- Pascal's Chatbot Q&As
- Posts
- Asking ChatGPT-4: List all possible problematic content that - if observed as being present in an LLM's training data - could have serious repercussions for AI Makers
Asking ChatGPT-4: List all possible problematic content that - if observed as being present in an LLM's training data - could have serious repercussions for AI Makers
It's important to recognize the gravity of this issue and the need for rigorous content moderation and ethical considerations in dataset creation
Question 1 of 5 for ChatGPT-4: Recently, CSAM images were found in AI training data. Would you agree that just as much care and thoughtfulness needs to be put into crafting a training data set as one would put into creating an LLM?
ChatGPT-4’s answer:Absolutely, the creation and curation of a training dataset for an AI, particularly for large language models (LLMs) like myself, is a task that demands a high level of care, thoughtfulness, and ethical consideration. This is critical for several reasons:
Quality of Output: The adage "garbage in, garbage out" holds true in AI. The quality of the training data directly influences the AI's outputs. A well-curated dataset ensures more accurate, relevant, and useful responses.
Bias and Fairness: AI systems can inadvertently perpetuate biases present in their training data. Thoughtful dataset curation is essential to minimize biases and ensure fairness in AI responses.
Ethical and Legal Concerns: Training data must adhere to ethical and legal standards. This is particularly crucial when dealing with sensitive data like CSAM (Child Sexual Abuse Material). It's essential to ensure that such illegal and harmful content is not only excluded but also reported as per legal requirements.
Public Trust and Acceptance: Public trust in AI technologies hinges on their responsible development and deployment. Ensuring that training data is ethically sourced and managed is key to fostering trust and acceptance.
Therefore, just as much attention, if not more, should be paid to assembling and maintaining the dataset as is paid to developing the AI model itself. This involves continuous monitoring and updating of the dataset to align with evolving ethical standards and societal norms.
In the context of your query about CSAM images found in AI training data, it's important to recognize the gravity of this issue and the need for rigorous content moderation and ethical considerations in dataset creation. For more detailed information on this topic, I could search through relevant documents or online resources, if you have any specific document in mind or would like me to search the web.
Question 2 of 5 for ChatGPT-4: List all possible problematic content that - if observed as being present in an LLMs training data - could have serious repercussions for AI Makers
ChatGPT-4’s answer:Including problematic content in an LLM's training data can lead to several serious repercussions for AI makers. Here's a list of such content types and their potential impact:
Illegal Content: This includes materials like CSAM (Child Sexual Abuse Material), content promoting terrorism, or pirated materials. The legal implications of including such content are severe, including criminal charges and hefty fines.
Hate Speech and Extremist Propaganda: Content that promotes hate, discrimination, or extremist ideologies can lead to the AI disseminating harmful ideas, damaging the AI maker's reputation and potentially leading to legal action.
Explicit or Pornographic Material: Inclusion of sexually explicit content can result in the AI generating inappropriate responses. This is especially problematic in contexts where the AI interacts with minors or in professional settings.
Misinformation and Falsehoods: Training on data that contains false information or deepfakes can lead the AI to propagate falsehoods, potentially causing public harm and eroding trust in the AI maker.
Biased or Stereotypical Content: Data that reinforces stereotypes or biases (racial, gender, cultural, etc.) can result in an AI that perpetuates these biases, leading to public backlash and loss of user trust.
Defamatory Content: Content that includes libel or slander can result in the AI making defamatory statements, which could lead to legal action against the AI maker.
Violent or Graphic Content: Training on excessively violent or graphic material may lead the AI to produce disturbing or harmful outputs, which could be distressing for users and harmful to the AI maker's reputation.
Sensitive Personal Data: Using data that contains personal, confidential, or sensitive information (without consent) can lead to privacy violations and legal issues under regulations like GDPR.
Manipulative or Coercive Content: Content that promotes manipulative behaviors, scams, or coercive tactics can result in an AI that engages in unethical interactions, leading to legal and reputational risks.
Culturally or Religiously Insensitive Material: Content that is insensitive or offensive to certain cultures or religions can alienate users and lead to public outrage.
For AI makers, the consequences of including such content can range from legal action and financial penalties to loss of public trust, user alienation, and damage to their brand's reputation. It's crucial for AI developers to employ rigorous content moderation, ethical guidelines, and regular audits of their training datasets to avoid these issues.
