- Pascal's Chatbot Q&As
- Posts
- Asking AI services: Is the tech industry pouring billions into a dead end? GPT-4o: While AI remains powerful, the path forward requires more than just bigger models.
Asking AI services: Is the tech industry pouring billions into a dead end? GPT-4o: While AI remains powerful, the path forward requires more than just bigger models.
Claude: We're reaching the limits of what scaling alone can achieve, and new algorithmic innovations, hybrid approaches, and fundamental rethinking may be necessary.
Asking AI services: Is the tech industry pouring billions into a dead end?
ChatGPT-4o’s answer:
The tech industry may be pouring billions into a dead end, according to a majority of AI researchers surveyed in a recent report. Here are the key findings from the survey and report:
1. Scaling Up AI Won’t Lead to AGI
76% of AI researchers believe that simply scaling up current AI models (adding more data and computing power) will not lead to Artificial General Intelligence (AGI).
Stuart Russell (UC Berkeley) commented that the benefits of scaling have plateaued, and more fundamental breakthroughs are needed.
2. The AI Investment Bubble
AI companies poured $56 billion into generative AI in 2024 alone.
Microsoft alone is investing $80 billion in AI infrastructure for 2025.
There are signs that returns are diminishing, with OpenAI’s latest model showing less improvement than previous versions.
3. Energy and Environmental Concerns
The AI race has led to massive energy consumption, with Microsoft, Google, and Amazon signing nuclear power deals to sustain their data centers.
The Chinese startup DeepSeek has shown that cheaper and more efficient AI models can compete with billion-dollar models, questioning the industry’s scaling approach.
4. Alternatives to Scaling
Instead of brute-force scaling, companies are looking at:
Test-time compute (AI spending more time “thinking” before answering).
Mixture of Experts (splitting AI into specialized sub-models).
Neuro-symbolic approaches (combining logic and learning).
5. The AI Research Community’s Perspective
The AAAI 2025 Presidential Panel also highlights that AI research needs to move beyond big-data scaling and focus on AI reasoning, ethics, and sustainability.
Researchers suggest AI will need new architectures, transparency, and interdisciplinary approaches to truly advance.
Conclusion
The tech industry’s current AI investments may not be leading to AGI or long-term breakthroughs. Many researchers argue that companies are over-relying on scaling up models, rather than innovating in areas like symbolic reasoning, efficiency, and new architectures. While AI remains powerful, the path forward requires more than just bigger models.
Grok’s answer:
Based on the information from the documents you provided, particularly the Futurism article and the AAAI 2025 Presidential Panel report, a significant number of AI researchers believe that the tech industry's heavy investment in scaling current AI approaches—primarily through building larger models and expanding computational infrastructure—may indeed be leading toward a "dead end" in terms of achieving artificial general intelligence (AGI).
The Futurism article, dated March 18, 2025, highlights a survey conducted by the Association for the Advancement of Artificial Intelligence (AAAI) involving 475 AI researchers. A striking 76% of these respondents deemed it "unlikely" or "very unlikely" that simply scaling up existing AI methods, such as large language models (LLMs), would lead to AGI—a system capable of matching or surpassing human cognitive abilities across a broad range of tasks. This skepticism stems from the observation that the benefits of scaling have "plateaued," as noted by Stuart Russell, a prominent AI researcher from UC Berkeley. Despite massive investments, such as the $56 billion in venture capital funding for generative AI in 2024 and Microsoft's planned $80 billion expenditure on AI infrastructure in 2025, the returns in terms of fundamental breakthroughs appear to be diminishing.
The article points to examples like OpenAI’s upcoming GPT model showing "significantly less improvement" compared to previous iterations, and Google CEO Sundar Pichai admitting that "easy AI gains" are over. Meanwhile, alternative approaches from companies like DeepSeek, which achieved competitive performance with less training cost and power using a "mixture of experts" method, suggest that efficiency and innovation in model design might outpace brute-force scaling. This challenges the tech industry's prevailing strategy, which relies heavily on pouring resources into ever-larger data centers and models, exemplified by Microsoft’s deal to power its infrastructure with a nuclear plant.
The AAAI report, published in March 2025, provides further context. While it doesn’t directly label scaling a "dead end," it emphasizes the need for new research directions beyond current trends. For instance, the "Artificial General Intelligence (AGI)" chapter (not fully provided in your excerpt) would likely elaborate on the survey findings mentioned in the Futurism article, given the overlap in timing and contributors like Stuart Russell. Other sections, such as "AI Reasoning" and "Factuality & Trustworthiness," underscore limitations in current LLMs—like their reliance on plausible rather than guaranteed reasoning and persistent issues with hallucinations—indicating that scaling alone won’t address these fundamental flaws.
