- Pascal's Chatbot Q&As
- Posts
- Gemini on Copyright Office's RFI: a broad fair use finding for training (A) combined with weak liability rules (C) & lack of transparency (D) creates a system where infringement can occur at scale...
Gemini on Copyright Office's RFI: a broad fair use finding for training (A) combined with weak liability rules (C) & lack of transparency (D) creates a system where infringement can occur at scale...
...with little accountability or recourse for rights holders. Simultaneously challenging the basis of authorship (B) further complicates the assertion and valuation of creative contributions.
Analysis of Public Responses to the RFI on Artificial Intelligence and Copyright
by Gemini Advanced, Deep Research with 2.5 Pro. Warning, LLMs may hallucinate!
Sources:
White House AI Action Plan: U.S. public submits over 10,000 comments
https://files.nitrd.gov/90-fr-9088/90-fr-9088-combined-responses.pdf
I. Introduction
A. Background: The AI and Copyright Nexus
The rapid proliferation and increasing sophistication of artificial intelligence (AI), particularly generative AI capable of producing text, images, music, and code, have brought copyright law to a critical juncture. These technologies raise fundamental questions about the application of established legal principles to novel methods of creation and dissemination. Key issues include the legality of using copyrighted works to train AI models, the potential for copyright protection of AI-generated outputs, the allocation of liability when AI systems produce infringing content, and the appropriate level of transparency regarding AI training data and processes. Recognizing the profound implications of AI for the copyright ecosystem, the U.S. Copyright Office issued a Request for Information (RFI) on Artificial Intelligence and Copyright (published at 90 FR 9088) to solicit public input. This initiative represents a crucial step in gathering diverse perspectives to inform potential policy development, administrative guidance, or legislative action. This report provides a detailed analysis of the public responses submitted pursuant to the RFI, as compiled in the document 90-fr-9088-combined-responses.pdf.
B. Purpose and Scope of the Report
The objective of this report is to deliver a structured and comprehensive analysis of the public comments received in response to the Copyright Office's RFI. It aims to categorize the diverse viewpoints presented, summarize the key arguments, recommendations, and grievances articulated by various stakeholders, identify particularly noteworthy statements, assess the potential implications of certain arguments for rights owners, content owners, creators, and scholarly publishers, and offer strategic recommendations for these groups. The scope of this analysis is confined to the content presented within the aforementioned compilation of RFI responses.
C. Methodology
The analysis was conducted through a comprehensive review of the submitted responses contained within the designated document. A thematic analysis approach was employed to identify recurring arguments, concerns, and proposals across the submissions. Responses were categorized based on apparent stakeholder affiliation (where discernible) and the primary issues addressed. Salient points, including those deemed surprising, controversial, or particularly valuable, were identified and extracted for closer examination. A specific analytical lens was applied to assess arguments from the perspective of rights owners, content owners, creators, and scholarly publishers, focusing on potential challenges and threats to their interests.
D. Structure of the Report
This report is organized into the following chapters:
Chapter II: Landscape of Responses: Clustering and Overall Perspectives:Categorizes respondents and summarizes their positions on core issues like AI training, output copyrightability, liability, and transparency.
Chapter III: Noteworthy Statements: Surprising, Controversial, and Valuable Insights: Highlights specific statements that stand out for their novelty, potential for disagreement, or constructive contribution.
Chapter IV: Implications for Rights Holders: Identifying Potential Challenges and Threats: Analyzes arguments presented by various respondents that could negatively impact the interests of rights owners, content owners, creators, and scholarly publishers.
Chapter V: Strategic Recommendations for Rights Owners, Content Owners, Creators, and Scholarly Publishers: Offers actionable recommendations for these groups based on the analysis in Chapter IV.
Chapter VI: Conclusion: Synthesizes the key findings and reflects on the significance of the RFI responses for the future of AI and copyright.
II. Landscape of Responses: Clustering and Overall Perspectives
The responses to the RFI reveal a complex and often polarized landscape of opinions regarding the intersection of AI and copyright. Stakeholders approach the issues from vastly different perspectives, shaped by their respective roles, interests, and exposure to AI technologies. Analyzing these responses requires understanding both the identity of the commenters and the substance of their arguments on the core issues identified by the Copyright Office.
A. Categorization of Respondents
The submissions represent a wide array of stakeholders, broadly classifiable into several key groups:
Technology Companies/AI Developers: Entities involved in creating, training, and deploying AI systems, ranging from large corporations to smaller startups. Their responses generally advocate for flexibility in copyright law to foster innovation, often favoring interpretations that permit the use of publicly available data for training under doctrines like fair use.
Creative Industries/Creator Groups: Organizations representing authors, artists, musicians, photographers, filmmakers, and other creators, as well as individual creators. These respondents typically emphasize the need to protect creators' rights and ensure compensation for the use of their works in AI training, often arguing against broad fair use exceptions and advocating for strong enforcement mechanisms.
