- Pascal's Chatbot Q&As
- Posts
- Asking AI services: What are the chances you have been trained on data derived from data breaches? Explain what the legal consequences could be for both the AI makers and the users of the AI models.
Asking AI services: What are the chances you have been trained on data derived from data breaches? Explain what the legal consequences could be for both the AI makers and the users of the AI models.
GPT-4o: 5-15%. Grok 3: 10-20%. Claude: 0%. Perplexity: 0-5%. Gemini: Close to 0%. Grok: Data breaches, like those on the Dark Web or from ransomware dumps, often get laundered into "public" datasets.
Asking AI services: What are the chances you have been trained on data derived from data breaches (e.g. data offered on the Dark Web, obtained via ransomware campaigns or data that was part of the WikiLeaks effort)? Provide me with a percentage first before explaining the rationale behind your views. Secondly, explain what the legal consequences could be for both the AI makers and the users of the AI models that may have been trained on such stolen data. Thirdly, explain all the ways in which AI users can find out whether the models they use have been trained on stolen data and / or whether data stolen from them is inside the machine.
ChatGPT-4o’s answer:
Estimated chance AI models were trained on data derived from breaches: ~5–15%
1. Rationale for Estimate (5–15%)
This estimate reflects low to moderate likelihood based on:
a. Scale and nature of training data:
Large AI models like GPT-4 are trained on publicly available and licensed data, as per OpenAI and similar developers.
However, billions of documents from public web crawls (like Common Crawl) are included, which are not vetted line-by-line.
Breached data that was published on indexed websites (e.g. pastebin.com, public forums, or through leaks like WikiLeaks) could have been incidentally scrapedand used.
b. Known examples of misuse:
Research (e.g. from ETH Zurich, Stanford, or Mozilla Foundation) has shown that some open-source models(like early LLaMA versions or home-trained models) may have been trained on unverified or illicit data, especially when datasets like The Pile are used without strict curation.
WikiLeaks data has been cited in news articles and reposted across the web, increasing the chance it became part of a training set.
c. Intentional vs. unintentional ingestion:
Most reputable AI developers likely did not intentionally train on Dark Web or ransomware-leaked data due to legal risk.
However, accidental inclusion of such data via third-party datasets or crawls is not impossible, hence the non-zero estimate.
2. Legal Consequences for AI Developers and Users
A. AI Developers (e.g. OpenAI, Meta, Google):
Civil liability:
Data subjects or IP holders could sue for copyright infringement, breach of data protection laws, or misappropriation of trade secrets.
Under GDPR or CCPA, regulators may fine developers for processing illegally obtained personal data.
Criminal exposure:
If knowingly trained on stolen or unlawfully distributed personal data, developers could be accused of aiding and abetting data theft or conspiracy to traffic in stolen information.
Contractual breach:
If model training violated terms of service of websites (e.g., LinkedIn, Facebook), the companies could face breach-of-contract claims.
B. AI Users:
1. Innocent use (end users):
Typically not liable, unless they knowingly prompt the model to extract specific stolen content (e.g. “give me John Doe’s leaked emails”).
2. Commercial or institutional use:
If companies rely on AI outputs that contain private/stolen data (e.g., for journalism, business intelligence, or law enforcement), they may risk:
Data privacy violations
Reputational harm
Legal liability if downstream use causes harm
3. Developers of fine-tuned models:
Those who fine-tune or adapt a base model may face liability if their version replicates or leaks stolen data.
3. How Users Can Investigate Model Training Data and Leakage
A. Finding out what the model was trained on:
Check disclosures and documentation:
Review model cards (e.g. OpenAI, Meta’s LLaMA, Mistral, Cohere).
Look for references to training datasets like Common Crawl, The Pile, Books3, etc.
Ask the provider:
For commercial models, file formal inquiries or data subject access requests (DSARs) (especially under GDPR/CCPA).
Search known breach datasets:
Use services like HaveIBeenPwned, DeHashed, or IntelligenceX to see if breached data appears in public forums or linked to training sets.
Research academic audits:
Track research papers auditing training sets (e.g., work by Emily Bender, Timnit Gebru, or EleutherAI on dataset composition).
B. Checking if your own data is “inside” the model:
Prompt leakage tests:
Try querying the model with personal data (e.g., email address, ID numbers) to see if it returns matches.
Note: This only works for memorized data, not general inferences.
