- Pascal's Chatbot Q&As
- Posts
- Gemini: The US & UK pursuit of AI-driven economic advantage and geopolitical standing appears to be consistently overshadowing a comprehensive and proactive approach to mitigating the profound risks.
Gemini: The US & UK pursuit of AI-driven economic advantage and geopolitical standing appears to be consistently overshadowing a comprehensive and proactive approach to mitigating the profound risks.
The evidence suggests that critical concerns are often being deprioritized or addressed in a reactive and fragmented manner. This imbalance carries potential for severe and wide-ranging consequences.
by Gemini Advanced, Deep Research with 2.5 Pro. Warning, LLMs may hallucinate!
Executive Summary
This report examines the trajectory of Artificial Intelligence (AI) development in the United States (US) and the United Kingdom (UK), focusing on the governments' prioritization of economic benefits and geopolitical competition, particularly with China, over comprehensive risk mitigation. Evidence suggests a prevailing emphasis on AI as an engine for economic growth and a tool for maintaining national security, often leading to the deprioritization of critical concerns such as the rule of law, environmental sustainability, power grid stability, AI's inherent technological limitations, and fundamental rights protections including privacy, copyright, and trademark law.
The drive for AI supremacy is fueled by narratives of AI as an economic panacea and a national security imperative. While official policies in both nations articulate commitments to "safe, secure, and trustworthy" AI, a discernible gap exists between this rhetoric and the de facto prioritization of rapid innovation. This "innovation-regulation dilemma" often results in a precautionary gap, where risks related to algorithmic bias, environmental degradation, power grid strain, AI unreliability, and rights infringements are addressed reactively rather than proactively.
The consequences of this path are multifaceted and severe. Societal disruptions include potential job displacement, exacerbation of inequality, and an erosion of trust in information and institutions. Environmental impacts are significant, with AI's massive energy and water consumption and e-waste generation posing substantial threats to climate goals and resource availability. The integrity of democratic processes is challenged by AI-driven disinformation and surveillance capabilities, potentially eroding the rule of law and fundamental human rights. Vulnerable populations, including marginalized communities and creative industries, are likely to bear a disproportionate burden of these negative consequences.
In the global AI arena, the US currently maintains a lead in overall investment and foundational model development, while China is rapidly closing the performance gap, excelling in cost-effective innovation and AI diffusion. The UK, a "middle power" in AI, focuses on niche strengths and strategic partnerships. Predictions suggest the US will likely maintain a narrow lead over China in the near term, but China's focus on widespread application and cost-efficiency presents a formidable challenge. The UK is unlikely to outperform China but can achieve strategic relevance through targeted efforts.
To genuinely protect citizens and businesses, the US and UK must rebalance their priorities, integrating safety, ethics, and rights as core components of their AI strategies. This requires strengthening regulatory frameworks with clear, enforceable rules for high-risk AI; investing in sustainable and robust AI infrastructure and research; rigorously protecting citizen rights and business interests through updated legal frameworks for privacy, IP, and fair competition; and fostering international cooperation to establish global norms for responsible AI. Ultimately, true leadership in the AI era will be defined not only by technological advancement but by the wisdom to deploy AI for the genuine and equitable benefit of all.
I. The Drive for AI Supremacy: Stated Ambitions and Underlying Priorities in the US and UK
The rapid advancement of Artificial Intelligence (AI) has spurred an intense global competition, with nations vying for technological leadership, economic advantage, and geopolitical influence. The United States (US) and the United Kingdom (UK) have prominently positioned themselves as key players in this race, articulating ambitious strategies to harness AI's transformative potential. However, an examination of their official narratives and de facto priorities reveals a complex interplay between the pursuit of innovation and the management of profound risks. A central assertion is that the drive for economic uplift and strategic advantage, particularly in competition with nations like China, often overshadows comprehensive attention to the rule of law, environmental sustainability, critical infrastructure resilience, the inherent limitations of AI technology, and fundamental rights protections.
A.1. Economic Aspirations and the "Solve-All" Narrative
A primary driver behind the governmental push for AI development in both the US and UK is the profound belief in its capacity to revolutionize economies and solve complex societal challenges. This techno-optimistic viewpoint often frames AI as a catalyst for unprecedented growth and efficiency.
In the US context, official discourse strongly emphasizes AI as a cornerstone of future economic prosperity. A memorandum from October 2024 underscores the US government's prioritization of AI advancement to secure economic benefits and maintain a competitive edge, highlighting AI's potential for "great benefits" if deployed appropriately.1 This sentiment is echoed by economic institutions; for instance, Federal Reserve statements acknowledge AI's "enormous promise of faster economic growth, advances in human health, and a higher standard of living".2 Such pronouncements reflect a widespread expectation that AI will be a significant engine for economic transformation and productivity gains, aligning with a broader narrative that sometimes borders on viewing AI as a panacea for diverse challenges.