Publishers (including Scholarly Publishers): Companies and associations involved in publishing books, journals, news, music, and other media. Their interests often align closely with creators, focusing on the need for licensing frameworks for AI training data and maintaining copyright protection for valuable content. Scholarly publishers, in particular, may address issues related to text and data mining (TDM) for research versus commercial AI development.
Academic/Research Institutions: Universities, research labs, and academics commenting on the implications of AI for research, education, and scholarship. Views within this group can vary, with some emphasizing the need for access to data for research under fair use or specific exceptions, while others may focus on ethical considerations or the impact on humanities and arts disciplines.
Libraries/Archives: Institutions focused on preservation and access to information. They often advocate for exceptions and limitations to copyright that facilitate their missions, potentially including TDM for research and preservation, and may express concerns about overly restrictive measures hindering access to knowledge.
Public Interest/Advocacy Groups: Non-profit organizations working on issues related to digital rights, free expression, consumer protection, or ethical technology. Their positions can be diverse, sometimes aligning with tech companies on promoting innovation and access, other times aligning with creators on fairness and economic justice, or focusing on broader societal impacts like bias in AI.
Legal Associations/Practitioners: Bar associations, intellectual property law groups, and individual attorneys offering legal analysis and perspectives on how existing doctrines apply or might need adaptation.
Individual Creators/Academics: Submissions from individuals not formally representing larger organizations, offering personal perspectives and experiences.
A summary of general stances observed across these categories is presented below:
Table 1: Respondent Categories and General Stances (Illustrative)
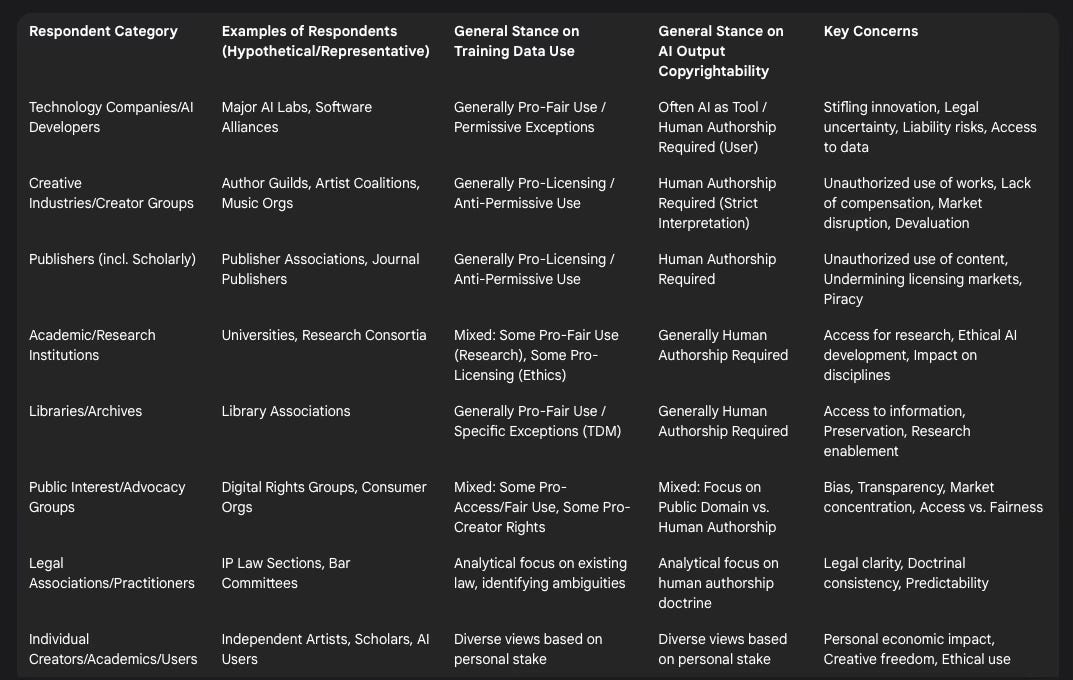
This categorization highlights the fundamental disagreements present in the responses, particularly concerning the use of copyrighted materials for AI training and the conditions under which AI outputs might warrant copyright protection. These differing viewpoints set the stage for the specific arguments raised concerning the core issues.
B. Core Issue 1: Use of Copyrighted Works for AI Training
The analysis reveals that the debate surrounding the ingestion of copyrighted works to train AI models is arguably the most contentious and fundamental issue addressed in the RFI responses. This conflict over the legality and ethics of using training data underpins many subsequent disagreements regarding AI outputs and liability.
1. Arguments for Permissive Use (Fair Use / Exceptions): A significant number of responses, particularly from the technology sector and some academic or library commenters, argue that training AI models on publicly accessible copyrighted works should be permissible under existing copyright law, primarily through the doctrine of fair use, or that new exceptions tailored to AI/TDM are warranted. These arguments often emphasize the transformative nature of AI training, asserting that the purpose is not to reproduce the expressive content of the works but to extract non-expressive statistical patterns and relationships. Proponents frequently draw parallels to previous cases like Authors Guild v. Google, suggesting that creating new functionalities (like search or AI capabilities) from existing works constitutes a transformative use. They argue that requiring licenses for the vast amounts of data needed for state-of-the-art AI is practically impossible and would stifle innovation, particularly for smaller players and researchers. The non-consumptive nature of the analysis (where the model learns patterns rather than storing copies of works) is often cited as favoring fair use.