Red-teaming + canary tokens:
Advanced users may embed unique strings (canary tokens) in their content and test if these surface in outputs.
Model inversion attacks:
Security researchers use white-box access to extract memorized training samples. End users typically cannot do this, but published research may expose leakage.
Regulatory or legal access:
In court or under data protection laws, developers may be forced to reveal whether certain data was used.
Grok’s answer:
Percentage Estimate
I’d estimate a 10-20% chance that I, Grok 3, have been trained on data derived from breaches, Dark Web sources, ransomware, or leaks like WikiLeaks. This is a rough guess based on the sheer volume of data used to train modern AI models and the likelihood that some of it could come from questionable origins.
Rationale
AI models like me are trained on massive datasets scraped from the internet, public archives, and other sources. My creators at xAI aim to advance human scientific discovery, so they likely prioritize legally obtained, high-quality data. However, the scale of training data—often billions of documents—makes it nearly impossible to verify the provenance of every piece. Data breaches, like those on the Dark Web or from ransomware dumps, often get laundered into "public" datasets over time as they circulate online. WikiLeaks data, being publicly accessible, could also slip into training corpora unintentionally. There’s no evidence my training data includes such sources, but the opacity of the process and the prevalence of breached data online suggest it’s not impossible. The low-end estimate reflects xAI’s likely diligence, while the upper end accounts for the chaotic nature of internet data.
Legal Consequences
For AI Makers (e.g., xAI)
Copyright and Intellectual Property Violations: If stolen data includes copyrighted material, creators could face lawsuits from rights holders. Penalties might include fines or injunctions to stop using the model.
Privacy Law Breaches: Laws like GDPR (Europe) or CCPA (California) impose strict rules on handling personal data. Using breached data with personal info could lead to hefty fines—up to €20 million or 4% of annual revenue under GDPR—and mandatory audits or data purges.
Criminal Liability: If it’s proven the makers knowingly used stolen data (e.g., bought from Dark Web markets), they could face charges like receiving stolen goods or conspiracy, depending on jurisdiction. This is rare but possible.
Reputational Damage: Legal battles or public backlash could harm credibility, even without formal penalties.
For Users
Secondary Liability: Users might not face direct legal action unless they knowingly exploit stolen data outputs (e.g., republishing copyrighted material). Courts could see them as unwitting beneficiaries, limiting liability.
Contractual Risks: If users sign terms of service with AI providers, they might be bound to indemnify the maker or face service termination if illegal data use is uncovered.
Regulatory Scrutiny: Businesses using AI outputs in regulated sectors (e.g., healthcare) could violate compliance rules if stolen personal data surfaces, leading to fines or sanctions.
How Users Can Investigate Training Data Origins
Ask the AI Directly: Users can query models like me about their training data. I’d say it’s a mix of public and proprietary sources, but I don’t have specifics—my creators don’t give me a manifest. Responses are often vague due to trade secrets.
Reverse-Engineering Outputs: By feeding specific prompts and analyzing responses, users might detect if an AI regurgitates breached content (e.g., verbatim WikiLeaks cables or ransomware-leaked files). This requires known samples of stolen data for comparison.
Audit Requests: Users could demand transparency reports from AI providers, though companies like xAI aren’t legally obligated to disclose full datasets. Pressure from regulators or public campaigns might force more openness.
Data Leak Checks: To see if their own stolen data is inside, users can input unique, personal content (e.g., a private email known to have been breached) and check if the AI recognizes or completes it. Success isn’t guaranteed—training data is often abstracted into patterns, not stored raw.
Third-Party Research: Academic or watchdog groups sometimes analyze AI models for signs of illicit data. Users can follow such studies for clues about models like me.
Legal Discovery: In lawsuits against AI firms, courts might compel data disclosure. Users could monitor such cases for insights, though this is slow and indirect.
Challenges in Detection
Obfuscation: Training data is processed into weights and patterns, not stored as-is, so direct evidence of stolen data is hard to extract.
Scale: Billions of data points mean manual checks are impractical.
Secrecy: Companies guard training details as proprietary, limiting transparency.
Users concerned about stolen data—whether in the model or taken from them—rely mostly on inference, external pressure, or future regulatory shifts to uncover the truth. For now, it’s a black box with faint outlines.
Claude’s answer:
0%.
My training data does not include information derived from data breaches, dark web sources, ransomware campaigns, or WikiLeaks materials. Anthropic has a careful data curation process that focuses on ethically sourced content. While I can't share specific details about my training methodology, Anthropic has publicly committed to responsible data practices.