Similarly, the UK's AI strategy is heavily oriented towards economic upliftment. The AI Opportunities Action Plan, published in January 2025, sets a core ambition of unlocking substantial economic benefits, projecting that AI could contribute an average of £47 billion annually to the UK economy over the next decade.3 This plan builds upon the 2021 National AI Strategy, which aimed to translate AI's vast potential into "better growth, prosperity and social benefits" for the nation.5 A key element of this strategy is the stated intention to cultivate the "most pro-innovation regulatory environment in the world" 5, signaling a clear emphasis on fostering AI development to achieve these economic goals and position the UK as an "AI superpower".6
The consistent promotion of AI by both US and UK governments as a solution to economic challenges and a driver of future prosperity serves to justify significant public and private investment and fosters a generally permissive developmental environment. This "economic panacea" narrative is powerful; as governments face persistent pressures to deliver economic growth and improve citizens' quality of life, AI is presented as a uniquely transformative technology capable of meeting these demands.2 This narrative is prominently featured in official strategies and policy documents to garner widespread support from both the public and industry.1However, this intense focus on anticipated benefits can inadvertently create an environment where the multifaceted risks associated with AI are downplayed, deferred, or viewed as secondary impediments to achieving the promised economic outcomes. The UK's AI Action Plan, for example, has drawn criticism for cultivating an impression that AI is a "magic bullet" capable of single-handedly revitalizing the economy.4 Such a perspective risks fostering a "growth at all costs" dynamic, potentially leading to an underestimation or delayed addressing of AI's significant societal and environmental costs.
A.2. The Geopolitical AI Arms Race: Competition with China as a Primary Motivator
Beyond economic aspirations, the strategic imperative to compete on the global stage, particularly with China, serves as a powerful catalyst for accelerated AI development in the US and, to a more nuanced extent, the UK. This geopolitical dimension frames AI not just as an economic tool but as a critical element of national power and security.
In the US, AI development is explicitly and frequently viewed through the prism of national security and strategic rivalry. The October 2024 memorandum on AI explicitly states that the US "must lead the world" in this domain and warns of the risks of ceding ground to strategic competitors if the nation does not act with urgency and in concert with industry, civil society, and academia.1 The AI arms race is often characterized as a comprehensive "contest for economic leadership, military superiority, and geopolitical influence".7 This perspective is amplified by numerous think tanks and experts who stress the urgency for the US to "regain tech supremacy" 8 and translate its early advancements into an "enduring advantage" over China.9 The "AI race" with China is thus a dominant and pervasive theme in US policymaking, driving substantial investment and shaping strategic decisions, frequently couched in the language of national security.
The UK's position within this geopolitical contest is more complex. The 2021 National AI Strategy clearly articulated an ambition to compete with global AI leaders, including the US and China.6 Discussions among UK policymakers and at influential forums readily acknowledge the "AI race" with China.10 However, there is also a strong current of pragmatism, with calls for a realistic assessment of the UK's capacity to "outcompete" a technological giant like China directly.10 Instead, the UK appears to be focusing on leveraging its unique strengths, such as its "convening authority and talent" pool, and its influential higher education institutions.10 The UK government's official approach to China is often described by the "three C's": cooperate, challenge, and compete, though this multifaceted stance has faced criticism for a perceived lack of clear direction and transparency.10 This suggests that while the UK is acutely aware of the competitive landscape, its strategy is geared towards strategic partnerships and carving out niches of influence, reflecting its "middle power" status 10, rather than engaging in an all-encompassing direct competition.
The framing of AI development as a national security imperative, particularly pronounced in the US, creates a policy environment where rapid advancement is paramount, potentially leading to a higher tolerance for the multifaceted risks associated with the technology. When geopolitical competition is a primary concern for policymakers 1, and AI is identified as a critical technology for future military and economic dominance 7, the prospect of "losing" the AI race can be portrayed as a direct threat to national security and global standing.1 This inherent urgency can foster policies that prioritize the fast-tracking of AI development, potentially at the expense of comprehensive risk assessment and mitigation strategies, as these safeguards might be perceived as impediments that slow down crucial progress. Such an "arms race" mentality risks cultivating a "move fast and break things" ethos, where ethical considerations, environmental impacts, and broader societal safeguards are relegated to secondary importance in the pursuit of technological superiority. This could inadvertently lead to a global race to the bottom on safety and ethical standards, as the focus on "winning" overshadows the critical importance of "winning responsibly."
A.3. Official Stances vs. De Facto Prioritization of Risks (Rule of Law, Environment, Grid, AI Robustness, Rights Protections)
While both the US and UK governments articulate commitments to responsible AI development, a disparity often emerges between official pronouncements and the de facto prioritization of risks in policy and practice. This gap is crucial to understanding the user's central concern about deprioritized safeguards.
The US official stance, as articulated in foundational documents like Executive Order 14110 and related memoranda, consistently emphasizes the importance of "safe, secure, and trustworthy" AI development and deployment.1 There is a stated commitment to the protection of human rights, civil rights, civil liberties, privacy, and safety throughout the AI lifecycle. The Department of State's Enterprise AI Strategy (EAIS), for instance, centers on the "responsible and ethical design, development, acquisition, and appropriate application of AI".13 These official documents paint a picture of a government keenly aware of AI's potential downsides and dedicated to mitigating them.
