- Pascal's Chatbot Q&As
- Posts
- EUIPO Report: The Development of Generative Artificial Intelligence from a Copyright Perspective: How GenAI systems interact with copyright law.
EUIPO Report: The Development of Generative Artificial Intelligence from a Copyright Perspective: How GenAI systems interact with copyright law.
The key messages are clear: Transparency is critical: GenAI systems must disclose their use of copyrighted material, and outputs must be traceable. Public institutions must step in.
EUIPO Report: The Development of Generative Artificial Intelligence from a Copyright Perspective.
Analyzed by ChatGPT-4o
📘 Introduction
In May 2025, the European Union Intellectual Property Office (EUIPO) published a landmark report titled “The Development of Generative Artificial Intelligence from a Copyright Perspective.” This in-depth study explores one of the most pressing challenges of our time: how to reconcile the explosive growth of generative AI (GenAI) systems with the foundational principles of copyright law. At a moment when AI tools are reshaping how we create, consume, and commercialize content, the report provides a much-needed technical, legal, and economic roadmap for policymakers, rights holders, and developers alike.
The report's primary goal is to clarify how GenAI systems interact with copyright law, focusing on both input (how copyrighted works are used to train AI models) and output (how AI-generated content may infringe, reproduce, or reshape existing intellectual property). It outlines the EU’s current legal framework—including the Copyright in the Digital Single Market (CDSM) Directive and the newly adopted AI Act—and maps how these instruments address the use of copyrighted content in AI development. The study also surveys existing and emerging mechanisms to support rights reservation (opt-outs), licensing markets, and content provenance.
The key messages are clear:
Transparency is critical: GenAI systems must disclose their use of copyrighted material, and outputs must be traceable.
Rights reservation must be enforceable: A patchwork of legal and technical opt-out measures currently exists, but lacks standardisation and enforceability.
Licensing is not only possible—it’s emerging: Markets for AI training data are developing rapidly, with new economic models and infrastructure to support fair compensation.
Public institutions must step in: There is an urgent need for central coordination, institutional support, and federated registries to empower rights holders, especially smaller creators and publishers.
This report is not merely descriptive—it is prescriptive. It lays out a future in which copyright and AI can coexist, provided that legal clarity, technical interoperability, and economic fairness are prioritized. In doing so, it offers one of the most comprehensive efforts yet to bridge the gap between innovation and rights protection in the AI era.
Here is a detailed analysis of the report “The Development of Generative Artificial Intelligence from a Copyright Perspective”, structured into key insights, sector-specific implications, stakeholder recommendations, and an evaluative conclusion.
🔍 Most Surprising, Controversial, and Valuable Findings
Surprising
High levels of memorisation persist in GenAI models, despite deduplication and filters: Studies (e.g., Ippolito 2023) show that models like Copilot still generate content closely resembling training data—even with mitigation mechanisms like prompt rewriting and filtering.
Troveo monetized ~1 million hours of UGC content, 25% of which comes from creators on TikTok, Instagram, and YouTube, showing an unexpected formalization of informal creator economies into AI training datasets.
OpenAI’s ‘Media Manager’ tool, aimed at launching in 2025, claims to offer machine-readable copyright alignment for multiple content types (text, image, audio, video), yet its actual opt-out criteria and scope remain undisclosed.
Controversial
Use of antagonistic and deceptive tactics by rights holders, including data poisoning and ‘honeypot’ files to thwart AI crawlers, reflects deep mistrust and escalating conflict between rights holders and AI developers.
Direct licensing deals between OpenAI, Google, and platforms like Reddit and Stack Overflow involve mostly user-generated content—raising questions about user consent and rights enforcement on platforms that operate under murky ownership dynamics.
Regulatory silence or ambiguity on whether opt-outs based on location (e.g., URL-based) or content ID will be required or respected under forthcoming frameworks, which could lead to chaos and non-compliance.
Valuable
Valunode's Open Rights Data Exchange (ORDE) is an EU-backed initiative creating a blockchain-based, verifiable registry for rights declarations, enabling tokenization, content identification, and secure licensing infrastructure.
Fairly Trained Certification provides an independent audit framework for GenAI developers to prove use of “copyright-safe” data, a vital transparency step, especially for smaller developers without reputational capital.
Perplexity AI’s publisher program introduces dynamic revenue-sharing and bundling of AI access with publisher subscriptions, suggesting a potentially replicable model for other RAG-based systems.
📚 Statements Beneficial for Content Creators, Rights Owners, and Scholarly Publishers
Rights reservation (opt-out) under Article 4 of the EU CDSM Directivebecomes the primary mechanism enabling rights holders to negotiate licenses for commercial TDM, establishing the legal gateway for monetization.
Federated databases supported by IP offices may help small publishers manage opt-outs and licensing efficiently and transparently, bridging the gap between creators and AI companies.
Emergence of technical licensing ‘counterparts’ in agreements (e.g., APIs, RAG tools) allows content owners not just to monetise access but to gain capabilities enhancing user experience and research applications.
User-generated content creators now have monetization routes via aggregation platforms like Troveo or platform-level deals (e.g., TikTok/Reddit licensing), shifting power from intermediaries back to creators.