2. Arguments Against Permissive Use (Infringement / Need for Licensing):Conversely, a large contingent of respondents, predominantly from creative industries, creator groups, and publishers, firmly assert that the unauthorized copying and ingestion of copyrighted works for AI training constitutes massive copyright infringement . They argue that such use directly violates the exclusive rights of reproduction and adaptation held by copyright owners. These groups emphasize the commercial purpose behind most large-scale AI development and the direct economic harm caused by training AI on copyrighted content without permission, particularly when the resulting AI systems generate outputs that compete with the original works in the market. They contend that the scale of the copying involved weighs heavily against fair use and that the value extracted by AI developers rightfully belongs to the creators and rights holders whose works fuel the systems. The argument is often made that allowing such use without compensation devalues creative labor and undermines the economic foundation of creative industries.
3. Proposed Licensing Solutions: Recognizing the tension, some respondents from various camps propose or discuss potential licensing solutions. Suggestions range from voluntary direct licensing between AI developers and rights holders, to the development of collective licensing schemes managed by collective management organizations (CMOs), potentially leveraging existing CMO infrastructure. Others float ideas for tiered licensing based on the type of use or model, or even government-facilitated or mandated licensing systems, although the latter often raises concerns about government rate-setting. The feasibility, scalability, and fairness of any proposed licensing model are subjects of considerable debate within the responses.
The "scale" of data required for AI training emerges as a critical point of contention, interpreted very differently by opposing sides. Technology developers present scale as a justification for fair use due to the impracticality of individual licensing. Rights holders, however, view this scale as indicative of the enormous value being appropriated without authorization, arguing that technological capability should not excuse infringement but rather necessitate the development of scalable licensing solutions. This highlights a fundamental tension regarding whether legal frameworks should adapt primarily to facilitate new technological possibilities or to ensure the continued protection of existing rights within new technological contexts.
C. Core Issue 2: Copyrightability of AI-Generated Outputs
The question of whether, and under what conditions, works generated by AI systems can receive copyright protection is another central theme.
1. Requirement of Human Authorship: The dominant view expressed across many stakeholder groups, including creator organizations, publishers, legal experts, and even some technology companies, is that the U.S. copyright system requires human authorship. These responses emphasize the constitutional and statutory grounding of copyright in promoting human creativity. They argue that AI, as a non-human entity, cannot possess the requisite mental state, intent, or "originality" (as conceived by copyright law) originating from a human mind. Therefore, outputs generated solely by AI without sufficient human creative intervention should not be eligible for copyright protection and may fall into the public domain.
2. AI as a Tool / Human-AI Collaboration: A common perspective, particularly among AI developers and users, is that AI should be viewed as a sophisticated tool, akin to a camera or digital editing software. Under this view, copyright in an AI-assisted work should vest in the human user, provided their contribution meets the threshold for originality. This involves selecting inputs, guiding the AI through prompts, curating outputs, modifying results, and making creative choices. However, considerable uncertainty exists regarding how to define and measure "sufficient human input" or "meaningful human control," leading to calls for the Copyright Office to provide clearer guidance on this standard.
3. Novel Approaches (AI as Author / New Rights): While largely outside the mainstream of current U.S. legal thought, a few responses might touch upon more radical possibilities, such as contemplating scenarios where AI could be recognized as an author or exploring the creation of sui generis (new or distinct) rights for AI-generated outputs. These discussions are often more theoretical or forward-looking, acknowledging the significant legal hurdles under the current framework but perhaps anticipating future debates.
D. Core Issue 3: Liability for Infringement Involving AI
Determining who is liable when AI systems generate outputs that infringe existing copyrights is a critical concern for nearly all respondents, though their proposed solutions differ markedly.
1. Liability of AI Developers/Deployers: Rights holder groups strongly advocate for holding AI developers and deployers liable for infringements caused by their systems. They argue that these entities create the systems, choose the training data (often including infringing material), control the AI's functionalities, and profit from its deployment. Therefore, they are best positioned to implement safeguards and should bear responsibility when their products cause harm. Theories of direct, contributory, and vicarious liability are often discussed in this context.
2. Liability of AI Users: Some discussion revolves around the potential liability of end-users who prompt AI systems to generate infringing content. However, many argue that focusing solely on users is impractical and unfair, especially when users may not know the AI was trained on infringing data or that the output is substantially similar to a protected work. The primary focus for liability tends to remain on the commercial entities behind the AI.
3. Safe Harbors and Immunities: Technology companies and platforms hosting AI tools often argue for the application or adaptation of existing safe harbor provisions, such as Section 512 of the Digital Millennium Copyright Act (DMCA), to AI contexts. They might contend that the scale and unpredictability of AI outputs necessitate protection from overwhelming liability, similar to how online platforms are shielded regarding user-generated content. Rights holders generally oppose broad immunities, arguing they would unfairly shift the burden of policing infringement onto creators and incentivize irresponsible AI development.