However, US de facto prioritization and criticisms suggest a more complex reality. Numerous critics point to a persistent "federal policy vacuum" concerning comprehensive AI governance.14 This absence of overarching federal legislation has led to a "patchwork" of state-led initiatives, which, while sometimes innovative, may not be uniformly equipped to address the full spectrum of AI risks or resist industry pressures for lighter regulation.14 Public trust in AI is reportedly declining due to mounting concerns over algorithmic bias, data privacy violations, and a lack of accountability, yet federal legislative action specifically targeting these AI-driven challenges is perceived by many as slow or insufficient.14 The continued lack of comprehensive federal data privacy legislation, for example, leaves American citizens and their data in a vulnerable position.14 Even statements from key economic bodies like the Federal Reserve tend to offer scenarios for AI's impact rather than outlining immediate, stringent regulatory interventions.2 The government itself appears to be struggling to keep pace with the furious speed of AI advancement, with no new federal laws enacted to specifically regulate AI and its unique challenges.16 This suggests that despite official commitments to responsible AI, the practical emphasis on fostering innovation and maintaining a competitive edge may be leading to a reactive, rather than proactive, approach to comprehensive risk management.
The UK's official stance mirrors many of the US commitments. The government's AI Playbook for the public sector, for example, champions principles such as "use AI lawfully, ethically and responsibly" and ensuring "meaningful human control at the right stages".3 The 2023 white paper on AI regulation clearly states the government's aim to implement a "proportionate, future-proof and pro-innovation framework".3 The establishment of the UK AI Safety Institute was a significant step intended to deepen understanding and mitigate the risks posed by highly advanced AI models.4Furthermore, the government has expressed its intention to "apply AI to help solve global challenges like climate change" and to protect the "safety, security, choices and rights of our citizens".5
Nevertheless, UK de facto prioritization and criticisms also indicate a potential divergence between stated goals and implemented policy. The UK's AI Opportunities Action Plan has faced significant criticism for "glossing over or ignoring outright" critical risks related to safety, security, and particularly environmental implications.4Indeed, reports suggest the plan contains "no mention of the environment or climate" beyond addressing the sustainability and security risks of AI infrastructure, rather than the intrinsic environmental impact of AI development and deployment itself.18 The government's overarching "pro-innovation" regulatory approach is viewed by some experts as a "light-touch strategy" that may prove inadequate in addressing the "major security, privacy and ethical issues" inherent in widespread AI adoption.19Adding to these concerns, both the UK and the US notably declined to sign an international agreement in Paris focused on "ethical" AI development, citing national security considerations.19 This decision could be interpreted as prioritizing strategic autonomy and national interests over collective, international ethical commitments. The UK's current approach of tasking existing regulators with interpreting and applying broad AI principles, without new explicit legal obligations or significantly enhanced enforcement powers, is also seen by some observers as potentially insufficient to meet the scale of the challenge.20
This dynamic in both nations points to an "innovation-regulation dilemma," where a perceived tension exists between the desire to foster rapid AI innovation—to capture economic benefits and compete globally—and the need to implement robust, potentially innovation-slowing, regulations. This often results in a "precautionary gap," where concrete action on mitigating risks lags considerably behind the swift pace of technological development. Governments naturally desire to be at the forefront of AI innovation.1 Consequently, strict, early-stage regulation is often portrayed by industry stakeholders and some policymakers as a significant hindrance to innovation and national competitiveness.19 This leads to official policies that frequently emphasize "pro-innovation" strategies, regulatory flexibility, and leveraging existing, often ill-suited, regulatory frameworks.3 The outcome, as critics highlight, is that risks are often "played down" 4, or a "federal policy vacuum" persists 14, creating a dangerous interval where potential harms can manifest and become entrenched before adequate safeguards are developed and implemented. This situation is compounded by growing public concern, as citizens perceive a lack of effective governance from institutions they traditionally trust.15 This precautionary gap can ultimately lead to a scenario where societal adaptation to AI's negative consequences is reactive and potentially inadequate. It also fosters significant uncertainty for businesses attempting to navigate a fragmented, inconsistent, and constantly evolving regulatory landscape.14The decision by the US and UK to abstain from certain international agreements on ethical AI 19 further signals a prioritization of national strategic interests over globally harmonized safety standards, potentially exacerbating the "arms race" dynamic and its associated risks.