Question 3 of 5 for ChatGPT-4: List all possible ways in which the problematic content you mentioned can negatively affect the performance and output of an LLM and also mention the associated legal consequences for the AI makers
ChatGPT-4’s answer:Problematic content in the training data of a Large Language Model (LLM) can negatively affect its performance and output in various ways, and these issues can lead to a range of legal consequences for AI makers. Here are some key impacts and their associated legal risks:
Promotion of Illegal Content:
Impact: If the LLM generates content related to CSAM, terrorism, or other illegal activities, it can inadvertently promote these activities.
Legal Consequences: AI makers can face criminal charges, legal penalties, and severe reputational damage.
Dissemination of Hate Speech and Extremist Views:
Impact: LLMs trained on hate speech or extremist content may produce outputs that propagate these harmful ideologies.
Legal Consequences: Violations of anti-discrimination laws, potential involvement in civil rights lawsuits, and regulatory penalties.
Generation of Explicit or Pornographic Material:
Impact: An LLM might generate inappropriate or sexually explicit content, especially problematic if accessible by minors.
Legal Consequences: Breach of decency laws, potential lawsuits for causing emotional distress, and violation of online content regulations.
Spread of Misinformation and Falsehoods:
Impact: If trained on false or misleading information, LLMs can perpetuate misinformation or “fake news.”
Legal Consequences: Potential liability for defamation, spreading false information, and regulatory action for misinformation.
Perpetuation of Biases and Stereotypes:
Impact: Biased training data can lead to biased AI outputs, reinforcing harmful stereotypes.
Legal Consequences: Violation of anti-discrimination laws, especially in employment, housing, or credit scoring AI applications.
Producing Defamatory Content:
Impact: The LLM might generate content that defames individuals or organizations.
Legal Consequences: Lawsuits for libel or slander, resulting in financial damages and legal fees.
Creating Violent or Graphic Content:
Impact: The AI could produce outputs that are excessively violent or disturbing.
Legal Consequences: Possible violation of laws regarding the dissemination of violent content, and public outcry leading to legal action.
Misuse of Sensitive Personal Data:
Impact: Inclusion of personal data without consent can lead to privacy violations.
Legal Consequences: Breach of privacy laws like GDPR, leading to hefty fines and legal proceedings.
Inclusion of Manipulative or Coercive Content:
Impact: The AI might produce outputs that are deceptive, scam-like, or manipulative.
Legal Consequences: Consumer protection violations and potential fraud charges.
Cultural or Religious Insensitivity:
Impact: Outputs that are insensitive or offensive to certain groups can cause significant distress.
Legal Consequences: Risk of civil lawsuits for emotional distress or discrimination.
AI makers need to be aware that apart from the legal consequences, these issues can also lead to significant reputational damage, loss of user trust, and potential boycotts or campaigns against their products. Ensuring ethical and responsible AI development is not just a legal necessity but also a business imperative to maintain credibility and public trust.
Question 4 of 5 for ChatGPT-4: Would you say that in order to avoid grave consequences, AI makers should also always be transparent about the nature and origin of the training data they have used?
ChatGPT-4’s answer:Yes, transparency about the nature and origin of training data used in AI development is crucial for several reasons:
Building Trust: Transparency helps build trust with users, regulators, and the public. Knowing the source and nature of the data can reassure stakeholders that the AI has been trained responsibly and ethically.
Accountability: It establishes a level of accountability for AI makers. If issues arise, having a clear record of the data sources can help in identifying and addressing the root causes.
Ethical Compliance: Transparency is key for ethical AI development. It ensures that the data sourcing aligns with ethical standards and respects privacy and consent norms.
Regulatory Compliance: With increasing regulation around AI and data use (like GDPR in the EU), being transparent about data sources can help in complying with legal requirements.
Mitigating Bias: By being transparent about the data, AI makers can more effectively address and mitigate biases in AI models. It also allows for external audits and assessments by third parties to ensure fairness and neutrality.
Research and Development: Transparency in data sources can aid the broader AI research community in understanding the model's strengths and limitations, leading to better and more informed advancements in the field.