Institutional support for solution discoverability (e.g., public repositories of opt-out tools, webinars, crawler ID databases) reduces asymmetries between small rights holders and large GenAI firms.
Media Manager and rights alignment tools represent a potential shift from reactive scraping deterrence to proactive content governance.
✅ Recommendations for Stakeholders
For AI Developers
Adopt transparent licensing models and proactively engage with registry systems (e.g., Valunode, Fairly Trained).
Integrate dual-purpose technologies that support both input rights compliance and output transparency (e.g., watermarking, content provenance).
Collaborate with public institutions to align with standardised opt-out and detection protocols to avoid regulatory fragmentation.
For Regulators and Public Institutions
Support federated registries and standardised APIs for opt-out and licensing communications.
Establish monitoring and reporting mechanisms for AI bot activity and crawler compliance (e.g., REP agent identifier registries).
Expand funding and training support for rights holder organisations and CMOs to navigate technical licensing environments.
For Rights Holders and Publishers
Engage in collective licensing schemes but insist on voluntary participation to maintain rights flexibility.
Experiment with bundled licensing (e.g., API access + data rights) for greater negotiation leverage.
Collaborate with solution providers to deploy watermarking, fingerprinting, and provenance tagging in line with legal recognition.
🎯 Is This a Good Development? Why?
Yes—with qualifications.
The report reflects a maturing ecosystem where generative AI is no longer “wild-scrape and hope,” but is slowly pivoting toward structured engagement with rights holders. The introduction of technical opt-out mechanisms, emerging marketplaces, and content registry infrastructures signal a shift toward copyright-aware AI governance.
What makes this a good development is:
The recognition of creator autonomy, even amid high-capital AI development.
Movement toward incentive alignment via revenue-sharing, APIs, and certification models.
A pluralistic approach that accommodates commercial, user-generated, and academic content types.
However, risks remain if standards are fragmented, or if enforcement and implementation don’t keep pace with model deployment. The value of this development ultimately depends on execution—especially the willingness of AI developers to adopt licensing-by-default, and the capacity of institutions to mediate this ecosystem fairly.
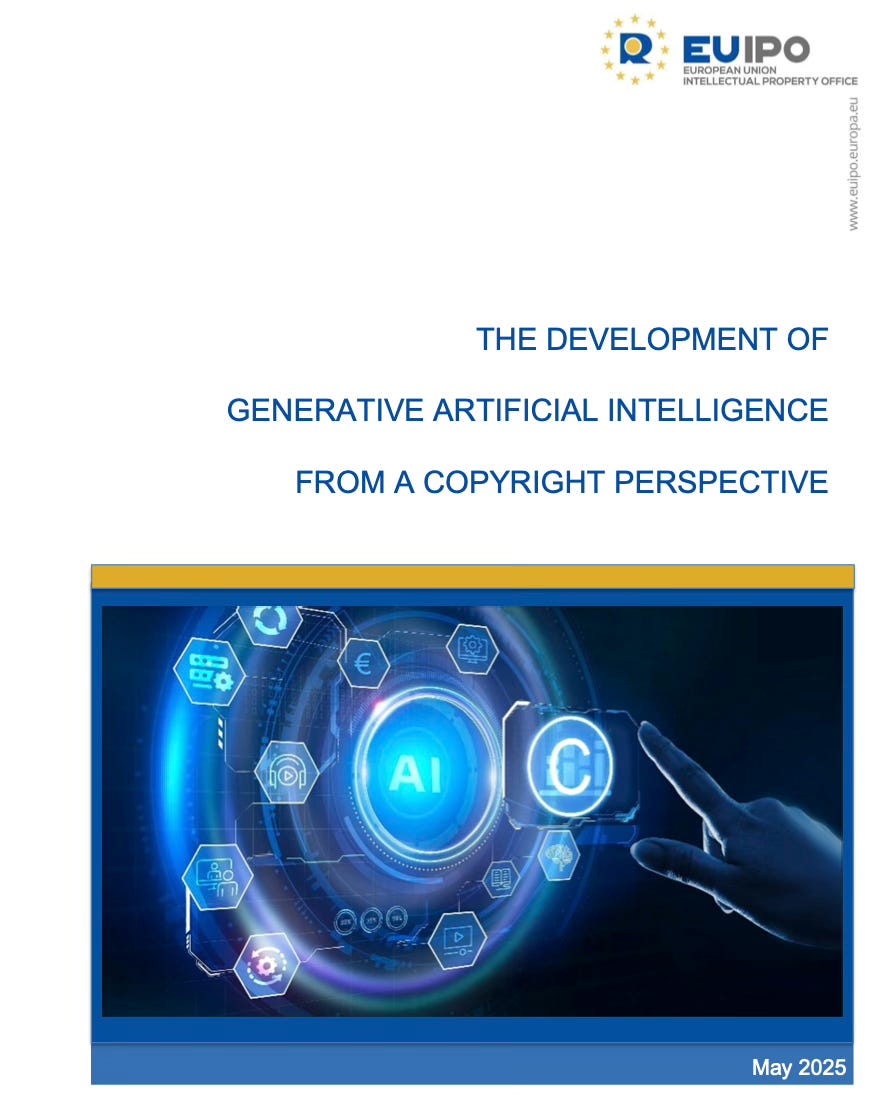