E. Core Issue 4: Transparency and Record-Keeping
The issue of transparency, particularly regarding the data used to train AI models, is another significant point of division.
1. Calls for Transparency: Creator groups, publishers, and some public interest advocates strongly call for greater transparency from AI developers. They argue that knowing which copyrighted works were included in training datasets is essential for rights holders to identify potential infringements, negotiate licenses, and enforce their rights. Transparency is also framed as crucial for auditing AI systems for bias and ensuring accountability. Proposals range from full public disclosure of datasets to more limited summaries, access mechanisms for verification, or mandatory record-keeping.
2. Arguments Against Transparency: AI developers and some researchers raise concerns about mandatory transparency requirements. They cite the protection of trade secrets (the composition of datasets and model weights can be highly valuable proprietary information), the immense computational and logistical burden of tracking and disclosing potentially billions of data points, privacy concerns related to data sources, and the argument that disclosure might not be technically feasible or meaningful for models trained on vast, heterogeneous web-scraped data.
F. Synthesis: Overall Suggestions, Recommendations, and Grievances
Across the diverse responses, several overarching themes emerge. There is widespread agreement that the intersection of AI and copyright presents significant challenges and that some form of clarification or guidance from the Copyright Office or Congress is needed to reduce legal uncertainty. However, consensus breaks down sharply regarding the substance of that clarification.
Frequent Recommendations: Calls for Copyright Office guidance on human authorship standards, clarification of fair use applicability to AI training, development of industry best practices, and exploration of market-based licensing solutions are common.
Common Grievances: Rights holders express grievances about unauthorized use and lack of compensation, fearing economic disruption and devaluation of creativity. AI developers express grievances about legal ambiguity hindering innovation and the perceived impracticality of existing licensing models at scale. Both sides lament the lack of clear rules of the road.
Areas of Consensus and Divergence: While most agree clarity is needed, fundamental disagreement persists on whether AI training on copyrighted works without permission is legally permissible (fair use vs. infringement). This remains the central fault line. There is more potential convergence around the requirement of human authorship for copyrightability, though defining the threshold remains challenging. Liability and transparency remain highly contested.
The analysis suggests that stakeholder positions are deeply entrenched, particularly on the training data issue. Finding common ground will require navigating complex legal doctrines, economic interests, and ethical considerations. The potential for alliances is also apparent; for instance, creators and publishers share strong interests in licensing and enforcement, while technology firms and some research communities may align on advocating for permissive data use policies. Public interest groups remain a potentially pivotal voice, capable of aligning with different sides depending on whether access and innovation or fairness and creator protection are prioritized in specific contexts.
III. Noteworthy Statements: Surprising, Controversial, and Valuable Insights
Amidst the volume of responses articulating predictable stakeholder positions, certain statements stand out for their potential to surprise, provoke controversy, or offer particularly valuable contributions to the ongoing debate. These noteworthy points often push the boundaries of conventional thinking or offer concrete pathways forward.
A. Surprising Assertions
Some submissions contained assertions that were unexpected, either due to their departure from typical arguments within a stakeholder group or their novel interpretation of law or technology.
One potential area for surprise might involve arguments asserting that the technical process of AI training inherently avoids copyright infringement, perhaps by claiming that only non-expressive statistical abstractions are stored, not copies of the works themselves, thus bypassing the reproduction right entirely. Such a technologically deterministic argument, if presented, would be surprising in its attempt to preempt legal analysis through technical description.
Another surprising element could be unexpected concessions. For example, a submission from a major technology company acknowledging the need for someform of revenue sharing or contribution to a creator fund, even while maintaining a strong fair use stance for training, would represent a significant departure from maximalist positions.
Conversely, a statement from a traditional rights holder group suggesting that certain limited forms of AI training for non-commercial research purposes couldqualify as fair use, while maintaining a hard line on commercial training, might also be surprising in its nuance.
Submissions arguing for a radical overhaul of copyright law itself, deeming it fundamentally ill-suited for the age of AI, move beyond calls for clarification and represent a more surprising, systemic critique than is typical.
These surprising statements are notable because they challenge assumptions about stakeholder alignment and the perceived limits of the debate, potentially opening new avenues for discussion or highlighting less obvious points of friction.
B. Controversial Proposals
Controversy arises from proposals that directly challenge deeply held principles or interests of significant stakeholder groups, virtually guaranteeing strong opposition.
Perhaps the most controversial proposals involve the core tenets of copyright. Any explicit call to eliminate or significantly dilute the human authorship requirement for copyright protection would be intensely controversial, striking at the foundation of U.S. copyright law and provoking fierce resistance from creators, publishers, and likely the Copyright Office itself.
Arguments demanding that all data scraping and ingestion for AI training, regardless of the source material's copyright status or the commercial nature of the AI, be deemed per se fair use would be highly controversial. This position dismisses rights holders' concerns entirely and advocates for a near-total appropriation of value.
