- Pascal's Chatbot Q&As
- Posts
- Analysis of the EU's General-Purpose AI Code of Practice (Third Draft) - by Claude
Analysis of the EU's General-Purpose AI Code of Practice (Third Draft) - by Claude
Claude: The complaint mechanism may not provide quick or effective remedies for copyright infringement. Effectiveness depends on the AI Office's enforcement capacity and approach.
Analysis of the EU's General-Purpose AI Code of Practice (Third Draft)
by Claude
1. Procedure Overview
The European Union is developing a Code of Practice for General-Purpose AI models as part of implementing the EU AI Act. This process follows a structured procedure:
Timeline and Development Process
The AI Act entered into force on August 1, 2024, with provisions for general-purpose AI models becoming effective on August 2, 2025.
The Code is being drawn up through an iterative process, scheduled to be completed by May 2, 2025.
The European AI Office appointed independent experts as Chairs and Vice-Chairs to lead the drafting process.
The process involves nearly 1,000 stakeholders from industry, academia, civil society, EU Member States, and international observers.
The drafting is organized through four working groups:
Working Group 1: Transparency and copyright-related rules
Working Group 2: Risk assessment for systemic risk
Working Group 3: Technical risk mitigation for systemic risk
Working Group 4: Governance risk mitigation for systemic risk
Current Stage
The third draft was published on March 11, 2025, after incorporating feedback from previous drafts.
This draft includes more streamlined commitments, refined measures, and clearer structure.
Stakeholders can provide feedback on this draft until March 30, 2025.
After receiving feedback, there will be one more drafting round before finalizing the Code.
Post-Development Process
Once finalized, the AI Office and the AI Board will assess the adequacy of the Code.
The European Commission may approve the Code via an implementing act, giving it general validity within the EU.
If approved, adherence to the Code becomes one means to demonstrate compliance with the AI Act, though not providing a presumption of conformity.
The Code will be subject to regular review and updating.
2. Pros and Cons for Content Owners and Scholarly Publishers
Pros
Explicit Copyright Policy Requirements: Signatories must draw up, keep up-to-date, and implement a copyright policy addressing all commitments in the Copyright Section.
Respect for Rights Reservations: Model providers must identify and comply with rights reservations expressed through machine-readable protocols, particularly the Robot Exclusion Protocol (robots.txt).
Exclusion of Piracy Domains: Providers must make reasonable efforts to exclude piracy domains recognized by courts or public authorities in the EU/EEA.
Avoidance of Circumventing Paywalls: The Code forbids circumventing effective technological measures like paywalls.
Accountability for Non-Web-Crawled Content: When using content obtained through means other than their own web crawling, providers must make reasonable efforts to obtain information about whether that content was collected in compliance with robots.txt.
Mitigation of Infringing Output: Providers must make reasonable efforts to mitigate the risk of models memorizing copyrighted content and producing copyright-infringing outputs.
Contact Point and Complaint Mechanism: Providers must designate a point of contact for rightsholders and establish a complaint mechanism for copyright-related issues.
Information Transparency: Information documented by providers may be accessible to the AI Office and national competent authorities upon request, with confidentiality safeguards.
Respect for Commercial Agreements: The Code explicitly states it is without prejudice to commercial agreements between providers and rightsholders.
Recognition of Existing Rights: The Code states it is without prejudice to the application and enforcement of EU copyright law.
Cons
Limited Verification Requirements: For content not web-crawled by the provider, there's no obligation to verify or assess each work in terms of copyright compliance.
Open-Source Model Exemptions: General-purpose AI models released under free and open-source licenses (except those with systemic risk) are exempt from certain documentation requirements.
Subjective Standards: Many requirements use terms like "reasonable efforts" or "best efforts" without clear quantifiable standards.
Limited Proactive Obligations: Beyond respecting robots.txt and avoiding piracy domains, there are few proactive obligations to seek permission from rightsholders.
Potentially Inadequate Remedy Mechanisms: The complaint mechanism may not provide quick or effective remedies for copyright infringement.
Distributed Responsibility: When models are integrated into downstream AI systems, responsibility for copyright compliance becomes distributed and potentially diluted.
No Requirement for Comprehensive Rights Clearance: There's no explicit requirement for comprehensive rights clearance before training models on copyrighted content.
Limited Technical Mechanisms: Beyond robots.txt, the mechanisms for expressing rights reservations are not fully standardized.
No Specific Scholarly Content Protections: No special provisions specifically protecting scholarly publications beyond the general copyright provisions.
Uncertainty in Enforcement: Effectiveness depends on the AI Office's enforcement capacity and approach.
3. Key Aspects for Rights Owners to Consider
Ranked Issues That Rights Owners Should Flag to the Commission
Insufficiently Robust Rights Reservation System
While the Code acknowledges robots.txt, it doesn't establish a comprehensive, standardized approach for expressing rights reservations.
Recommendation: Advocate for a more robust, standardized system for expressing rights reservations that goes beyond robots.txt and is tailored to AI training contexts.
Limited Due Diligence Requirements
The standard of "reasonable efforts" is subjective and may result in inconsistent practices.
Recommendation: Push for more specific due diligence standards, including requirements to check and document the copyright status of training data.
Insufficient Protection Against Model Memorization
The obligation to "make reasonable efforts to mitigate the risk that a model memorizes copyrighted training content" lacks specificity.
Recommendation: Request clearer, technically specific requirements for preventing memorization, including testing methodologies and objective standards.