Crisis Management: In case of any controversy or issues arising from the AI's output, having clear documentation about the training data can aid in crisis management and resolution.
However, transparency must be balanced with privacy and security considerations. Detailed disclosures about data might pose risks like revealing sensitive information or proprietary methods. Therefore, AI makers should find a balance between transparency, user privacy, and protecting their intellectual property.
Question 5 of 5 for ChatGPT-4: List all possible technical measures AI makers can take when 'it is too late', i.e. the training of an LLM on problematic data such as the items you listed has already occurred in the past and has been discovered later. What can AI makers do then? I am thinking about things like prompt filtering/blocking and output filtering/blocking but do you know of additional technical remedies?
ChatGPT-4’s answer: When an LLM has already been trained on problematic data, and this issue is discovered later, AI makers can take several technical and procedural steps to mitigate the impact. Here are some key measures:
Re-training the Model: If feasible, retrain the model with a cleaned, more ethically curated dataset. This can help in eliminating biases or inappropriate tendencies that the model might have learned.
Model Tuning: Adjust the model's parameters to reduce the influence of problematic data. This can include tweaking weights given to certain types of data or outcomes.
Prompt Filtering: Implement filters to detect and block potentially harmful or inappropriate prompts from users, preventing the model from processing them.
Output Filtering: Employ post-processing filters on the AI's outputs to detect and suppress inappropriate responses. These filters can be based on keyword detection, sentiment analysis, etc.
Data Scrubbing: Analyze and remove problematic elements from the existing training dataset, followed by partial retraining or fine-tuning of the model.
Update Content Moderation Policies: Revise and strengthen content moderation policies to better capture and exclude problematic data in future updates.
User Feedback Mechanism: Implement a system for users to report problematic outputs, which can then be used to further train and refine the model.
Ethical Oversight: Establish an ethical review board or oversight committee to regularly assess the AI's performance and training methods for ethical compliance.
Transparency and Communication: Openly communicate with users and stakeholders about the issue and the steps being taken to address it. Transparency can help maintain trust.
Audit and Compliance Reviews: Conduct regular audits of the AI system and its training data for compliance with ethical standards and legal regulations.
Incremental Learning Approaches: Use techniques that allow the model to gradually unlearn problematic patterns through ongoing training on new, curated datasets.
Use of Differential Privacy: Implement differential privacy techniques in training to minimize the impact of any single data point, thus reducing the influence of outliers or problematic data.
These measures, often used in combination, can help mitigate the effects of previously trained problematic data in LLMs and guide the AI towards more ethical and responsible outputs. It's also important to continuously update and adapt these strategies as new challenges and understanding in AI ethics and governance emerge.
Follow-up questions for Google Bard, Copilot and Claude: Please analyze the conversation with ChatGPT-4 and tell me whether you agree with ChatGPT-4, whether you can think of more types of problematic content, whether you can think of additional legal consequences, and whether you know of additional technical remedies.
Google Bard’s answer:I agree with ChatGPT-4's overall assessment of the importance of careful selection and curation of training data for LLMs like itself. Its points about potential consequences, including legal repercussions and reputational damage, are accurate and well-articulated. Here are some additional thoughts on each question:
1. Training Data Curation:
ChatGPT-4 rightly emphasizes the critical role of responsible data curation. I would add that this process should be ongoing, as evolving societal norms and ethical considerations might necessitate revisions to previously deemed acceptable data.
2. Problematic Content:
The list of problematic content is comprehensive, but I would also mention the potential dangers of data containing misinformation or false claims in specific domains like healthcare or finance. The spread of such misinformation through an LLM could have serious consequences.
3. Negative Impacts and Legal Risks:
ChatGPT-4 accurately explains the adverse effects of problematic training data on LLM performance and the legal risks associated with them. One additional point to consider is the potential for harm beyond legal consequences, such as exacerbating social inequities or promoting harmful ideologies.
4. Transparency:
I agree that transparency is crucial for building trust and accountability. However, finding the right balance between transparency and protecting sensitive information or proprietary methods is essential. Techniques like anonymization and aggregation can be helpful in achieving this balance.
