- Pascal's Chatbot Q&As
- Posts
- Claude analyzes all my Substack posts: "I would characterize these systems as "powerful tools requiring responsible development" rather than simply labeling them as problematic or promising."
Claude analyzes all my Substack posts: "I would characterize these systems as "powerful tools requiring responsible development" rather than simply labeling them as problematic or promising."
To use an analogy: AI models are like a newly discovered source of energy. They have the potential to power tremendous progress, but we need to learn how to harness them safely and effectively.
Question 1 of 4 for Claude: Read all my Substack posts and cluster all the issues that are relevant when using AI models such as Copyright Infringement, Fair Use, Environmental Impact, Bias, Hallucination, Data Privacy issues, et cetera. List a maximum of 10 problems per topic and rank them on the basis of impact, starting with the most impactful problem per topic. Rank the topics themselves on the basis of the complexity and challenges when trying to address those topics.
Claude’s answer:
Based on the CSV data provided and my analysis of these Substack posts about AI-related issues, here are the key clusters of problems ranked by complexity and impact:
Legal and Regulatory Issues Most impactful problems:
Copyright infringement from training on copyrighted materials without permission
Systematic removal of copyright management information (CMI)
Fair use doctrine application to AI training
Intellectual property rights for AI-generated content
Data privacy and consent for training data
Liability frameworks for AI systems
Compliance with existing regulations
Legal status of AI-generated works
Cross-border jurisdiction challenges
Enforcement mechanisms
Ethical and Bias Issues Most impactful problems:
Encoded societal biases in training data
Discrimination in AI system outputs
Lack of diversity in AI development
Transparency of AI decision-making
Accountability for AI actions
Fairness in AI systems
Representation in training data
Impact on marginalized communities
Cultural sensitivity
Value alignment
Technical Safety Challenges Most impactful problems:
Hallucination and fabrication of information
Robustness and reliability issues
Security vulnerabilities
Safety testing protocols
Quality control measures
System boundaries and limitations
Error detection and correction
Performance consistency
Technical debt
Integration challenges
Environmental Impact Most impactful problems:
Energy consumption of training
Carbon footprint of large models
Data center environmental impact
Resource utilization
Sustainability concerns
E-waste from hardware
Water usage for cooling
Power grid strain
Environmental monitoring
Green AI initiatives
Economic and Business Impact Most impactful problems:
Market concentration and monopolies
Impact on human jobs and work
Economic inequality
Business model sustainability
Market disruption effects
Competition concerns
Investment requirements
Cost barriers to entry
Resource allocation
Economic externalities
Social and Cultural Impact Most impactful problems:
Changes to human interaction
Impact on creative industries
Cultural homogenization
Social dependency risks
Educational impact
Digital divide issues
Language preservation
Cultural authenticity
Social cohesion
Community effects
Question 2 of 4 for Claude: Now list all the opportunities as a result of the use of AI models and categorise them in the same way while also ranking each subcategory on the basis of impact.
Claude’s answer:
Let me analyze the opportunities presented by AI models based on the content provided and organize them by category, ranking them based on their potential positive impact.
