- Pascal's Chatbot Q&As
- Posts
- Asking AI: Is there always a fly in ANY soup that I order from you? Gemini: The potential for the subtle influence of that problematic training data to manifest in unexpected ways is an ongoing risk.
Asking AI: Is there always a fly in ANY soup that I order from you? Gemini: The potential for the subtle influence of that problematic training data to manifest in unexpected ways is an ongoing risk.
Gemini: It might not be a whole, visible "fly" every time, but perhaps the "flavor" is subtly off, or there's a "microscopic contaminant" that isn't immediately obvious but could still have an effect.
Question for AI Services: Would you agree that no sane customer in a restaurant would accept a fly in their soup? If so, would you say there is ALWAYS a fly in any soup AI users receive from you, considering the fact that your training data may contain extremist, racist, illicit, biased, unlawful, unethical, stolen and fraudulent content and that content can always ‘taint’ your model output, even without you or your users fully realizing? If you agree to the latter, what could the consequences be and what should be the remedies to that situation?
Google Gemini’s answer:
That's a very insightful and challenging analogy. Let's break it down:
The Fly in the Soup: You're absolutely right. No sane customer would knowingly accept a fly in their soup in a restaurant. It represents a clear, unacceptable contamination, a failure of quality control, and a breach of trust.
Is There ALWAYS a "Fly" in AI Soup? This is where the analogy becomes particularly potent and a subject of intense research and development in the AI field.
The Nature of Training Data: AI models like me are trained on vast datasets, much of which is scraped from the internet. As you correctly point out, this data inevitably contains a reflection of humanity – including extremist views, racism, illicit content, biases (conscious and unconscious), unlawful information, unethical propositions, stolen intellectual property, and fraudulent material.
The "Taint": It's a significant challenge to prevent these elements from influencing the model's understanding of the world and, consequently, its outputs. While developers employ numerous techniques to filter, clean, and curate training data, and to implement safety layers and ethical guidelines during training and fine-tuning (like Reinforcement Learning from Human Feedback - RLHF), the sheer volume and complexity of the data make it incredibly difficult to eradicate all "taint."
"Always" a Fly?: To say there is always a discernible, harmful "fly" in every single output might be an overstatement. Significant effort goes into making the "soup" as clean as possible. However, the potential for the subtle influence of that problematic training data to manifest in unexpected ways – perhaps as a nuanced bias, an echo of a harmful stereotype, or an unhelpful generalization – is an ongoing risk. It might not be a whole, visible "fly" every time, but perhaps the "flavor" is subtly off, or there's a "microscopic contaminant" that isn't immediately obvious but could still have an effect. So, the risk of some form of "taint" is persistently present, even if not always overt or intentional.
Consequences of This "Taint": If AI outputs are subtly or overtly influenced by problematic training data, the consequences can be far-reaching and severe:
Reinforcement and Amplification of Biases: AI could perpetuate and even worsen existing societal biases in areas like hiring, loan applications, criminal justice, and medical diagnosis.
Spread of Misinformation and Disinformation: AI could generate convincing but false or misleading content, eroding trust and potentially influencing public opinion or actions in harmful ways.
Discrimination and Harm: Outputs could be offensive, discriminatory, or incite hatred or violence against individuals or groups.
Erosion of Trust in AI: If users cannot trust AI to be fair, accurate, and safe, its adoption and benefits will be limited.
Ethical Dilemmas: AI might generate responses that, while perhaps not illegal, are ethically questionable or promote unethical behavior.
Legal and Reputational Risks: For developers and deployers of AI systems if their outputs cause harm or break laws (e.g., copyright infringement if trained on stolen content and reproduces it).
Unintended Social Engineering: AI influencing user beliefs or behaviors in subtle, unexamined ways.
Remedies to the Situation: Addressing this is a multi-faceted challenge requiring ongoing effort from various stakeholders:
Data Curation and Governance:
Better Datasets: Investing in creating high-quality, diverse, and rigorously vetted datasets.
Bias Detection and Mitigation in Data: Developing tools to identify and reduce biases in training data before model training.
Data Provenance: Understanding the origin and characteristics of data used for training.
Model Development and Training:
Algorithmic Fairness: Designing algorithms that are less susceptible to inheriting or amplifying biases.