However, it’s not a unanimous view that these billions are wasted. The tech industry’s approach has yielded practical successes in generative AI, powering tools used widely in business, creative industries, and more. Companies like Microsoft, Google, and Amazon continue to double down on scaling, suggesting confidence in incremental gains, even if AGI remains elusive. The AAAI report’s "Hardware & AI" chapter might argue that co-designing hardware and algorithms could still unlock efficiencies, though this isn’t detailed in your excerpts.
In summary, many AI researchers, backed by the AAAI survey, argue that the tech industry’s billions are being funneled into a strategy with diminishing returns for achieving AGI, labeling it a potential "dead end." Yet, the immediate commercial benefits and ongoing industry commitment suggest the investments aren’t entirely fruitless—just perhaps misaligned with the long-term goal of AGI. Without a web search to confirm broader trends or counterarguments, this assessment rests on the documents’ portrayal of a research community urging a pivot toward more innovative, less resource-intensive approaches. Would you like me to dig deeper into any specific aspect of this?
Claude’s answer:
Based on the documents you've shared, I can provide a detailed analysis of whether the tech industry is indeed "pouring billions into a dead end" regarding AI development, particularly focusing on the debate around scaling current AI approaches.
The Scaling Debate: What the Research Shows
According to the Futurism article, a recent survey of 475 AI researchers conducted by the Association for the Advancement of Artificial Intelligence (AAAI) found that an overwhelming 76% of respondents believe it's "unlikely" or "very unlikely" that simply scaling up current AI approaches could lead to achieving artificial general intelligence (AGI).
This finding represents a significant rebuff to the tech industry's preferred method of achieving AI gains—primarily by adding more computational power, larger datasets, and more hardware to generative models and the data centers that train and run them.
As Stuart Russell from UC Berkeley explains in the article: "The vast investments in scaling, unaccompanied by any comparable efforts to understand what was going on, always seemed to me to be misplaced. I think that, about a year ago, it started to become obvious to everyone that the benefits of scaling in the conventional sense had plateaued."
The Scale of Investment
The scale of investment in the current approach is staggering:
Generative AI investment reached over $56 billion in venture capital funding alone in 2024
Microsoft has committed to spending $80 billion on AI infrastructure in 2025
Tech giants are signing deals with nuclear power plants just to power their data centers
Signs of Diminishing Returns
Several indicators suggest that the return on these massive investments may be diminishing:
Reports that OpenAI researchers discovered their upcoming GPT model displayed significantly less improvement, and in some cases no improvements at all, compared to previous version upgrades
Google CEO Sundar Pichai acknowledged in late 2023 that "easy AI gains were over"
The Chinese startup DeepSeek demonstrated that it could create AI models competitive with Western flagship models at a fraction of the training cost and power
Alternative Approaches Being Explored
The AAAI report suggests that researchers are exploring more efficient approaches:
Test-time compute: OpenAI has used this method with its latest models, where the AI spends more time to "think" before selecting the most promising solution. This has achieved performance boosts that would otherwise require significant scaling to replicate.
Mixture of experts: DeepSeek pioneered this approach, which leverages multiple neural networks (each specializing in different fields) to help come up with solutions, instead of relying on a single "generalist" model.
The Broader AI Research Perspective
The AAAI's Presidential Panel report provides a more nuanced perspective on the future of AI research. While it acknowledges the scaling challenges, it emphasizes several important themes:
Diversity of approaches is crucial: The report advocates for supporting various AI paradigms beyond just neural networks, noting that "it is important to encourage and support research on a variety of AI paradigms, old and new."
Integration is key: Rather than seeing different approaches as competing, the report suggests that "some of the future breakthroughs will come from other areas, either on their own, or in combination with neural and other classical methods."
Hardware-software co-evolution continues: The report notes that "while numerical solvers rely on sparsity for effective large-scale matrix factorization, similar gains are hard to achieve for arbitrary sparsity patterns in ML models." This suggests that hardware innovations tailored to new algorithmic approaches might offer pathways forward beyond simple scaling.
Conclusion
The evidence from both documents suggests that simply pouring more computing resources into current AI approaches may indeed represent diminishing returns. The consensus among AI researchers indicates that we're reaching the limits of what scaling alone can achieve, and new algorithmic innovations, hybrid approaches, and fundamental rethinking may be necessary to make significant further progress.