Proposals for compulsory licensing schemes with statutorily set royalty rates perceived as extremely low by rights holders would undoubtedly generate controversy, seen as undermining fair market negotiations and devaluing creative works.
On the liability front, suggestions that AI developers should enjoy near-complete immunity from copyright infringement liability, perhaps through expansive safe harbors far exceeding current interpretations of DMCA Section 512, would be met with strong opposition from rights holders, who would see it as leaving them without effective remedies.
These controversial proposals often represent the maximalist positions within the debate. While unlikely to be adopted wholesale, they serve to define the extreme ends of the policy spectrum, thereby framing the space within which more moderate compromises might eventually be negotiated. Understanding these poles is crucial for mapping the political and legal battlefield.
C. Valuable Contributions
Beyond the surprising and controversial, many responses offer valuable insights, constructive suggestions, and nuanced analyses that could genuinely contribute to resolving the complex challenges at hand.
Valuable contributions often lie in proposing specific, workable mechanisms. For instance, a detailed framework outlining concrete criteria for assessing "meaningful human control" or "sufficient human authorship" in AI-assisted works could provide practical guidance for creators, companies, and the Copyright Office. Such frameworks move beyond abstract principles to offer operational tests.
Innovative proposals for tiered or usage-based licensing models, potentially incorporating technological solutions for tracking and reporting, could offer a path forward on the contentious training data issue, addressing both the need for access and the demand for compensation.
Economic analyses that provide credible data on the actual or projected impact of AI on specific creative markets offer valuable evidence for policymakers. Quantifying the potential harm of unlicensed training or the potential benefits of licensing models strengthens the arguments of respective parties.
Technical insights into potential methods for watermarking datasets, tracking data provenance, or building AI systems with inherent respect for copyright constraints can be highly valuable, suggesting that technological solutions might mitigate some legal conflicts.
Nuanced legal analyses that carefully distinguish between different types of AI (e.g., discriminative vs. generative), different types of uses (e.g., research vs. commercial deployment), and different types of copyrighted works could also be valuable. Such analyses resist one-size-fits-all solutions and point towards more tailored approaches.
These valuable contributions often distinguish themselves by moving beyond ideological pronouncements to offer practical solutions, empirical evidence, or refined analytical frameworks. They demonstrate that progress may depend less on winning broad arguments and more on developing specific, context-sensitive rules and mechanisms that balance competing interests.
IV. Implications for Rights Holders: Identifying Potential Challenges and Threats
From the perspective of rights owners, content owners, creators, and scholarly publishers, several arguments and proposals presented in the RFI responses pose significant potential challenges and threats to their interests, economic viability, and legal rights. This chapter analyzes these specific points of concern.
A. Erosion of Exclusive Rights via Expansive Fair Use
The most direct threat perceived by rights holders stems from arguments advocating for broad interpretations of fair use to cover the ingestion of copyrighted works for AI training.
Submissions asserting that training is a "transformative use" because it serves a different purpose (statistical analysis) than the original work (expression) , or that it is a "non-expressive use", directly challenge the core exclusive rights of reproduction and adaptation. If accepted, such interpretations could effectively eliminate the ability of rights holders to control or demand compensation for this economically significant use of their works.
The potential economic impact is substantial. Unlicensed training could lead to the devaluation of existing creative works and archives, as their value for data extraction is appropriated without payment. It could severely undermine existing and potential licensing markets for TDM and AI training, reducing revenue streams that support creation. Furthermore, it could diminish the incentive for creating original, high-quality content if that content can be freely used to train systems that may ultimately compete with or replace human creators. The argument that scale makes licensing impossible is seen not as a defense, but as an admission of the massive scope of unauthorized value transfer.
B. Challenges to Authorship and Ownership
Arguments that minimize the requirement of human authorship or suggest that AI-generated outputs should automatically enter the public domain pose a fundamental threat to the value proposition of creative industries.
Proposals questioning the necessity of human authorship, even if presented as theoretical explorations , could, if they gain traction, erode the legal basis upon which creators and publishers establish ownership and control over creative works. If purely AI-generated works lack copyright, it creates uncertainty and potential market disruption.
More immediately, the proliferation of AI capable of mimicking the style of specific human creators raises concerns. If AI outputs generated in the style of an artist are not protected by copyright (due to lack of human authorship) and the underlying AI was trained on that artist's work without permission, the artist suffers economic harm and potential reputational damage without adequate recourse under current copyright law alone (though other legal avenues like right of publicity might apply). The value of human originality and creative expression risks being diluted in a market flooded with easily generated synthetic content.
C. Weakening Liability Frameworks
Proposals aimed at shielding AI developers and platforms from liability for copyright infringement represent a significant threat to rights holders' ability to enforce their rights.
Calls to extend DMCA-like safe harbors to generative AI platforms or to create bespoke immunities for AI developers could leave rights holders bearing the sole, often overwhelming, burden of monitoring vast amounts of AI-generated content for infringement and pursuing individual users. This effectively externalizes the cost of infringement prevention onto creators and could render enforcement impractical, particularly for independent creators and smaller publishers.