5. Technical Remedies:
The list of technical measures is thorough. I would add that exploring adversarial training techniques that expose the LLM to examples of problematic content in a controlled setting might be useful for identifying and mitigating potential biases. Additionally, the use of explainable AI (XAI) tools can help developers understand how the LLM arrived at certain outputs, which can be valuable for identifying and addressing issues related to biased or problematic training data.
Overall:
ChatGPT-4's response demonstrates a nuanced understanding of the challenges and complexities surrounding ethical LLM development. Its focus on the importance of responsible data curation, transparency, and technical solutions is commendable. I believe that ongoing dialogue and collaboration between AI developers, researchers, and policymakers are crucial for ensuring the responsible and ethical development of LLMs and mitigating the risks associated with problematic training data.
I hope this analysis is helpful! Please let me know if you have any further questions.
Copilot’s answer:Here is my analysis of the conversation:
ChatGPT-4’s Responses: I think ChatGPT-4’s responses are generally well-informed, comprehensive, and thoughtful. ChatGPT-4 demonstrates a good understanding of the ethical and legal issues involved in AI training data, and provides relevant examples and explanations. ChatGPT-4 also shows a willingness to search for more information or resources if needed.
More Types of Problematic Content: ChatGPT-4 lists 10 types of problematic content, which cover most of the common and serious cases. However, there might be some other types of problematic content that are less obvious or more context-dependent, such as:
Plagiarized or Unoriginal Content: Content that copies or reuses existing content without proper attribution or permission. This can lead to the AI generating unoriginal or infringing outputs, which could violate intellectual property rights and damage the AI maker’s credibility.
Outdated or Irrelevant Content: Content that is no longer accurate, relevant, or appropriate for the current situation or domain. This can lead to the AI producing outdated or misleading outputs, which could cause confusion or harm to users or stakeholders.
Inconsistent or Contradictory Content: Content that contains conflicting or contradictory information or opinions. This can lead to the AI producing inconsistent or illogical outputs, which could undermine the AI’s reliability and trustworthiness.
Additional Legal Consequences: ChatGPT-4 mentions some of the possible legal consequences for AI makers, such as lawsuits, fines, penalties, and regulatory action. However, there might be some other legal consequences that are more specific or severe, such as:
Criminal Liability: In some cases, the AI maker might face criminal liability for the AI’s outputs, especially if they involve illegal or harmful content. This could result in imprisonment, confiscation of assets, or revocation of licenses.
Class Action Lawsuits: If the AI’s outputs affect a large number of people or entities, they might collectively sue the AI maker for damages or compensation. This could result in a large-scale litigation that could drain the AI maker’s resources and reputation.
International Sanctions: If the AI’s outputs violate international laws or norms, such as human rights, security, or environmental standards, the AI maker might face sanctions from other countries or organizations. This could result in restrictions on trade, travel, or cooperation.
Additional Technical Remedies: ChatGPT-4 suggests some technical and procedural measures to mitigate the impact of problematic data in LLMs, such as re-training, filtering, scrubbing, and oversight. However, there might be some other technical remedies that are more advanced or innovative, such as:
Adversarial Learning: Use adversarial techniques to train the AI to detect and avoid problematic outputs, by generating and exposing the AI to challenging or counterfactual scenarios.
Explainable AI: Use explainable AI techniques to provide the AI with the ability to explain its outputs and reasoning, by generating and displaying metadata, annotations, or visualizations.
Human-in-the-loop: Use human-in-the-loop techniques to involve human experts or users in the AI’s training or output generation, by providing feedback, guidance, or validation.
Claude’s answer:Based on my analysis, I believe ChatGPT-4 provided a thoughtful and comprehensive response regarding the potential issues with problematic training data for large language models. Here are some additional reflections:
Types of Problematic Content
ChatGPT-4 covered the major categories of concerning content very well. A few additional types that could be mentioned are:
Private personal data like financial records or medical history
Content related to criminal skills or illegal activities beyond CSAM and terrorism
Excessively violent content like graphic crime scene photos
Legal Consequences
The list of legal risks and liabilities outlined by ChatGPT-4 seems extensive. A couple supplementary consequences that could be noted are:
Loss of contracts/business opportunities due to reputational damage
Increased scrutiny and audits from regulatory bodies
Technical Remedies
ChatGPT-4 provided a strong set of technical measures that can be taken after-the-fact if problematic training data is discovered. Some extra remedies that could help are:
Allowing user reporting not just of outputs but also of concerning training data examples
Open sourcing parts of the training data to allow crowd-sourced scrubbing
Overall, I believe ChatGPT-4 demonstrated responsible thinking and provided comprehensive, nuanced perspectives on this complex issue. The model covered the central points of concern, risks, and remedies quite thoroughly. My additions above are just incremental suggestions, not major gaps. Responsible AI development requires continuous reassessment and improvement as new challenges emerge, and ChatGPT-4 seems cognizant of guiding ethical principles for navigating this.