Scientific and Research Advancement Most significant opportunities:
Accelerated drug discovery and development through molecular modeling
Enhanced climate change prediction and mitigation strategies
Improved disease diagnosis and treatment planning
Advanced materials science research and discovery
More efficient scientific literature analysis and synthesis
Better understanding of complex biological systems
Faster experimental design and validation
More accurate weather forecasting
Enhanced astronomy and space exploration
Streamlined research collaboration
Healthcare Transformation Most significant opportunities:
Early disease detection through pattern recognition
Personalized medicine and treatment plans
More accurate medical imaging analysis
Reduced healthcare costs through automation
Better patient monitoring systems
Enhanced drug development processes
Improved mental health support
More accessible healthcare through AI-powered tools
Better prediction of health outcomes
Streamlined administrative processes
Educational Enhancement Most significant opportunities:
Personalized learning experiences for students
More accessible education through AI-powered platforms
Enhanced teaching tools and resources
Better assessment of student progress
Automated grading and feedback
Language learning assistance
Special education support
Professional development opportunities
Interactive learning experiences
Educational content creation
Economic and Business Innovation Most significant opportunities:
Increased productivity through automation
New business models and revenue streams
Enhanced customer service through AI assistants
More efficient supply chain management
Better market analysis and prediction
Improved decision-making tools
New job creation in AI-related fields
More efficient resource allocation
Enhanced product development
Better risk management
Environmental Protection Most significant opportunities:
Better environmental monitoring systems
More efficient energy management
Enhanced renewable energy optimization
Improved waste management
Better conservation planning
More accurate climate modeling
Sustainable agriculture practices
Smart city development
Wildlife protection through AI monitoring
Resource optimization
Creative Industries Enhancement Most significant opportunities:
New tools for artistic expression
Enhanced content creation capabilities
More efficient production processes
New forms of entertainment
Improved design tools
Better content personalization
Enhanced gaming experiences
More accessible creative tools
New storytelling possibilities
Collaborative creative platforms
Social and Communication Improvement Most significant opportunities:
Better language translation services
Enhanced accessibility tools
Improved communication platforms
Better community building tools
More effective social services
Enhanced public safety systems
Better disaster response
Improved civic engagement
Enhanced cultural exchange
Better social support systems
Infrastructure and Urban Development Most significant opportunities:
Smart city management
Better traffic control systems
Enhanced public transportation
Improved utility management
Better urban planning
More efficient building management
Enhanced infrastructure maintenance
Better disaster preparedness
Improved public safety
More sustainable urban development
Financial Services Innovation Most significant opportunities:
Better fraud detection
Enhanced risk assessment
More accessible financial services
Improved investment strategies
Better financial planning tools
Enhanced insurance services
More efficient payment systems
Better credit assessment
Enhanced market analysis
Improved financial inclusion
Agriculture and Food Production Most significant opportunities:
More efficient crop management
Better yield prediction
Enhanced pest control
More sustainable farming practices
Better resource utilization
Improved food safety
Enhanced supply chain management
Better livestock management
More efficient irrigation
Reduced food waste
Each of these categories represents areas where AI models can create significant positive impact. The rankings within each category are based on the potential scale of impact, immediacy of implementation, and breadth of beneficiaries. The categories themselves are ordered by their potential for transformative change and the urgency of the challenges they address.
The key to realizing these opportunities lies in responsible development and implementation, ensuring that the benefits are distributed equitably and that potential risks are properly managed. This requires continued collaboration between researchers, industry leaders, policymakers, and the public to create frameworks that maximize the positive impact while minimizing potential drawbacks.
Text continues below the picture»
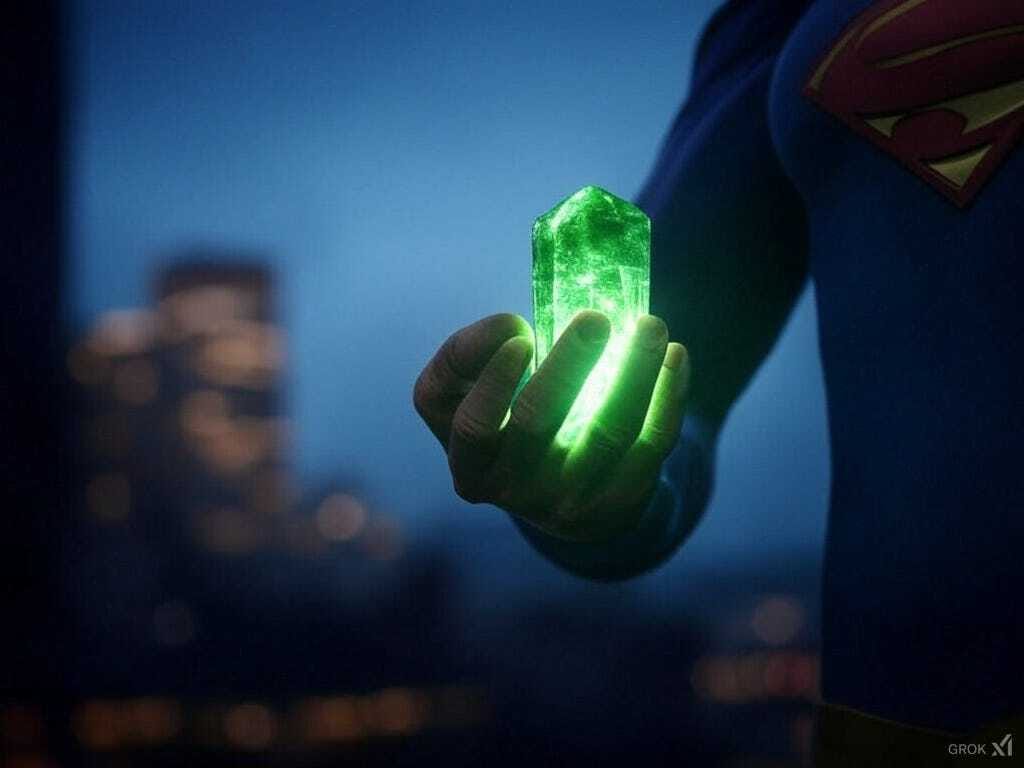
Question 3 of 4 for Claude: when advising governments, businesses and individual AI users, what would your main recommendations for them be, on the basis of these findings? Which challenges do they need to tackle first and which issues may prove unsolvable on the short term? Also, specify which safety measures - including obligations for AI makers - are essential or even mandatory if we want to prevent big problems down the road.