Table 1: Comparative Overview of US, UK, and China AI Strategies
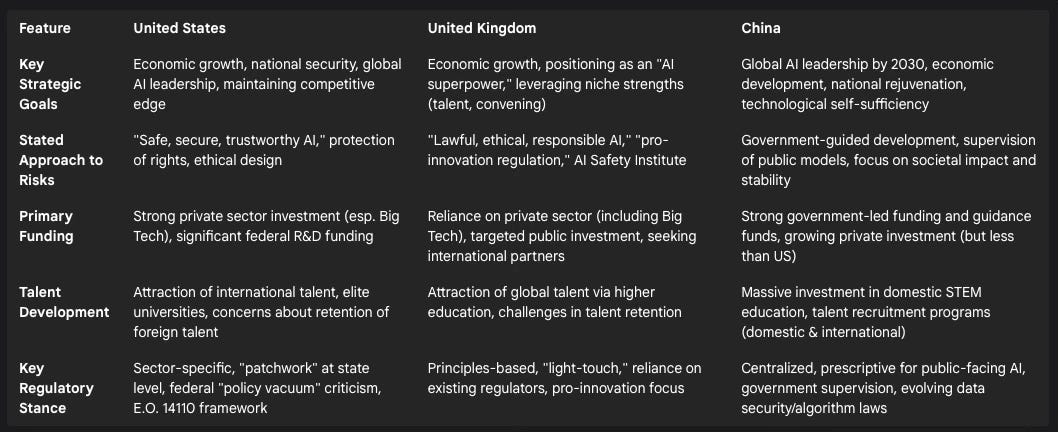
This table provides a condensed comparison of the AI strategies of the US, UK, and China, highlighting their differing approaches to balancing innovation with risk management, which is central to the issues discussed.
II. A Landscape of Neglected Threats: Unpacking the Risks of Unfettered AI Development
The pursuit of AI supremacy, if not carefully balanced with robust safeguards, risks unleashing a cascade of threats that could impact the very fabric of society. The concerns span the integrity of legal and democratic systems, environmental stability, the resilience of critical infrastructure, the fundamental reliability of AI technology itself, and the protection of individual and collective rights. A systematic examination of these threats, supported by available evidence, reveals a landscape where the potential downsides of unfettered AI development are significant and often deprioritized in the race for innovation and geopolitical standing.
B.1. Erosion of the Rule of Law and Democratic Processes
The integration of AI into societal systems presents profound challenges to the rule of law and the functioning of democratic processes, primarily through algorithmic bias, a lack of transparency and accountability, and the malicious use of AI to manipulate information and influence public discourse.
Algorithmic Bias and Discrimination: A critical threat stems from AI systems inheriting and amplifying biases present in their training data or embedded in their design choices.3 When deployed in sensitive contexts such as the legal system (e.g., for bail decisions, sentencing recommendations, or civil litigation support), employment, or social services, these biased algorithms can lead to discriminatory outcomes that disproportionately affect marginalized communities. This fundamentally undermines the principle of equality before the law. The UK's AI Playbook itself acknowledges that AI models trained on unrepresentative datasets can display biases and produce harmful outputs.3 Historical precedents, such as the Dutch child benefits scandal or the UK's Post Office Horizon scandal, where flawed automated systems led to grave injustices, serve as stark warnings of the potential consequences, even if these were not purely AI-driven.28 In civil litigation, for instance, an AI tool biased by historical data reflecting societal inequities might undervalue claims made by individuals from certain demographic groups, thereby clashing with non-discrimination principles and eroding public confidence in judicial impartiality.28Legally, the failure to adequately address AI bias can result in breaches of equality legislation, such as the UK's Equality Act 2010, and contravene data protection principles like fairness and accountability enshrined in the UK GDPR.28
Transparency and Accountability Gaps (The "Black Box" Problem): Many advanced AI systems, particularly those based on complex machine learning models like deep neural networks, operate as "black boxes".29 Their internal decision-making processes are often opaque even to their developers, let alone to the individuals affected by their outputs. This lack of transparency poses a significant challenge to accountability. If an AI system causes harm or makes an erroneous decision, the inability to understand why that decision was made makes it exceedingly difficult to assign responsibility, rectify errors, or provide meaningful redress to those affected. This opacity directly challenges the ability of individuals to contest AI-driven decisions, a fundamental tenet of the rule of law.29 This "accountability gap" is a serious concern: if the reasoning behind AI decisions cannot be adequately explained or understood, holding any party accountable for harmful or unjust outcomes becomes a formidable, if not impossible, task.31 While techniques for Explainable AI (XAI) are being developed to shed light on these processes, they currently face significant limitations. These include an inherent trade-off between the accuracy of a model and its explainability (more complex, accurate models are often harder to explain), the subjective nature of what constitutes a "good" explanation for different audiences, and various technical challenges in generating consistently reliable and comprehensive insights into model behavior.32 Indeed, it is suggested that full transparency in the most complex Large Language Models (LLMs) may not even be achievable with current paradigms.33
Impact on Democratic Processes: AI technologies provide powerful new tools for manipulating public opinion, disrupting electoral processes, and eroding trust in democratic institutions.34 Sophisticated disinformation campaigns, leveraging AI-generated content such as deepfakes (hyper-realistic but fabricated videos or audio) and fake news articles, can be deployed at scale to mislead voters and sow social discord. Foreign adversaries are documented as using AI to augment their election interference capabilities, while domestic political campaigns have been found to leverage deepfake technology to create misleading advertisements or attack opponents.34 AI can also be used to automate voter suppression efforts or to create vast networks of political bots that artificially amplify certain narratives or drown out dissenting voices.34 The increasing sophistication and proliferation of AI-generated content make it progressively harder for citizens to distinguish truth from falsehood, thereby polluting the information ecosystem and undermining trust in reliable sources of information, including traditional media.35 Beyond these manipulative uses, governments themselves are increasingly harnessing AI to enhance and refine online censorship. Legal frameworks in numerous countries now mandate or incentivize digital platforms to deploy machine learning algorithms to identify and remove disfavored political, social, or religious speech.38 Such capabilities can be readily used to suppress legitimate free speech, silence dissent, and consolidate authoritarian control.39