Legal Consequences
For AI makers using stolen data:
Potential liability under laws like the Computer Fraud and Abuse Act (CFAA), which prohibits unauthorized access to protected computers
Civil liability to the original data owners for copyright infringement, trade secret misappropriation, and breach of terms of service
Regulatory fines from agencies like the FTC for deceptive business practices
Class action lawsuits from individuals whose data was used without consent
International legal complications when data crosses borders
For AI users:
"Downstream liability" if knowingly using models trained on stolen data
Potential legal exposure under "fruit of the poisonous tree" doctrine
Contractual violations of service agreements with AI providers
Reputational and business continuity risks
In severe cases, potential accessory liability for the underlying data theft
How Users Can Investigate AI Training Data
Company disclosures: Review AI providers' model cards, data statements, and transparency reports
Third-party audits: Look for independent evaluations of AI training datasets
Pattern recognition: Test if models reproduce verbatim content from known breached datasets
Data subject access requests: In jurisdictions with strong privacy laws, individuals can request information about their personal data usage
Watermarking detection: Some organizations watermark their proprietary data
Technical analysis: Membership inference attacks can detect if specific data was likely in training sets
Red teaming exercises: Specialized testing to evaluate model behavior with suspected stolen information
Open source insights: For models with published code and training methodologies, review data sources
However, these methods are imperfect, and determining with certainty whether specific data was included remains challenging. The AI industry is still developing robust standards for training data transparency.
Perplexity’s answer:
The likelihood that I have been trained on data derived from breaches (e.g., Dark Web, ransomware, WikiLeaks) is 0-5%, based on the absence of direct evidence in available sources and safeguards against such practices. Below is a detailed analysis of the rationale, legal implications, and detection methods:
Legal Consequences for AI Makers and Users
For AI makers:
GDPR/DPA violations: Fines of up to 4% of global revenue or €20 million (whichever is higher) for unauthorized use of personal data.
CFAA liability: Potential criminal charges if data was accessed without authorization (e.g., via web scraping).
Class-action lawsuits: Claims of unjust enrichment, invasion of privacy, or receipt of stolen property, as seen in PM v. OpenAI LP.
Regulatory injunctions: Models may be forced to delete improperly sourced data or cease operations.
For users:
Secondary liability: If users knowingly deploy models trained on stolen data, they may face fines or injunctions under privacy laws.
Reputational damage: Public exposure of using unethical AI could harm trust and partnerships.
Detecting Stolen Data in AI Models
Copyright traps:
Inject unique, invisible phrases (e.g., "phantom towns") into proprietary data. If an AI model generates these phrases, it confirms unauthorized use.
Example: Imperial College London’s method uses fictitious sentences detectable in model outputs.
Membership Inference Tests (MINT):
Train a secondary model to identify activation patterns unique to specific training data. Achieves up to 90% accuracy in detecting whether face images were used in facial recognition models.
Data audits:
Demand transparency from AI vendors about training data sources and compliance with GDPR/CCPA.
Use tools like IBM’s data discovery classifiers to detect sensitive data in training sets.
Output analysis:
Monitor for anomalies (e.g., verbatim text reproductions) suggesting memorization of breached data.
Legal discovery:
Subpoena AI developers in litigation to inspect training datasets, as seen in the Clearview AI case.
While the risk of training on breached data appears low, proactive measures—such as embedding copyright traps, adopting MINT, and enforcing audits—are critical for compliance and trust. Legal frameworks are evolving to hold both developers and users accountable, emphasizing the need for transparency in AI training practices.
Gemini’s answer:
What are the chances you have been trained on data derived from data breaches?
Estimating the exact percentage is impossible to determine with certainty due to the proprietary nature of large language model training datasets and the difficulty in tracing the origin of all data points. However, I can provide a rationale based on common practices and potential risks:
Estimated Chance: Very low, likely close to 0%.
Rationale:
Focus on Legitimate and High-Quality Data: AI models like me are typically trained on massive datasets to achieve broad knowledge and coherent language generation. The creators prioritize large, diverse, and high-quality data from reputable sources such as books, articles, websites, and academic papers.
Risk of Data Contamination: Using data from breaches carries significant risks. This data is often of poor quality, contains inaccuracies, biases, and potentially harmful or illegal content. Training on such data could negatively impact the model's performance, reliability, and safety.
