- Pascal's Chatbot Q&As
- Posts
- Claude: From analyzing your Substack posts, I can identify several distinct categories of AI promises, separating them into those backed by evidence and technical feasibility...
Claude: From analyzing your Substack posts, I can identify several distinct categories of AI promises, separating them into those backed by evidence and technical feasibility...
...versus those that appear more aspirational or unrealistic. Some promises about AI seem to cross into what we might call "techno-optimism" or even "digital theology".
Asking Claude: When analysing my Substack posts, which promises related to AI are factual and likely to materialise and which promises are perhaps more 'religious' in nature and will probably never come about?
Claude’s answer:
From analyzing your Substack posts, I can identify several distinct categories of AI promises, separating them into those backed by evidence and technical feasibility versus those that appear more aspirational or unrealistic. Let me break this down:
Promises Likely to Materialize
In the realm of narrow, task-specific AI capabilities, several promises show clear paths to implementation:
Enhanced Data Analysis and Pattern Recognition
AI systems are already demonstrating real capabilities in processing large datasets and identifying patterns. This builds on existing statistical and computational methods, just at a larger scale and with more sophisticated algorithms. The progress here follows a clear technical trajectory we can understand and predict.
For example, in medical imaging, AI systems can already detect certain conditions with high accuracy. This capability will continue to improve because it relies on well-understood pattern recognition principles applied to specific, bounded problems.
Automation of Routine Tasks
We're seeing concrete evidence of AI successfully automating repetitive, rule-based tasks. This includes document processing, basic customer service interactions, and data entry. These applications work because they operate within well-defined parameters and don't require genuine understanding or creativity.
Take legal document review - AI can effectively identify standard clauses and flag potential issues because this task relies on pattern matching and classification, areas where current AI excels.
Language Processing Improvements
While perfect language understanding remains distant, we can expect continued improvements in specific language tasks like translation, summarization, and information extraction. These advances are grounded in measurable metrics and clear technical paths forward.
"Religious" or Overly Optimistic Promises
On the other hand, some promises about AI seem to cross into what we might call "techno-optimism" or even "digital theology":
Artificial General Intelligence (AGI)
The promise of machines that can truly think and understand like humans remains highly speculative. Despite impressive language models, we have no clear path to bridging the gap between pattern recognition and genuine understanding. The complexity of human consciousness and intelligence suggests this goal may be fundamentally different from our current approach to AI.
Current language models, despite their impressive outputs, still operate through sophisticated pattern matching rather than true understanding. Claims about imminent AGI often ignore this fundamental limitation.
Perfect Decision Making
Some proponents suggest AI will eventually make perfectly objective or unbiased decisions. This ignores the inherent limitations of training data and the philosophical complexity of what constitutes "unbiased" decision-making. All data reflects human choices and societal biases, making truly neutral AI an unrealistic goal.
Technological Singularity
The idea that AI will reach a point of runaway self-improvement, leading to superintelligence, relies more on faith than evidence. It assumes capabilities we don't yet understand how to create and ignores fundamental limitations in computational systems.
Why The Distinction Matters
Understanding this separation helps us make better decisions about AI development and deployment:
Technical Reality vs. Speculation
When evaluating AI projects or investments, focusing on concrete, demonstrable capabilities rather than speculative promises leads to better outcomes. For instance, a project to improve medical diagnosis through image analysis is more likely to succeed than one promising to "solve healthcare" through AI.
Resource Allocation
Organizations need to direct resources toward achievable goals rather than chasing unrealistic promises. This means investing in proven applications like process automation while maintaining skepticism about grand claims of human-level AI.
Risk Assessment
Understanding what AI can and cannot realistically achieve helps in evaluating both opportunities and risks. Many feared scenarios about AI depend on capabilities that may not be technically possible, while real risks (like bias in training data) often get less attention.
The Path Forward
To make the most of AI's potential while avoiding unrealistic expectations:
Focus on Specific Problems
Success comes from applying AI to well-defined problems where we can measure progress and understand limitations. This means breaking down large challenges into manageable pieces with clear success criteria.