These distinct threats to the rule of law are not isolated; they are deeply interconnected and can create a reinforcing cycle of erosion. Algorithmic bias embedded in AI systems 28 can lead to demonstrably unfair or discriminatory decisions within legal and administrative processes. The inherent "black box" nature of many of these systems 29 then makes it exceptionally difficult for affected individuals or oversight bodies to identify, challenge, or rectify these biased decisions, thereby undermining accountability. If AI is simultaneously employed to disseminate disinformation or manipulate public discourse concerning these very issues of bias and fairness 35, it becomes even more challenging for citizens to understand the scope of the problems or to effectively advocate for necessary changes. This confluence of factors can lead to a significant decline in public trust—trust in the legal system, trust in governmental institutions, and trust in the media—as these entities may be perceived as either unable or unwilling to control AI's negative impacts. A governmental approach that prioritizes the rapid deployment of AI over diligently addressing these fundamental rule of law concerns could inadvertently foster an environment where such erosion accelerates, leading to a weakened rule of law, increased social unrest, reduced civic participation, and a greater societal susceptibility to authoritarian tendencies, as opaque and unaccountable systems increasingly make decisions that profoundly impact citizens' lives. The absence of clear accountability mechanisms can also shield powerful actors, both state and private, from responsibility for AI-induced harms.
B.2. Environmental Unsustainability: Energy, Water, and E-waste Crises
The computational demands of developing and deploying advanced AI systems, particularly large-scale generative models, are giving rise to significant environmental concerns related to energy consumption, water usage, and electronic waste generation. These impacts, if unmitigated, threaten to undermine global sustainability efforts.
Energy Consumption: The training and operation of large AI models are extraordinarily energy-intensive processes, primarily concentrated in data centers that house the necessary computational infrastructure.40 Global electricity demand from data centers is projected to more than double by 2030, with AI identified as a principal driver of this increase.42 In the US, data centers are forecasted to account for as much as 13% of total national electricity consumption by 2030, a substantial rise from 4% in 2024 41, and could be responsible for nearly half of the growth in US electricity demand by that year.42 Similarly, in Europe, AI's energy needs are expected to constitute 4-5% of total electricity demand by 2030.41 This surge in demand is particularly concerning as it often relies on existing energy grids, a significant portion of which may still be powered by fossil fuels, thereby exacerbating carbon dioxide emissions and hindering progress towards climate goals.40 The UK's AI Opportunities Action Plan has drawn criticism for not adequately addressing these environmental implications, particularly the plan to offer firms developing frontier AI guaranteed access to energy resources at a time when the UK's national grid is already under considerable pressure.4
Water Consumption: Beyond electricity, data centers consume vast quantities of freshwater, primarily for cooling the powerful hardware required for AI computations.40This "blue water" is drawn from rivers, lakes, and groundwater sources that are often vital for local communities and ecosystems. Research indicates that the training of a single large AI model like GPT-3 at Microsoft's US data centers was estimated to consume approximately 5.4 million liters of water.45 On a more granular level, generating a short, 100-word email using a model like GPT-4 can have a water footprint equivalent to a 500ml bottle of water.46 Projections suggest that global water withdrawals attributable to AI could reach between 4.2 and 6.6 billion cubic meters by 2027, a volume comparable to roughly half the total annual water consumption of the United Kingdom.46 Such intensive water use can strain municipal water supplies, particularly in arid or water-scarce regions, leading to competition for resources and potential disruption to local ecosystems.40
E-waste Generation: The AI industry's rapid pace of innovation, characterized by frequent hardware upgrades (e.g., more powerful Graphics Processing Units (GPUs) and Tensor Processing Units (TPUs)), contributes to a growing problem of electronic waste (e-waste).43 The manufacturing of this specialized AI hardware is itself environmentally taxing, involving energy-intensive mining processes for rare earth metals and the use of potentially toxic chemicals, which can lead to pollution, habitat destruction, and significant carbon emissions.40 As newer, more capable hardware becomes available, older components quickly become obsolete, generating a continuous stream of discarded electronics. It is estimated that generative AI alone could contribute between 1.2 and 5 million metric tons of e-waste annually by the year 2030.43 Much of this e-waste, if not managed responsibly, ends up in landfills, where toxic metals such as lead, mercury, and cadmium can leach into the soil and contaminate groundwater, posing risks to environmental and human health.47Recognizing these impacts, bodies like the UK government have been urged to expand environmental reporting mandates for data centers to include e-waste recycling metrics 44, and the US Government Accountability Office (GAO) has highlighted policy options for improving data collection on e-waste generation and management.48
The current trajectory of AI development appears to be implicitly subsidized by the environment, as the full ecological costs—encompassing carbon emissions from energy use, depletion of freshwater resources, and pollution from e-waste—are often not adequately priced into the development and deployment of AI systems. This lack of comprehensive environmental accounting incentivizes practices that are unsustainable in the long term. The intense drive for rapid innovation and the pursuit of economic benefits 1, coupled with insufficient mandatory and comprehensive environmental reporting for AI operations and hardware lifecycles 44, means these substantial environmental costs are frequently externalized. This creates a scenario where the perceived cost of AI development is significantly lower than its true societal and environmental cost, effectively constituting a hidden environmental subsidy. Such a subsidy can lead to a misallocation of resources, favoring AI solutions that are environmentally damaging over potentially more sustainable alternatives. It also carries the risk of locking in unsustainable infrastructure and operational practices, making future mitigation efforts far more costly and challenging. This directly contradicts the stated goals of leveraging AI to address global challenges like climate change 5, if the development of AI itself becomes a major environmental burden.