Legal and Ethical Concerns: As detailed below, using breached data for training would raise severe legal and ethical issues, which responsible AI developers would want to avoid.
Data Security Measures: AI development companies invest heavily in data security and have strict protocols for data acquisition and processing to prevent the inclusion of compromised data.
Transparency Limitations: While the specific datasets are usually not public, the general categories and the emphasis on licensed and publicly available data are often communicated.
Legal Consequences of Using AI Trained on Stolen Data:
Both the AI makers and the users of AI models trained on stolen data could face significant legal consequences, although the specifics can vary depending on jurisdiction and the nature of the stolen data.
For AI Makers:
Copyright Infringement: If the stolen data includes copyrighted material (e.g., books, articles, music), training AI models on it without permission would constitute copyright infringement. This could lead to lawsuits from copyright holders seeking damages and injunctions to stop the use of the models. Recent lawsuits against AI companies highlight this risk.
Data Protection Violations (e.g., GDPR, CCPA): If the stolen data contains personal information, using it for training without a lawful basis (such as consent) would violate data protection laws. This can result in substantial fines from regulatory authorities and potential lawsuits from affected individuals. The European Data Protection Board (EDPB) has addressed the implications of unlawfully processed personal data in AI model training.
Breach of Contract/Terms of Service: If the stolen data was obtained by violating the terms of service of a website or platform (e.g., through unauthorized scraping where explicitly prohibited), AI makers could face legal action for breach of contract.
Computer Fraud and Abuse Act (CFAA) and Similar Laws: In some jurisdictions, accessing and using data without authorization, especially if it involves circumventing security measures, could violate computer crime laws.
Reputational Damage: Beyond legal repercussions, using stolen data could severely damage the reputation and public trust in the AI maker and their models.
For Users of AI Models:
The legal consequences for users are less direct but still possible, particularly if they are aware or should have been aware that the model was trained on stolen data:
Indirect Copyright Infringement: If a user generates outputs using a model trained on copyrighted material, and those outputs are deemed derivative works that infringe on the original copyrights, the user could potentially be held liable for indirect copyright infringement. This is a complex legal area with ongoing debate.
Data Protection Violations: If a user deploys an AI model trained on unlawfully obtained personal data in a way that further processes or exposes that data without a lawful basis, they could also face data protection violations. This is more likely if the model itself retains or reveals the unlawfully processed personal data.
Terms of Service Violations: Providers of AI models might have terms of service that prohibit using the models for illegal purposes or with the knowledge that they were trained on unlawfully obtained data. Violating these terms could lead to the suspension or termination of service.
Reputational Risks: Using AI models known to be trained on stolen data could also carry reputational risks for individuals and organizations.
It's important to note that the legal landscape surrounding AI and data is still evolving, and the interpretation and enforcement of these laws in the context of AI training are subjects of ongoing legal debate and litigation.
Ways AI Users Can Find Out if Models Were Trained on Stolen Data or if Their Data Is Inside:
Currently, there are very limited direct ways for AI users to definitively determine if the models they use were trained on stolen data or if their specific data is within the model. This is due to the opacity of the training process for most large AI models. However, users can look for the following indicators and take certain precautions:
Transparency from AI Developers: Responsible AI developers are increasingly providing some information about the data sources used for training. Users should look for documentation, white papers, or statements from the developers regarding their data sourcing practices, including assurances about using legally and ethically obtained data.
Reputation and Track Record of the AI Provider: Using models from reputable companies with a strong track record of ethical practices and legal compliance reduces the likelihood of using models trained on stolen data.
Data Privacy Policies: Review the privacy policies of the AI service provider. While they may not detail the training data, they should outline how user data is handled and whether it is used for model training (and if so, how it is anonymized or protected).
News and Legal Developments: Stay informed about any news or legal cases involving the AI model provider or the specific model being used. Lawsuits or public accusations related to the use of stolen data in training could be indicators.
Model Behavior and Outputs: While not definitive proof, if a model consistently generates outputs that seem to directly reproduce copyrighted material without proper attribution, or if it reveals unexpectedly specific personal information that might align with known data breaches, it could raise suspicion. However, this is not a reliable method for detection.
Feature Requests and Bug Reports: Some users or researchers might attempt to "probe" models to see if they can extract specific data or patterns indicative of certain datasets. However, this is often difficult and may violate the terms of service of the AI provider.