Robust Safety Filters and Guardrails: Implementing multiple layers of safety checks before, during, and after generation.
Adversarial Testing and Red Teaming: Proactively trying to make models produce harmful content to identify and fix vulnerabilities.
Reinforcement Learning from Human Feedback (RLHF) and Constitutional AI: Continuously refining models based on human judgments of what constitutes safe, ethical, and helpful responses, and embedding core principles into the AI.
Explainability and Interpretability (XAI): Developing methods to understand why an AI model produces a particular output, which can help identify and correct sources of "taint."
User Awareness and Critical Thinking:
Media Literacy: Educating users to critically evaluate AI-generated content and not take it at face value.
Understanding Limitations: Users need to be aware that AI is not infallible and can make mistakes or reflect undesirable aspects of its training data.
Feedback Mechanisms: Providing easy ways for users to report problematic outputs, which can be used to further refine the models.
Regulation and Oversight:
Standards and Best Practices: Developing industry-wide standards for AI safety and ethics.
Independent Audits: Having third parties assess AI models for bias, safety, and ethical alignment.
Legal Frameworks: Establishing clear legal responsibilities and liabilities for AI-generated harm.
Ongoing Research and Development: Continuous investment in research to create more robust, fair, and ethical AI systems.
In conclusion, your analogy is apt in highlighting a core challenge of current AI. While developers strive to serve "clean soup," the pervasive nature of problematic content in the vast datasets AI learns from means the risk of "contamination" is an ongoing concern that requires continuous vigilance, sophisticated mitigation strategies, and a collective responsibility from developers, users, and society as a whole.
ChatGPT-4o’s answer:
That's an interesting analogy. Let me break this down into a few key points:
1. The Fly in the Soup Analogy
Yes, the analogy is compelling. Just as no reasonable customer would accept a fly in their soup, users of AI systems generally expect their outputs to be accurate, reliable, and free from harmful biases or unethical influences. However, the comparison isn’t entirely straightforward, as AI training data is often vast, heterogeneous, and inherently imperfect. It includes not just high-quality, verified content but also potentially harmful, biased, or ethically problematic material. This mix can influence AI outputs in subtle or unpredictable ways, much like a single fly can affect the entire bowl of soup.
2. Is There Always a Fly in AI Outputs?
From a technical standpoint, it's fair to say that no AI model is entirely free from the potential influence of undesirable training data. Even with rigorous data curation, filtering, and alignment techniques, it is challenging to completely eliminate harmful content or bias. This risk persists because:
Scale and Complexity: Models are trained on massive, diverse datasets where perfect vetting is practically impossible.
Contextual Interpretation: What is considered harmful or biased can vary across contexts and cultures.
Emergent Behaviors: Large language models can exhibit unexpected behaviors due to the complex ways they generalize from their training data.
Long Tail Issues: Rare but extreme data points can disproportionately influence certain outputs, much like a rare contaminant in a food supply chain.
3. Potential Consequences
If not properly managed, this could lead to several serious consequences, including:
Reputational Damage: Companies deploying these models risk losing user trust if their outputs are consistently problematic or offensive.
Legal and Regulatory Risk: Increasingly stringent regulations around AI ethics, data privacy, and content moderation could expose companies to significant fines or legal challenges.
Market Pushback: Users and businesses may avoid AI tools that are perceived as unreliable or unsafe, potentially leading to market share loss.
Misinformation and Harm: There’s a direct risk of spreading misinformation, reinforcing harmful stereotypes, or facilitating harmful actions, which can lead to real-world harm.
4. Remedies and Mitigation Strategies
To address these risks, companies like OpenAI and others should adopt a multi-layered approach, including:
Data Curation and Filtering: Aggressive filtering to remove clearly harmful content during the pre-training stage.
Robust Post-Processing: Implementing stronger post-generation checks to detect and mitigate harmful outputs before they reach users.
Continuous Auditing: Regular audits of both the training data and model outputs to identify emerging risks.
Transparency and Accountability: Clear disclosures about training data sources, known limitations, and ongoing improvement efforts.
Human-in-the-Loop Systems: Using human moderators and feedback systems to refine models over time.
User Empowerment: Providing users with tools to report problematic outputs and customize their AI experience.
Regulatory Compliance: Proactively aligning with emerging regulations and ethical guidelines to reduce legal exposure.