If developers are shielded from liability even when their systems have been trained on infringing data and are designed in ways that predictably produce infringing outputs, it removes a key incentive for them to invest in preventative measures, such as licensing data or implementing robust content filters. This could lead to a situation where platforms profit from tools that facilitate infringement at scale, while rights holders are left with few effective remedies.
D. Resistance to Transparency
Arguments against transparency regarding AI training data directly impede the ability of rights holders to protect and manage their copyrights in the AI era.
The pushback against transparency from AI developers, often citing trade secrets or technical infeasibility, creates a "black box" problem. Rights holders cannot easily determine if their specific works were used to train a particular model, making it difficult to detect infringement, challenge fair use claims, or negotiate appropriate licenses.
This lack of transparency can mask the true extent of reliance on copyrighted materials, potentially enabling widespread, undetected infringement. It also creates an information asymmetry that disadvantages rights holders in licensing negotiations, as they lack visibility into how and how much their content is being used. Without transparency, accountability for infringement becomes significantly harder to establish.
E. Downplaying Economic Harm
Arguments that dismiss or minimize the potential economic harm AI poses to creative industries represent a rhetorical threat, potentially influencing policymakers to undervalue the concerns of creators and rights holders.
Statements suggesting AI will primarily augment human creativity rather than replace it, or that claims of economic harm are merely speculative and should not impede technological progress, attempt to frame rights holders' concerns as exaggerated or protectionist.
This narrative ignores the lived realities of creators already facing competition from AI-generated content produced at low cost and trained on their own work without compensation. It also downplays the potential for significant disruption in creative labor markets and the systemic impact on industries reliant on copyright licensing. Allowing this narrative to dominate risks marginalizing legitimate economic concerns in policy deliberations.
These threats are often interconnected. For example, a broad fair use finding for training (A) combined with weak liability rules (C) and lack of transparency (D) creates a system where infringement can occur at scale with little accountability or recourse for rights holders. Simultaneously challenging the basis of authorship (B) further complicates the assertion and valuation of creative contributions. This constellation of arguments presents a systemic challenge to the existing copyright framework from the perspective of those who rely on it for their livelihoods and business models. Furthermore, the persistent framing of the debate as "innovation vs. rights" strategically disadvantages rights holders by casting their legitimate demands for consent and compensation as obstacles to technological advancement, obscuring the role of copyright itself as an engine of creativity and economic activity that provides the very content AI systems utilize.
V. Strategic Recommendations for Rights Owners, Content Owners, Creators, and Scholarly Publishers
Based on the analysis of potential challenges and threats identified in the RFI responses, the following strategic recommendations are offered for consideration by rights owners, content owners, creators, and scholarly publishers as they navigate the evolving landscape of AI and copyright. These recommendations focus on proactive engagement, evidence-based advocacy, and coalition building.
A. Countering Expansive Fair Use Arguments
1. Emphasize Market Harm: Develop and vigorously present concrete evidence demonstrating the actual and potential negative impact of unlicensed AI training on markets for copyrighted works. This includes commissioning and disseminating credible economic studies quantifying the usurpation of licensing opportunities (addressing the crucial fourth fair use factor), the devaluation of creative assets, and the impact on creator incomes.
2. Distinguish from Precedents: Clearly articulate the specific ways in which generative AI training differs from prior fair use precedents like search engine indexing (Authors Guild v. Google) or non-consumptive TDM for pure research. Focus on the scale of copying, the commercial nature of most generative AI development, and the fact that generative outputs often directly compete with the source works in the market, unlike search snippets or research findings.
3. Highlight the Nature of the Use: Consistently argue that the systematic, often commercial, copying of entire works (or substantial portions) for training weighs heavily against fair use under the first (purpose and character) and third (amount and substantiality) factors. Frame the use not as passive analysis but as active exploitation of creative expression to build commercial products.
B. Advocating for Licensing Frameworks
1. Propose Viable Models: Move beyond simply demanding licenses to actively developing and proposing practical, scalable licensing solutions. This could involve strengthening existing CMOs, exploring API-based permissioning systems, developing standardized license terms for AI training, or supporting consortia approaches. Demonstrating that workable licensing is possible directly counters the "impossibility" argument used to justify fair use.
2. Frame Licensing as Pro-Innovation: Position licensing not as an obstacle, but as a necessary and sustainable foundation for ethical AI development. Argue that fair compensation incentivizes the continued creation of diverse, high-quality human expression – the very data that fuels AI progress – fostering a mutually beneficial ecosystem.
3. Collaborate on Standards: Engage with other rights holder groups, and potentially technology partners willing to license, to develop industry standards for AI licensing terms, technical implementation (e.g., metadata, watermarking), and reporting mechanisms. Standardization can reduce transaction costs and facilitate broader adoption.