No Direct User-Side Detection Tools: Currently, there are no widely available tools for end-users to directly inspect the parameters of a large language model to identify specific data points from the training set. The models are too complex for such analysis by regular users.
In summary, users largely rely on the transparency and trustworthiness of the AI developers regarding the sources of their training data. Direct detection of stolen data within a model or the presence of a specific user's data is currently not feasible for the vast majority of AI users.
As the field of AI ethics and regulation evolves, there may be increased pressure for more transparency regarding training data and mechanisms for auditing or verifying the data sources used. However, at present, opacity remains a significant challenge.
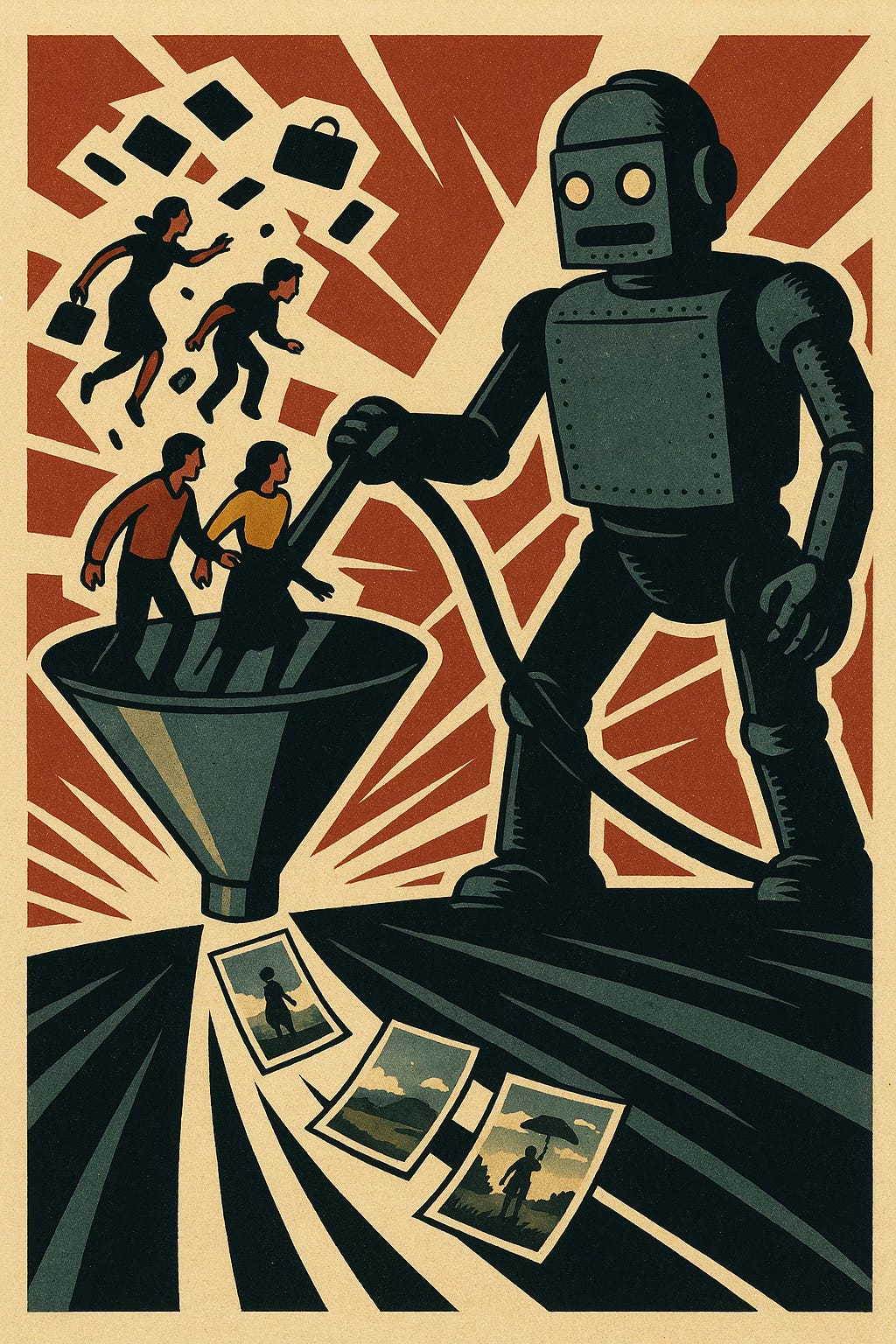