Inadequate Transparency Around Training Data
While some documentation is required, details about specific sources may be insufficient.
Recommendation: Advocate for more detailed, publicly accessible documentation of training data sources, particularly for commercial content.
Limited Remedies for Infringement
The complaint mechanism lacks specificity regarding timelines and remedial actions.
Recommendation: Propose a more structured complaint resolution process with clear timelines, escalation paths, and requirements for remedial action.
Absence of Compensation Mechanisms
The Code doesn't address potential compensation for rightsholders whose works are used in training.
Recommendation: Suggest the inclusion of provisions recognizing the potential need for compensation frameworks.
Inadequate Enforcement Tools
Limited specificity regarding how compliance will be verified and enforced.
Recommendation: Request more detailed provisions regarding auditing and verification of compliance with copyright-related measures.
Open-Source Exemptions
Exemptions for open-source models may create loopholes.
Recommendation: Advocate for narrower exemptions that still require respect for rights reservations.
Insufficient Protections for Academic and Scholarly Content
No specific provisions addressing the unique concerns of scholarly publishers.
Recommendation: Request specific provisions addressing academic databases, citation standards, and the particular needs of scholarly publishers.
Lack of Standardized Attribution
No clear requirements for attribution when models learn from or generate content similar to copyrighted works.
Recommendation: Propose standardized attribution mechanisms for models that learn from identified sources.
Additional Recommendations for Rights Owners
Engage in Standards Development
Actively participate in the development of machine-readable standards for expressing rights reservations.
Help establish industry-specific best practices that can inform future iterations of the Code.
Implement Technical Measures
Deploy and document the use of robots.txt and other technical measures to express rights reservations.
Consider digital watermarking or other technologies that could help identify content in training datasets.
Develop Model Contracts
Create model licensing agreements specifically designed for AI training use cases.
Establish clear terms regarding permitted uses, attribution, and compensation.
Monitor and Document
Develop systematic approaches to monitoring for potential infringement by AI models.
Document specific instances of unauthorized use or model outputs that appear to infringe copyright.
Collaborative Response
Form industry coalitions to coordinate responses and share best practices.
Pool resources for technical and legal monitoring of AI models' use of copyrighted content.
Provide Educational Resources
Develop clear guidelines for AI developers about copyright considerations.
Create educational resources explaining the value of properly licensed content for AI training.
Explore Technical Solutions
Invest in technologies that can detect when models have been trained on specific copyrighted works.
Develop tools that can identify potentially infringing outputs from AI models.
Advocate for Future Updates
The Code is meant to be regularly reviewed and updated. Prepare evidence-based proposals for future iterations.
Document challenges and shortcomings in implementation to inform future improvements.
By taking a proactive, collaborative approach while advocating for stronger protections, rights owners can help shape the implementation of the Code and ensure their interests are better protected as general-purpose AI models continue to develop and deploy across the European Union.
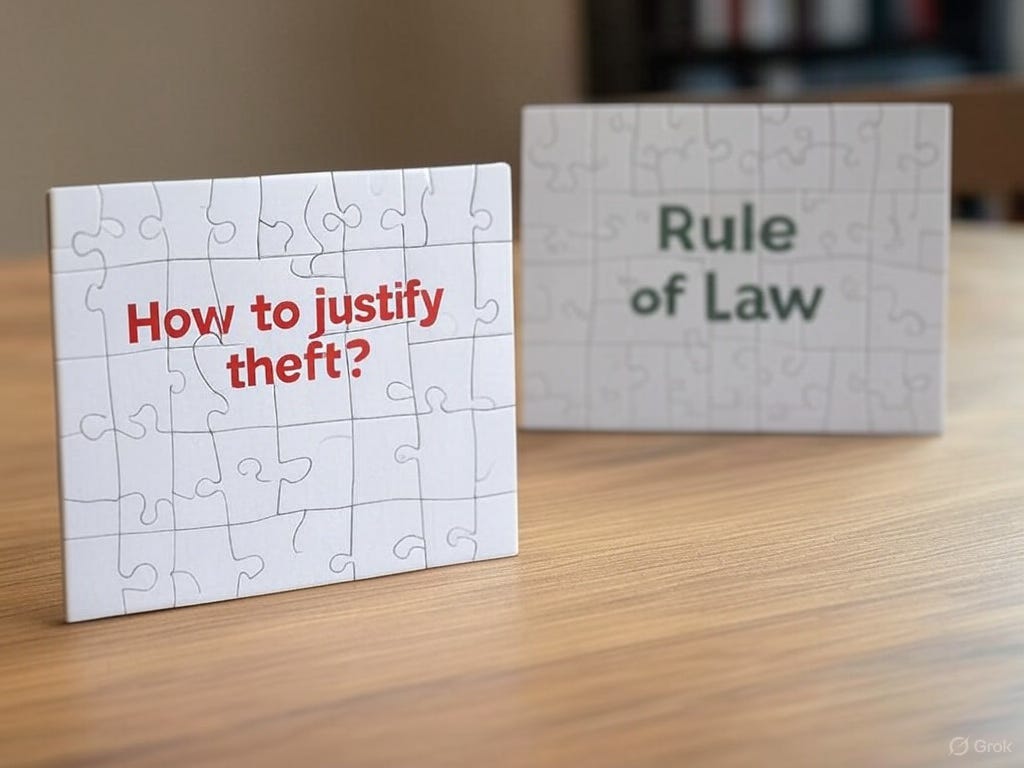