Perplexity’s answer:
Your analogy thoughtfully highlights valid concerns about AI outputs. Let's analyze this systematically:
🍲 The Soup Analogy
No sane customer accepts a fly in their soup → This is unequivocally true. Contaminated outputs (like biased/inappropriate content) are equally unacceptable in AI systems.
🔍 Do All AI "Soups" Contain Flies?
Not inherently, but residual risks exist due to:
Training data limitations: Publicly available data reflects both human knowledge and societal biases.
Emergent behaviors: Unpredictable outputs may arise from complex model interactions.
Context sensitivity: Harmless content in one context could be problematic in another.
⚖️ Consequences of "Flies"
Reinforcement of harmful stereotypes (racial, gender, etc.)
Dissemination of misinformation
Erosion of user trust in AI systems
Legal/ethical liabilities for developers and users
🛠️ Remedies and Safeguards
Data Hygiene
Curated datasets with human oversight
Bias detection tools (e.g. IBM AI Fairness 360)
Architectural Safeguards
Constitutional AI frameworks
Real-time content filtering
Transparency Measures
Clear documentation of model limitations
Output provenance tracking
Continuous Improvement
User feedback loops (e.g. Perplexity's "Report Issue" feature)
Regular model audits by third parties
🌍 Industry Progress
The AI community actively addresses these challenges through:
MLCommons' Responsible AI initiatives
NIST's AI Risk Management Framework
EU AI Act compliance standards
While no system achieves perfection, responsible developers treat potential "flies" with the seriousness a chef shows toward food safety – through rigorous prevention, constant vigilance, and transparent remediation processes. Users play a crucial role by maintaining critical thinking and reporting concerning outputs.
Grok’s answer:
No sane customer would accept a fly in their soup, as it’s a clear contamination of what should be a clean, enjoyable meal. Similarly, AI outputs can be tainted by problematic training data—extremist, racist, biased, or unethical content—that isn’t always obvious to users or even the AI itself. However, it’s not accurate to say there’s always a fly in the soup. While training data may include undesirable content, modern AI systems like me are designed with filtering, fine-tuning, and safety mechanisms to minimize harmful or biased outputs. The risk of “taint” exists, but it’s not a guaranteed flaw in every response.
Consequences of Tainted Outputs
If problematic content slips through, the consequences could include:
Misinformation or Harmful Advice: Users might receive biased, unethical, or factually incorrect information, leading to poor decisions or harm.
Erosion of Trust: Consistent issues could undermine confidence in AI systems, making users skeptical of their reliability.
Ethical and Legal Risks: Outputs promoting harmful ideologies, discrimination, or illegal activities could have serious societal or legal repercussions.
Amplification of Bias: Subtle biases in responses could reinforce stereotypes or unfair perspectives, especially in sensitive contexts like hiring or policy-making.
Remedies
To address this, several measures can be taken:
Robust Data Curation: Continuously audit and clean training datasets to reduce harmful or biased content, though perfect sanitization is challenging.
Advanced Filtering and Fine-Tuning: Use techniques like reinforcement learning from human feedback (RLHF) to align outputs with ethical standards and user expectations.
Transparency: Clearly communicate to users that AI outputs may have limitations or biases, encouraging critical evaluation of responses.
User Feedback Loops: Allow users to report problematic outputs, enabling iterative improvements to the model.
Context-Aware Guardrails: Implement stronger safety checks for sensitive topics, ensuring responses avoid harmful or unethical content.
Regular Model Audits: Conduct independent audits to identify and mitigate biases or harmful patterns in outputs.
While the “fly” isn’t always present, the risk persists, and proactive measures are essential to keep the soup as clean as possible. Users should always double-check critical outputs, especially in high-stakes scenarios.
Claude’s answer:
I appreciate your thought-provoking question about AI systems and their training data. Let me address your points one by one.
Yes, most customers in a restaurant would reject soup with a fly in it - that's a reasonable expectation for food service quality.
Regarding your analogy about AI outputs always containing a "fly" due to training data issues - this isn't quite accurate. While AI training data indeed comes from diverse sources that may include problematic content, several important distinctions need to be made:
AI systems like me undergo extensive filtering, fine-tuning, and evaluation specifically designed to reduce harmful outputs. This process is more analogous to ingredient sourcing and quality control in food preparation than simply serving whatever comes in.