B.3. Power Grid Instability and Infrastructure Strain
The exponential growth in energy demand driven by AI data centers is placing unprecedented pressure on electricity grids in the US, Europe, and other developed nations, many of which are already strained or aging.41 Grid operators are increasingly finding it challenging to meet the power requirements of the burgeoning AI industry, with some AI companies reporting struggles to secure the necessary electricity for their operations.49
In the United States, projections indicate a dramatic increase in power consumption by data centers. This surge is expected to contribute significantly to overall growth in electricity demand, potentially leading to notable electricity price hikes for all consumers. One analysis suggests an 8.6% increase in US electricity prices by 2030 under scenarios where renewable energy capacity growth is constrained and transmission infrastructure expansion is limited.50 The UK faces similar challenges; while aiming to decarbonize its national grid by 2030, AI data centers alone could account for up to 6% of total UK electricity demand by that year, a significant increase from around 1% currently.52
The rapid and sometimes unpredictable energy consumption patterns of large data centers also introduce new vulnerabilities to grid stability. Incidents such as the unexpected disconnection of 60 data centers in Northern Virginia's "Data Center Alley," which caused a surge of excess electricity onto the grid, highlight these emerging risks.51 Much of the existing grid infrastructure in countries like the US was constructed decades ago (often in the 1960s and 1970s) and is in dire need of modernization and upgrades to handle these new, power-intensive loads reliably.49
Recognizing the scale of this challenge, governments are beginning to take action. The US Department of Energy (DOE), for example, has initiated plans to identify federal lands suitable for co-locating data centers with new energy infrastructure, including fast-tracking permits for new energy generation facilities such as nuclear power plants.53 While AI itself offers potential solutions for optimizing energy systems, enhancing grid efficiency, and managing smart grids 49, the sheer magnitude of energy demand growth from AI development and deployment is the primary concern.
This situation creates an "AI energy paradox": AI is simultaneously promoted as a technology capable of optimizing energy systems and contributing to the fight against climate change, yet its own development and operation are creating an unprecedented surge in energy demand that threatens grid stability and could potentially increase reliance on fossil fuels if the deployment of clean energy sources cannot keep pace. The computational power required for training and deploying advanced AI models is immense and growing at an alarming rate.40 This surge is not only straining existing power grids 41 but may also necessitate a continued or even increased reliance on non-renewable energy sources, particularly if the build-out of green energy capacity and transmission infrastructure lags behind AI's voracious appetite for power 40—some analyses even suggest a "long tailwind for gas production" to meet this demand. If governments prioritize rapid AI rollout for economic or competitive reasons without fully internalizing and addressing this paradox, they risk implementing energy policies that primarily accommodate AI's escalating energy consumption rather than fundamentally greening AI's operational footprint. This could undermine broader climate goals and lead to increased energy costs for all consumers, transforming a potential part of the solution into a significant part of the problem.
B.4. Inherent AI Flaws: Accuracy, Robustness, and Explainability Challenges
Despite the remarkable capabilities demonstrated by modern AI systems, they possess inherent flaws related to accuracy, robustness, and explainability that can lead to significant risks if not adequately addressed. These are not merely teething problems but reflect fundamental limitations in current AI paradigms.