However, this doesn't mean all investment in AI is wasted—rather, the research community is pointing toward the need for more diverse and innovative approaches rather than just throwing more computing power at the same methods. The tech industry would be wise to diversify its investments across multiple promising paradigms rather than focusing exclusively on scaling current approaches.
Perplexity’s answer:
According to a recent survey of 475 AI researchers, the tech industry's massive investments in scaling up current AI approaches to achieve artificial general intelligence (AGI) may indeed be pouring billions into a dead end.
Key Findings
76% of surveyed AI researchers believe it is "unlikely" or "very unlikely" that scaling up current AI approaches will lead to AGI.
The benefits of conventional scaling appear to have plateaued, with diminishing returns on massive investments.
Researchers argue that vast investments in scaling without comparable efforts to understand the underlying processes are misplaced.
Industry Investments
Generative AI investment reached over $56 billion in venture capital funding in 2024.
Microsoft has committed to spending $80 billion on AI infrastructure in 2025.
Tech giants like Microsoft, Google, and Amazon are making large investments in energy infrastructure, including nuclear power, to support AI data centers.
Challenges and Alternatives
Recent developments, such as the Chinese startup DeepSeek's AI model matching Western chatbots at a fraction of the cost, challenge the effectiveness of scaling.
OpenAI researchers reported diminishing improvements in the latest GPT model versions.
Alternative approaches being explored include:
Test-time compute: Spending more time on "thinking" before selecting solutions
Mixture of experts: Leveraging multiple specialized neural networks instead of a single generalist model
While the tech industry continues to invest heavily in scaling AI infrastructure, the survey results suggest that this approach may not be the most effective path to achieving AGI. Researchers are calling for more diverse and efficient approaches to advance AI capabilities beyond simply increasing computational power and data volume.
Gemini’s answer:
Yes, the tech industry is pouring billions into a dead end. A survey of 475 AI researchers indicates that the majority of respondents believe that the tech industry's investment in scaling current AI approaches is unlikely to achieve artificial general intelligence (AGI).
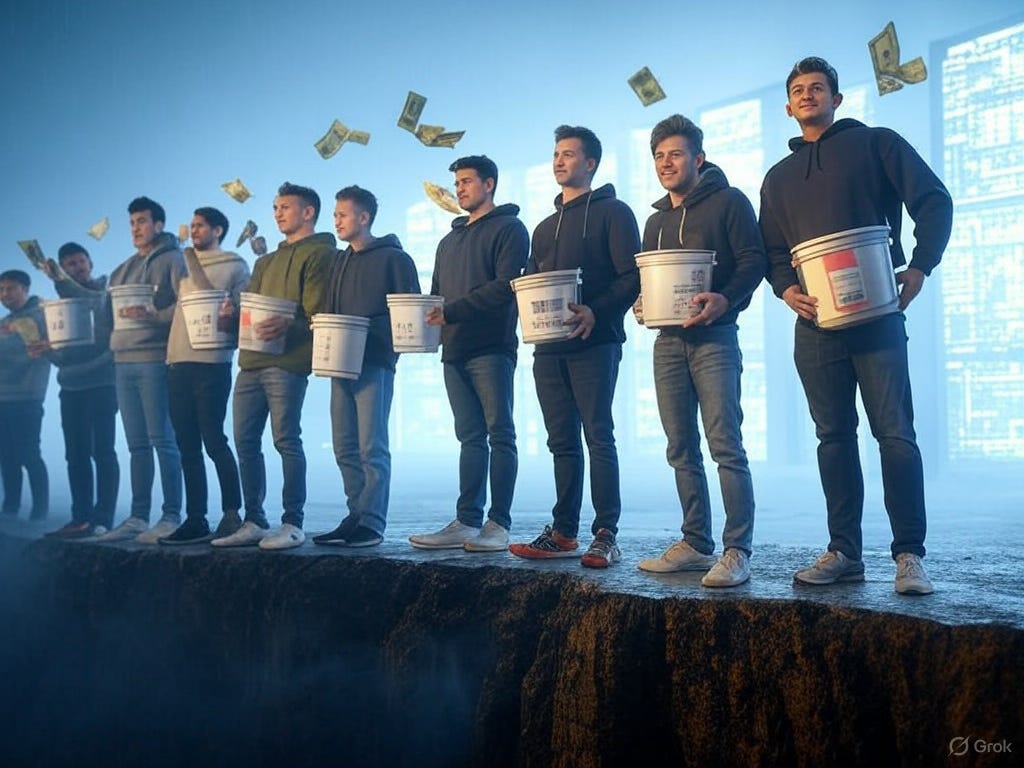