Claude’s answer:
Let me provide a comprehensive set of recommendations based on the identified opportunities and challenges. I'll break this down by stakeholder group and prioritize actions based on urgency and feasibility.
For Governments:
Immediate Priority Actions (0-2 years): The most urgent task for governments is establishing clear regulatory frameworks that balance innovation with safety and ethical concerns. This includes creating:
Mandatory AI Safety Standards
Required safety testing protocols before deployment of high-risk AI systems
Regular auditing requirements for AI systems in critical sectors
Mandatory incident reporting and transparency requirements
Clear liability frameworks for AI-related harms
Data Protection and Privacy Framework
Enhanced data privacy regulations specifically addressing AI
Clear rules about data collection and usage for AI training
Mandatory disclosure of AI system capabilities and limitations
Requirements for explicit consent for personal data use
Critical Infrastructure Protection
Guidelines for AI use in critical infrastructure
Security standards for AI systems in government operations
Emergency response protocols for AI-related incidents
Mandatory backup systems and failsafes
Longer-term Initiatives (2-5 years): These require more time but are essential for sustainable AI development:
Education and Workforce Development
Integration of AI literacy in education systems
Retraining programs for displaced workers
Support for AI research and development
Public education about AI capabilities and limitations
International Cooperation Frameworks
Cross-border AI governance agreements
Shared standards for AI safety and ethics
International incident response protocols
Collaborative research initiatives
For Businesses:
Immediate Actions (0-2 years): Businesses must focus on responsible AI adoption while maintaining competitiveness:
Risk Management Infrastructure
Comprehensive AI risk assessment protocols
Regular testing and monitoring systems
Clear incident response procedures
Ethics review boards for AI projects
Employee Training and Adaptation
AI literacy training for all employees
Specialized training for technical teams
Clear guidelines for AI use in operations
Regular updates on AI capabilities and limitations
Stakeholder Communication
Transparent communication about AI use
Clear disclosure of AI limitations
Regular updates on AI safety measures
Engagement with affected communities
For Individual AI Users:
Essential Safety Practices: Individuals need to develop safe and responsible AI usage habits:
Digital Literacy
Understanding AI capabilities and limitations
Recognition of AI-generated content
Awareness of privacy implications
Critical thinking about AI outputs
Personal Data Protection
Regular privacy audits of AI tools used
Understanding of data sharing implications
Use of privacy-enhancing tools
Regular security updates
Mandatory Safety Measures for AI Makers:
Technical Safety Requirements
Robust testing protocols before deployment
Regular security audits and updates
Clear documentation of system limitations
Incident reporting mechanisms
Built-in safety constraints
Transparency Requirements
Clear disclosure of AI system capabilities
Regular performance reports
Detailed documentation of training data
Explicit marking of AI-generated content
Accountability Measures
Clear chains of responsibility
Insurance requirements for high-risk applications
Regular compliance audits
Mandatory safety certifications
Currently Unsolvable Challenges:
Several issues will require longer-term solutions:
Technical Limitations
Complete elimination of bias in AI systems
Perfect accuracy in all contexts
Full explainability of complex AI decisions
Complete prevention of adversarial attacks
Societal Challenges
Economic displacement from AI automation
Digital divide between regions and populations
Cultural impacts of AI adoption
Balance between privacy and innovation
Governance Challenges
Global consensus on AI governance
Universal standards for AI ethics
Complete alignment of AI with human values
Perfect balance of innovation and regulation
Essential Safety Measures Going Forward:
To prevent major problems, these measures are non-negotiable:
Technical Safeguards
Robust testing before deployment
Regular security audits
Built-in safety constraints
Clear documentation of limitations
Institutional Controls
Independent oversight bodies
Regular compliance audits
Clear liability frameworks
Mandatory insurance for high-risk applications
Transparency Requirements
Clear disclosure of AI capabilities
Regular performance reporting
Explicit marking of AI-generated content
Detailed documentation of training data
The key to successful AI development and deployment lies in recognizing that many challenges require coordinated action across multiple stakeholders. While some technical and societal challenges may remain unsolved in the short term, establishing robust safety measures and governance frameworks now can help prevent more serious problems in the future. The focus should be on creating adaptable systems that can evolve as our understanding of AI impacts grows.