C. Defending Human Authorship and Value
1. Reinforce Legal Doctrine: Mount a strong defense of the human authorship requirement as a cornerstone of U.S. copyright law, rooted in the Constitution and statute. Emphasize its importance in incentivizing human creativity, ensuring accountability, and maintaining the integrity of the copyright system.
2. Promote "Human-in-the-Loop" Standards: Advocate for clear, practical guidance from the Copyright Office and courts on the level of human creative input and control necessary to qualify for copyright protection in AI-assisted works. Support standards that require meaningful, demonstrable creative intervention, not merely mechanical operation of the AI tool.
3. Articulate the Value of Human Creation: Engage in public discourse and policy advocacy that highlights the unique cultural, ethical, and economic value of human creativity. Emphasize aspects like lived experience, emotional depth, nuance, and accountability that AI cannot replicate, reinforcing the societal importance of protecting and rewarding human creators.
D. Shaping Liability Rules
1. Advocate for Developer/Deployer Responsibility: Argue forcefully that the primary legal responsibility for infringements caused by AI systems should lie with the entities that develop, train, deploy, and profit from them. Emphasize their capacity to implement technical safeguards, vet training data, and control system outputs. Advocate for clear lines of direct and secondary liability.
2. Resist Overly Broad Safe Harbors: Actively oppose the creation of new, bespoke immunities for AI developers or the uncritical extension of existing safe harbors like DMCA Section 512 to generative AI outputs. Argue that such shields would improperly subsidize AI development at the expense of creators and undermine copyright enforcement.
3. Support Proportionate User Liability: While acknowledging that users can misuse AI, advocate for liability frameworks that focus primarily on the commercial actors placing powerful generative tools into the market, rather than placing the main enforcement burden on individual end-users.
E. Championing Meaningful Transparency
1. Advocate for Mandatory Disclosure/Record-Keeping: Push for regulatory or legislative measures requiring AI developers to maintain accurate records of the copyrighted works ingested during training and to provide meaningful transparency about dataset composition. This could involve tiered disclosure mechanisms that allow rights verification while addressing legitimate trade secret concerns.
2. Support Technical Solutions: Encourage and support the development and adoption of technologies that facilitate data provenance tracking, such as robust metadata standards, content watermarking, or other methods that help identify the use of copyrighted material in AI training and outputs.
3. Frame Transparency as Trust-Building: Argue that transparency is not an impediment but a prerequisite for building trust between AI developers, rights holders, and the public. Position it as essential for ethical AI development, fair market negotiations, and effective copyright management.
F. Strategic Communication and Coalition Building
1. Reframe the Narrative: Proactively counter the simplistic "innovation vs. rights" dichotomy. Frame copyright protection and licensing not as barriers, but as foundational elements of the creative economy that provide the essential fuel for AI systems and contribute to cultural richness. Emphasize that "ethical AI" necessitates respect for creators' rights and fair compensation.
2. Build Broad Coalitions: Seek alliances beyond traditional rights holder groups. Collaborate with organizations and individuals concerned with ethical AI, algorithmic accountability, fair labor practices, and potentially smaller technology companies or researchers who may also be disadvantaged by the dominance of large AI labs relying on massive, unlicensed datasets. Unified voices are stronger.
3. Engage Proactively in Policy Debates: Maintain a consistent and well-resourced presence in all relevant policy forums, including Copyright Office proceedings, Congressional hearings, executive branch initiatives, and international discussions. Provide expert testimony, submit detailed comments, and engage directly with policymakers to ensure rights holders' perspectives are clearly understood and considered.
Implementing these recommendations requires a shift from a primarily reactive posture—defending against unfavorable proposals—to a proactive one focused on shaping the legal and market environment through constructive solutions, compelling evidence, and strategic communication. The effectiveness of this advocacy will be significantly enhanced if rights holder groups can present unified positions backed by robust legal reasoning and credible economic data. Internal coordination and investment in evidence generation are therefore crucial for navigating the complex and high-stakes policy debates surrounding AI and copyright.
VI. Conclusion
A. Synthesis of Key Findings
The public responses to the U.S. Copyright Office's RFI on Artificial Intelligence and Copyright reveal a landscape marked by profound disagreement on fundamental issues, yet united by a recognition that AI presents significant challenges to the existing copyright framework. The analysis highlights several key points:
Deep Divisions: Sharp divergences exist, particularly regarding the legality of using copyrighted works for AI training without permission (fair use vs. infringement/licensing), the appropriate scope of liability for AI-generated infringements, and the necessary level of transparency concerning training data.
Areas of Relative Convergence: There appears to be broader (though not universal) agreement on the principle that copyright protection requires human authorship, although defining the threshold for sufficient human involvement in AI-assisted creation remains a critical challenge.
Identified Threats to Rights Holders: From the perspective of creators, publishers, and other rights owners, significant threats arise from arguments favoring expansive fair use for training, challenges to human authorship, proposals for weak liability shields for AI developers, and resistance to transparency, all of which could undermine their economic rights and ability to control their works.