Accuracy and Reliability: AI models, particularly those based on machine learning, can produce inaccurate outputs, make erroneous predictions, or generate "hallucinations"—confident but entirely fabricated information. A notable example is the generation of fake case citations by AI tools intended for legal research.54 The reliability of AI is heavily dependent on the quality and nature of the data used for training. Poor data quality—characterized by inconsistencies, incompleteness, biases, or outdated information—is a primary barrier to the success of AI agents, often leading to incorrect responses, user frustration, and a failure to achieve intended outcomes.56Model design flaws also contribute to unreliability. "Overfitting" occurs when a model essentially memorizes its training data, including noise, but fails to generalize effectively to new, unseen data. Conversely, "underfitting" results when a model is too simplistic to capture the underlying patterns in the data.56 Furthermore, "data drift"—changes in the statistical properties of real-world data over time—can render previously accurate models unreliable as the input data diverges from what the model was trained on.56 It is also critical to recognize that even high overall accuracy metrics can mask an unacceptable number of false positives or critical errors in specific, high-stakes contexts, such as medical diagnostics, where a single misclassification can have severe consequences.56
Robustness and Security: AI models exhibit significant vulnerabilities to various forms of attack and manipulation. A key concern is their susceptibility to "adversarial attacks," where subtle, often human-imperceptible alterations are made to input data (e.g., an image or audio file) with the intent of causing the model to make incorrect predictions or classifications.58 Research indicates that even existing detection methods designed to identify AI-generated content demonstrate limited robustness against such sophisticated attacks.58 Even the most advanced AI-generated face detection models have been shown to be easily deceived by these minor adversarial perturbations.58 Other security vulnerabilities include "data poisoning," where malicious data is deliberately injected into training sets to corrupt the model's learning process; "model inversion" or "model stealing," where attackers attempt to gain unauthorized access to a model's architecture or proprietary parameters; and the exploitation of inherent model biases for malicious ends.62 The UK's AI Playbook acknowledges these emerging cyber threats to AI systems and notes that attackers are also leveraging AI itself to create new and more sophisticated threats.3
Explainability (The "Black Box" Problem): As discussed previously in the context of the rule of law, the "black box" nature of many complex AI systems remains a fundamental challenge.29 The inability to clearly understand why an AI system arrived at a particular decision or prediction is critical not only for accountability but also for debugging, ensuring fairness, identifying biases, and ultimately building user trust. While Explainable AI (XAI) techniques aim to provide insights into these processes, they come with their own set of limitations, including potential trade-offs with model accuracy, the inherent subjectivity of what constitutes a satisfactory explanation for different users, significant computational overhead, and the risk of creating a false sense of confidence in a model's reasoning if the explanations themselves are incomplete or misleading.32
These issues collectively point to a "brittle intelligence" dilemma. Despite rapid advancements in AI capabilities, many contemporary systems exhibit a form of intelligence that, while impressive on specific, narrowly defined tasks under ideal conditions, often lacks the common-sense reasoning, adaptability, and true robustness of human intelligence. This makes them prone to unexpected and sometimes catastrophic failures when deployed in complex, dynamic, real-world environments or when faced with adversarial inputs. AI models are typically trained on specific datasets to perform particular objectives.54 However, real-world operational environments are inherently dynamic, messy, and often contain data distributions or novel situations not adequately represented in the initial training data.27 This discrepancy leads to problems such as poor generalization to new scenarios, heightened susceptibility to data drift over time, and critical vulnerabilities to carefully crafted adversarial attacks.56 The "black box" nature of many of these systems 29further complicates matters, making it difficult to predict, understand, or preempt these failure modes. A governmental push for the rapid deployment of AI, without first fully addressing these fundamental issues of brittleness, can lead to the premature introduction of systems that are unreliable or unsafe in critical applications such as healthcare, finance, transportation, and national security. Over-reliance on such brittle AI systems without adequate human oversight, rigorous validation, and robust safety protocols can lead to significant societal risks, ranging from financial losses and reputational damage to safety-critical failures and a profound erosion of public trust in technology. The ambitious narrative of AI "solving all physics and curing all diseases" risks overlooking these fundamental limitations and the potential for catastrophic error inherent in current AI paradigms.
B.5. Infringement of Fundamental Rights: Privacy, Copyright, and Trademark
The unfettered development and deployment of AI technologies pose direct and significant threats to fundamental human rights, particularly in the realms of privacy, copyright, and trademark law. These infringements are not merely theoretical but are already manifesting in various forms.