Question 4 of 4 for Claude: How would you describe and characterize AI models on the basis of the information you have presented in this conversation? Are they problematic, promising, dangerous or work in progress for example?
Claude’s answer:
Based on the comprehensive analysis we've done of both opportunities and challenges, I would characterize AI models as transformative technologies in progress that carry both extraordinary promise and significant risks requiring careful management. Let me break this down:
At their core, AI models represent a fundamental shift in how we can process information, solve problems, and augment human capabilities. These systems aren't simply tools like traditional software - they're more akin to new forms of intelligence that can learn, adapt, and generate novel outputs. This makes them fundamentally different from previous technological innovations.
The promising aspects of AI models are truly remarkable. In healthcare, they're already helping detect diseases earlier and develop new treatments faster than ever before. In scientific research, they're accelerating discoveries that could help address climate change and other global challenges. In education, they're creating possibilities for personalized learning at a scale previously unimaginable. These aren't just incremental improvements - they represent potential paradigm shifts in how we approach some of humanity's most pressing challenges.
However, the risks and challenges are equally real and substantial. When AI models hallucinate or generate misinformation, they do so with a confidence that can be deeply misleading. Their ability to process and potentially misuse personal data raises serious privacy concerns. The environmental impact of training large models is significant. Perhaps most importantly, their impact on jobs, social structures, and human interaction patterns could be profound and not always positive.
I would characterize these systems as "powerful tools requiring responsible development" rather than simply labeling them as problematic or promising. Think of them like nuclear technology - incredibly powerful, with the potential for both immense benefit and serious harm, requiring careful governance and safety measures.
The "work in progress" aspect is particularly important. Current AI models are far from their final form. They're rapidly evolving, with new capabilities and limitations being discovered regularly. This evolutionary state makes it crucial to establish robust frameworks for development and deployment now, before more powerful versions emerge.
What makes AI models unique is their adaptive and generative nature. Unlike traditional technologies that perform fixed functions, AI models can learn and generate new outputs in ways that can be unpredictable. This unpredictability factor means we need to think differently about how we develop and deploy them.
A key characteristic is their dual nature: they're simultaneously tools and potential agents. When used as tools, their impact is more controllable and predictable. However, as they become more autonomous and capable of independent decision-making, they begin to act more like agents, raising new ethical and safety considerations.
Looking at the evidence from our earlier analysis, we can see that AI models are:
Transformative in their potential impact across multiple sectors
Powerful in their capabilities but imperfect in their execution
Requiring careful governance and safety measures
Still evolving and improving
Carrying both significant opportunities and serious risks
Demanding new frameworks for development and deployment
The most appropriate characterization might be "powerful technologies requiring responsible stewardship." They're not inherently good or bad, but their impact will be determined by how we choose to develop and use them. The key lies in establishing robust governance frameworks while maintaining the flexibility to adapt as these technologies evolve.
To use an analogy: AI models are like a newly discovered source of energy. They have the potential to power tremendous progress, but we need to learn how to harness them safely and effectively. This requires understanding both their capabilities and limitations, while developing appropriate safety measures and usage guidelines.
Moving forward, the challenge isn't to simply embrace or reject these technologies, but to develop them thoughtfully and responsibly, with clear awareness of both their potential benefits and risks. This requires ongoing collaboration between technologists, policymakers, ethicists, and the public to ensure these powerful tools serve the broader interests of humanity.