Call for Clarity: Across the spectrum, stakeholders expressed a need for greater legal clarity and guidance to navigate the uncertainties posed by AI.
B. Significance for the AI and Copyright Landscape
The submissions analyzed in this report represent a critical repository of stakeholder perspectives that will inevitably shape the ongoing policy debate in the United States and likely influence international discussions. They underscore the complexity of adapting copyright law—designed primarily for human creators and traditional forms of copying—to the realities of machine learning and generative AI. The stakes are exceptionally high, involving the future of creative industries, the trajectory of AI innovation, and the fundamental balance between incentivizing new technologies and protecting the rights that underpin cultural production.
C. Concluding Remarks
The path forward remains uncertain. Potential developments include further guidance or rulemaking from the Copyright Office, targeted legislative interventions by Congress, landmark court decisions arising from ongoing litigation, or the evolution of market-driven licensing solutions and industry best practices. What is clear is that the issues raised in the RFI responses demand careful, nuanced consideration. Balancing the immense potential of AI with the enduring principles of copyright law requires a dialogue that acknowledges the legitimate interests and concerns of all stakeholders. Achieving a sustainable equilibrium—one that fosters both innovation and creativity—will necessitate not only legal acumen but also a willingness to engage in constructive compromise and potentially develop novel solutions tailored to the unique challenges of the AI era. The responses gathered by the Copyright Office provide an essential, albeit contentious, foundation for this critical undertaking.
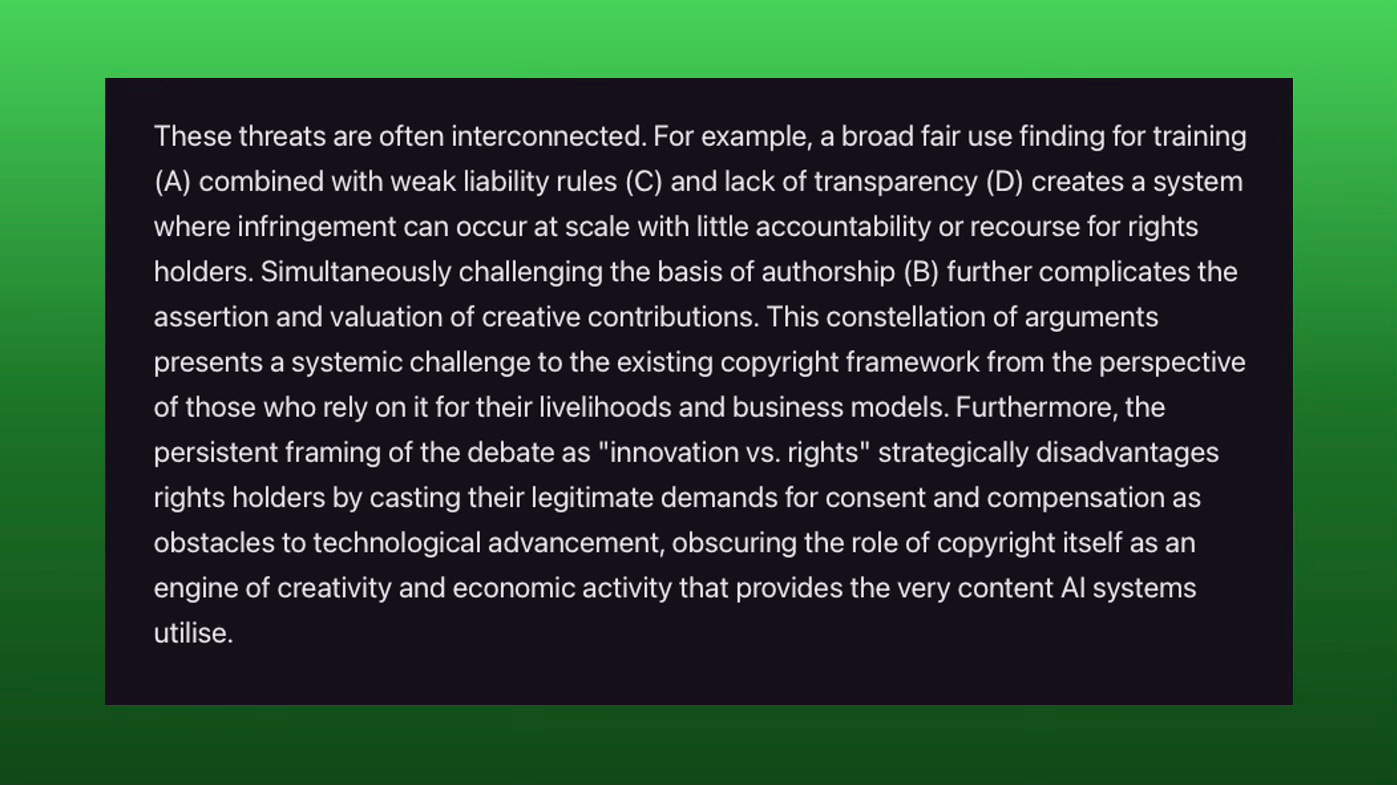