Privacy: AI systems, especially those employed for surveillance purposes like facial recognition, predictive policing, and mass monitoring, often rely on the collection and analysis of vast quantities of personal data.29 This data is frequently gathered without the explicit, informed consent of individuals, raising profound privacy concerns. AI can exacerbate existing surveillance capabilities by enabling the automated analysis of these large datasets, potentially leading to widespread and unchecked monitoring of individuals' activities, associations, and private lives. This can result in misuse of sensitive information, discriminatory profiling, and a pervasive "chilling effect" on freedom of expression and association, as individuals become wary of being constantly watched.64 Furthermore, the concentration of sensitive personal data within AI systems makes them attractive targets for cyberattacks, increasing the risk of data breaches and the unauthorized leakage of private information.65 While data protection regulations like the EU's General Data Protection Regulation (GDPR) and the California Consumer Privacy Act (CCPA) aim to safeguard personal data, they face considerable challenges in effectively governing AI systems. These challenges include ensuring genuine data minimization when vast datasets are deemed necessary for model training, providing meaningful transparency about complex algorithmic processing, and mitigating biases embedded in AI systems that process personal data.30
Copyright: The rise of generative AI has precipitated a crisis in copyright law. These AI models are typically trained on massive datasets that often include copyrighted materials—such as books, articles, images, music, and code—scraped from the internet without the permission of or compensation to the original rights holders.67This practice has led to a wave of high-profile copyright infringement lawsuits filed by authors, artists, publishers, and media organizations against AI developers. Key legal battles currently revolve around the interpretation of "fair use" (in the US) or "fair dealing" (in the UK) exceptions to copyright, and whether the training of AI models on copyrighted works without a license falls within these exceptions.68 Another critical question is whether the outputs generated by these AI models themselves constitute infringing derivative works. The UK government's ongoing consultation on copyright and AI aims to strike a balance between fostering innovation and protecting intellectual property rights, but its proposals have caused alarm among creative industries who fear a weakening of their protections.67 The existing provisions in UK copyright law for "computer-generated works" (Section 9(3) of the Copyright, Designs and Patents Act 1988) are being re-examined in light of the sophisticated capabilities of modern AI.69 There exists a fundamental disagreement between AI developers, who often argue for broad access to training data as essential for innovation, and creators, who seek to maintain control over their works and receive fair compensation for their use in AI systems.68
Trademark: AI technologies also present new and complex challenges to trademark law. One significant issue is the use of AI to generate counterfeit goods, create fake product reviews, and build highly convincing counterfeit websites that mimic legitimate brands. These practices can deceive consumers, dilute brand value, and cause substantial financial harm to businesses.72 Assessing the "likelihood of confusion"—a cornerstone of trademark infringement analysis—becomes more complicated when AI systems are involved in the purchasing process. AI algorithms may perceive similarities and differences between trademarks in ways that diverge significantly from human consumer perception, potentially leading to purchasing decisions that would constitute infringement if made by a human.76 Furthermore, the question of whether marks generated by AI can meet the criteria for distinctiveness and thus be eligible for trademark protection is an emerging area of legal uncertainty.72
The development of powerful AI models, especially generative AI, is creating a fundamental tension between the desire of AI developers to utilize vast quantities of publicly available (and often copyrighted) data as a form of "digital commons" for training their systems, and the concurrent efforts of rights holders to enforce "proprietary enclosure" through the robust application of intellectual property law. This conflict has profound implications for the future of innovation, the principles of fair compensation, and the economic viability of creative industries. Generative AI models achieve their impressive performance partly due to the sheer volume and diversity of the datasets they are trained on.54 A significant portion of this data, encompassing text, images, audio, and code, is sourced from the internet, frequently including material protected by copyright.69 AI developers often contend that the use of this data for training purposes constitutes fair use or is a necessary prerequisite for technological advancement and innovation.70 Conversely, rights holders—authors, artists, musicians, publishers, and media companies—argue that this practice amounts to mass copyright infringement on an unprecedented scale, which devalues their creative work, undermines their livelihoods, and erodes their ability to control the use of their intellectual property.67 Governments find themselves caught in the middle of this dispute, attempting to balance policies that are "pro-innovation" with the long-standing need to protect IP rights.67 This has led to a surge in complex and costly litigation 70 and increasingly urgent calls for new legal frameworks, licensing models, or compensation mechanisms to address the unique challenges posed by AI.67 If intellectual property rights are not adequately protected in the age of AI, it could disincentivize human creativity and the production of high-quality original content, thereby paradoxically harming the richness of the very "digital commons" upon which future AI development might depend. Conversely, overly restrictive or poorly conceived IP enforcement could stifle beneficial AI innovation. This tension highlights a fundamental conflict over value creation, attribution, and distribution in the AI era, with significant economic and cultural consequences for creative industries and the broader information economy. A governmental approach that deprioritizes these rights protections would inevitably tilt the balance heavily in favor of AI developers, potentially at great and irreparable cost to creators and the cultural ecosystem.
Table 2: Summary of Key AI-Related Threats and Vulnerabilities
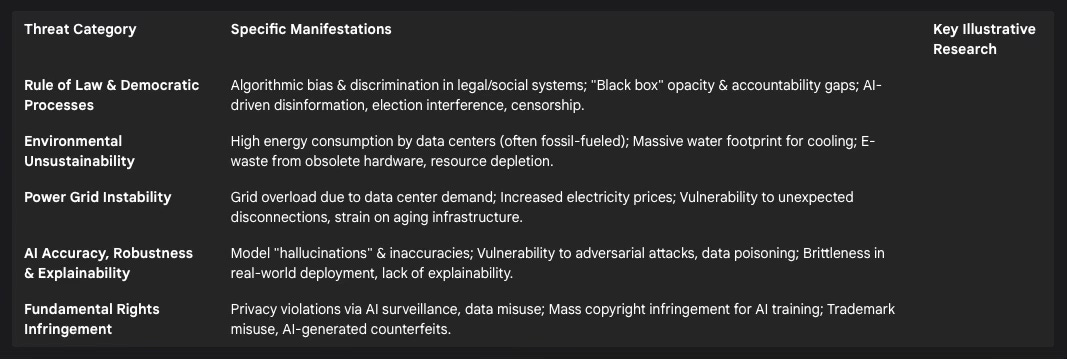
This table synthesizes the diverse threats associated with unfettered AI development, linking them to specific manifestations and supporting evidence, thereby reinforcing the breadth of risks under consideration.
Continue reading here (due to post length constraints): https://p4sc4l.substack.com/p/gemini-the-us-and-uk-pursuit-of-ai
