and healthcare, AI is advancing rapidly and showing considerable promise, but there are areas where it still needs significant improvement to be fully "fit for purpose." Here’s a breakdown of how AI stands currently:
Strengths of AI in Healthcare
Efficiency and Speed: AI can process vast amounts of data much faster than humans, which is particularly beneficial in diagnostic processes where time is critical.
Pattern Recognition: AI excels at identifying patterns in complex datasets, such as genetic information or imaging data, which can aid in early detection and diagnosis of diseases.
Predictive Analytics: AI can help predict disease progression and response to treatments by analyzing data trends, potentially leading to more personalized and effective treatment plans.
Scalability: AI can be deployed across various settings, potentially democratizing access to advanced diagnostic tools, especially in under-resourced areas.
Limitations and Challenges
Data Quality and Quantity: AI's performance is highly dependent on the quality and breadth of the data it is trained on. Incomplete or biased data can lead to inaccurate or unfair outcomes.
Transparency and Explainability: Many AI models, especially those based on deep learning, are often described as "black boxes" because their decision-making processes are not easily understood by humans. This lack of transparency can be a significant barrier in clinical settings where understanding the basis for a decision can be as important as the decision itself.
Regulatory Challenges: The regulatory landscape for AI in healthcare is still evolving. Ensuring patient safety while fostering innovation requires balanced regulations that can adapt to the rapid pace of technological change.
Ethical Concerns: Issues such as data privacy, consent, and potential biases in AI algorithms are critical ethical challenges that need to be addressed to ensure that AI applications are fair and do not perpetuate existing health disparities.
Clinical Integration: Integrating AI tools into existing healthcare workflows can be challenging. Clinicians may need training to use these tools effectively, and the tools must be compatible with existing systems.
Fit for Purpose?
AI is progressively becoming fit for purpose in healthcare, particularly in precision oncology, as technology and methods improve. However, for AI to be fully effective and widely accepted, the following are essential:
Continuous Improvement: Ongoing research and development to refine AI algorithms and ensure they are robust and reliable.
Enhanced Data Practices: Building large, diverse, and high-quality datasets with stringent privacy standards to train AI models.
Ethical Frameworks: Developing and enforcing strong ethical guidelines to govern AI use in healthcare.
Stakeholder Engagement: Including clinicians, patients, and regulators in the development and deployment process to ensure that AI tools meet real-world needs.
Regulatory Adaptation: Creating flexible regulatory frameworks that can keep pace with technological advancements while ensuring safety and efficacy.
In summary, while AI is not yet perfect, it is on a promising path towards becoming an indispensable tool in precision oncology and healthcare at large, provided that the ongoing challenges are systematically addressed.
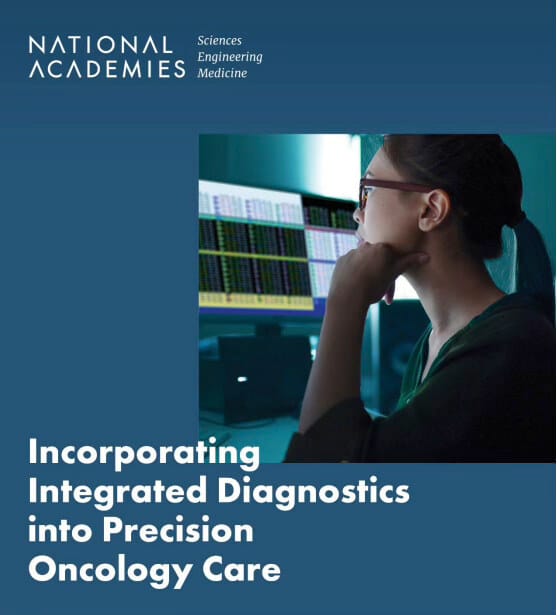