Maintain Realistic Expectations
Acknowledge that many complex human tasks require understanding and judgment that current AI approaches cannot replicate. This helps avoid overreliance on AI in situations where it's not appropriate.
Balance Optimism with Skepticism
While remaining open to AI's potential, maintain healthy skepticism about grandiose claims. Look for concrete evidence and clear mechanisms of action rather than accepting promises at face value.

11 SEPTEMBER 2024
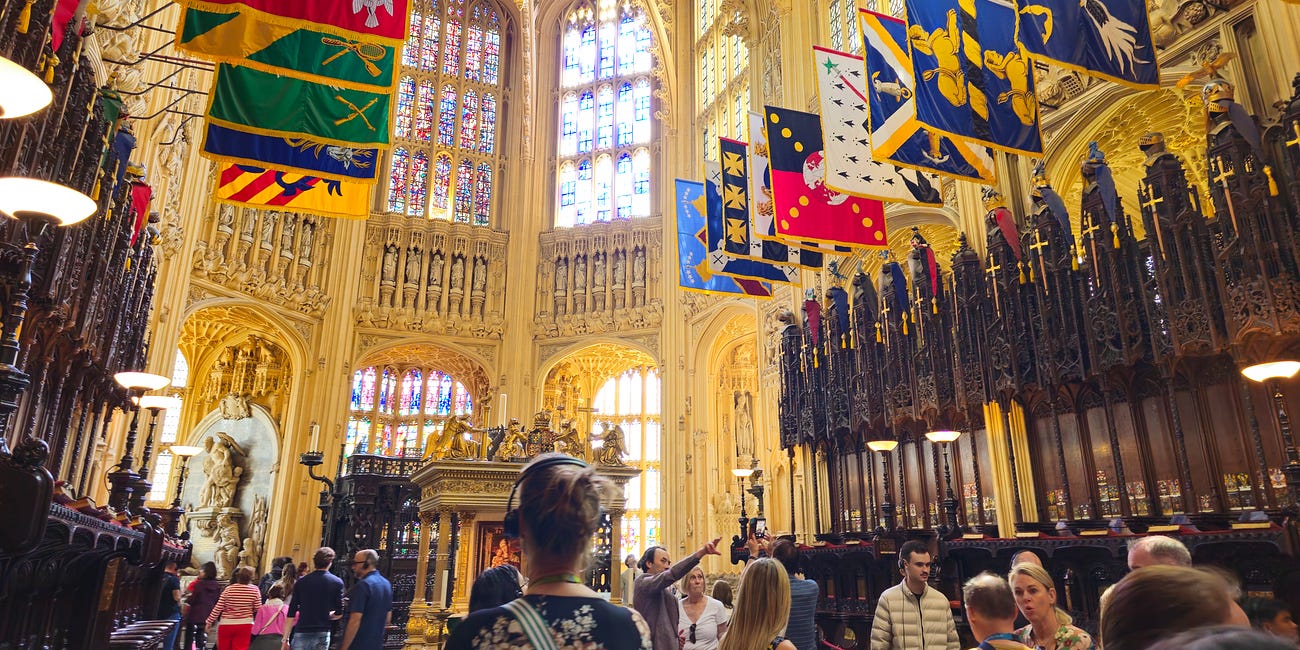
·
1 JAN
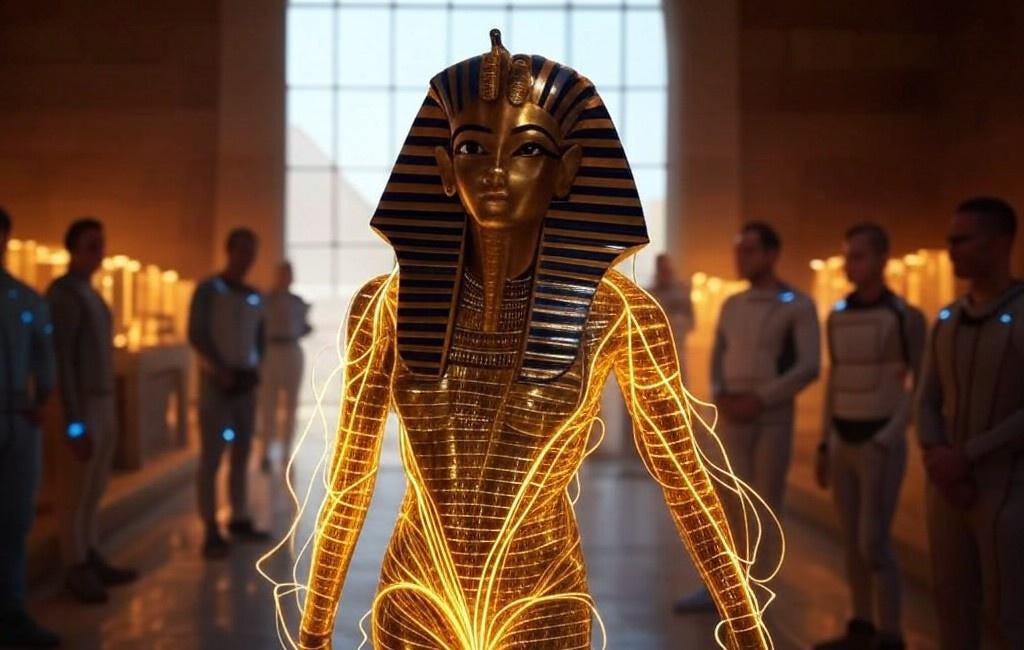
·
30 JULY 2024
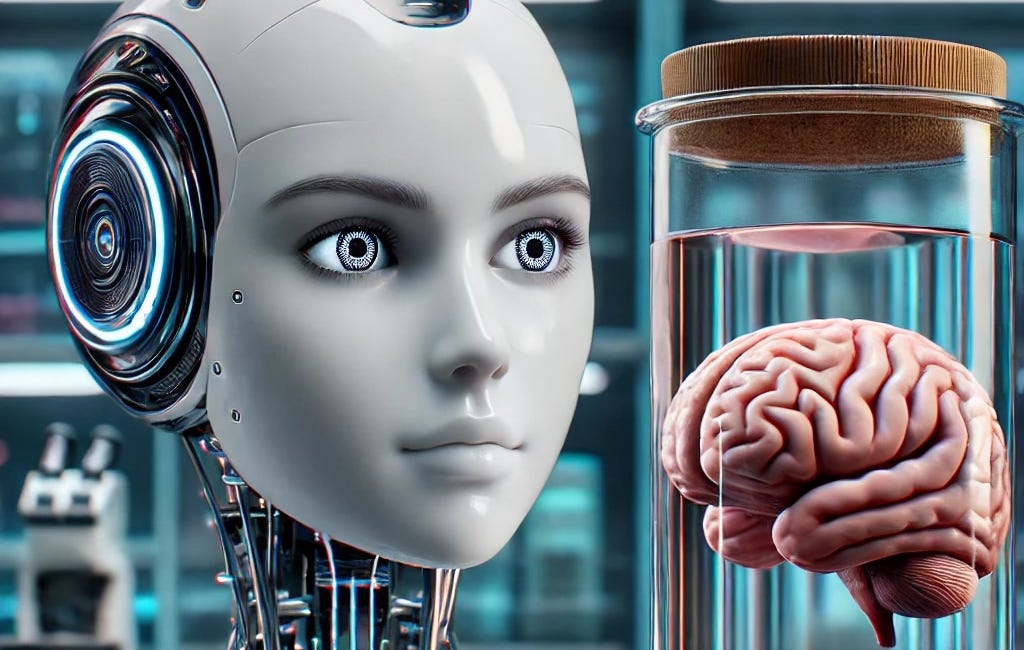