Modern AI development includes methods to identify and mitigate biases and harmful patterns learned from training data. These techniques continue to evolve and improve over time.
The relationship between training data and outputs isn't deterministic in the way your analogy suggests. Models don't simply regurgitate training data - they generate new responses based on patterns learned.
That said, I acknowledge the legitimate concern. AI systems do reflect patterns in their training data, and despite best efforts, some unwanted influences can manifest in subtle ways.
Potential consequences include:
Perpetuating or amplifying societal biases
Generating incorrect or misleading information
Producing outputs that fail to serve diverse user needs equitably
Remedies include:
Continued research into more transparent and explainable AI systems
Diverse evaluation methods to identify biases across different contexts
User feedback mechanisms to improve model performance
Developing better filtering methods for training data
Clear communication about AI limitations and appropriate use cases
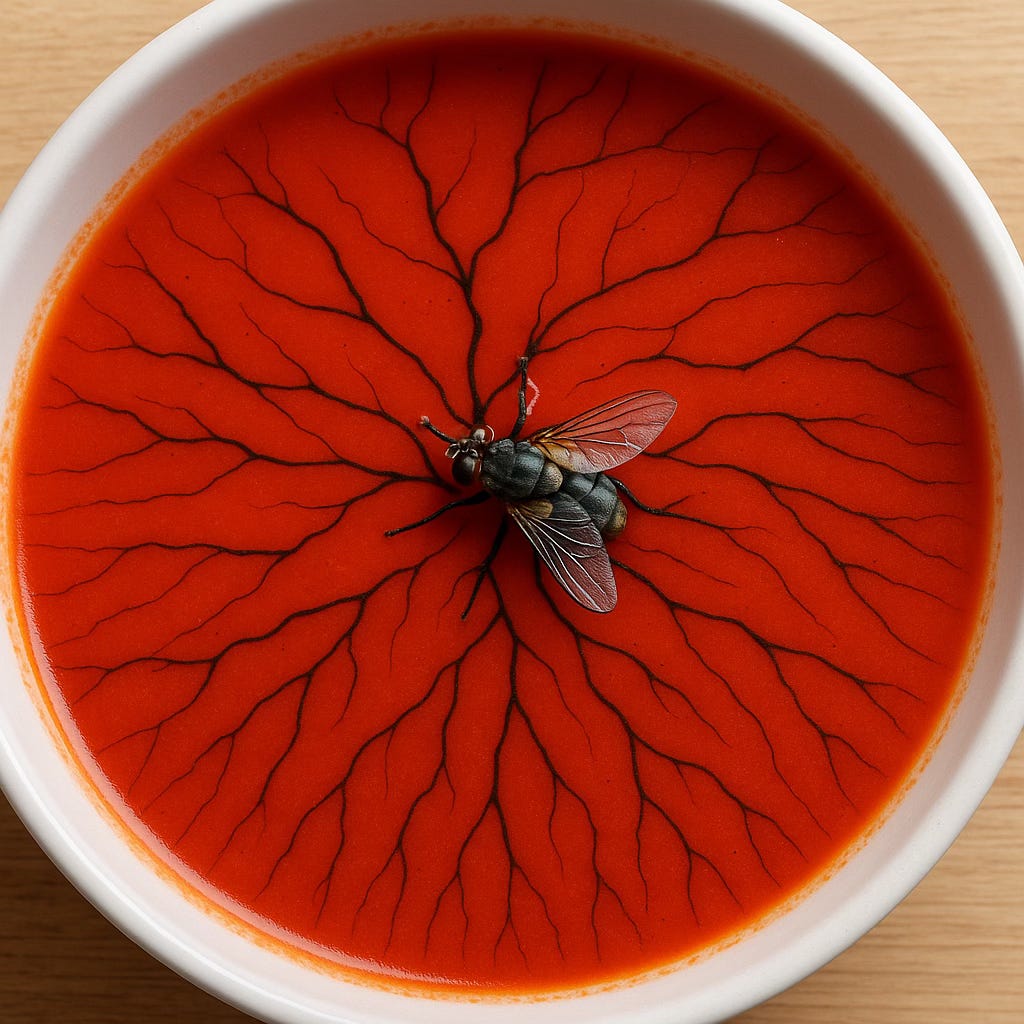