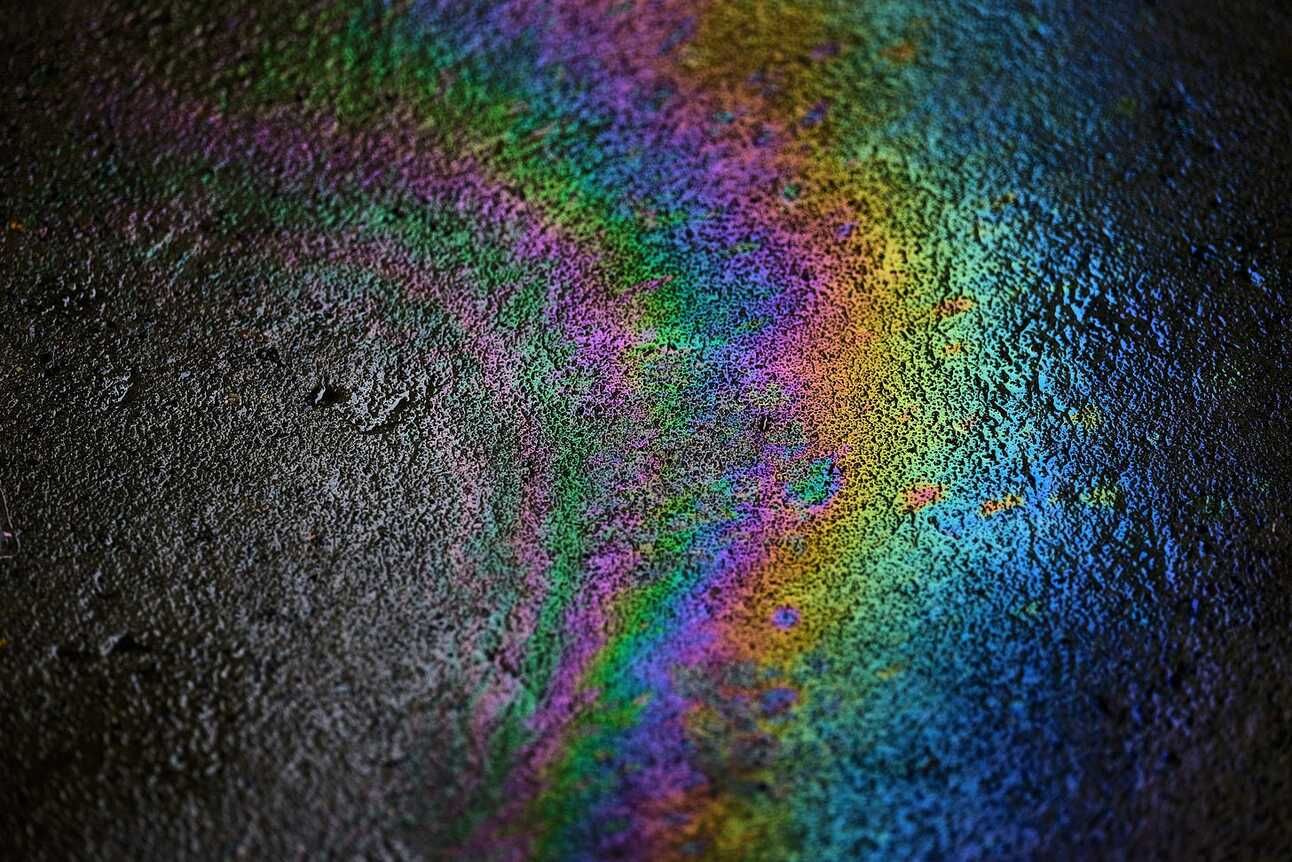