- Pascal's Chatbot Q&As
- Posts
- AI, particularly GenAI and LLMs, represents the next significant transformation catalyst for the scholarly publishing industry, following the shift from print to digital.
AI, particularly GenAI and LLMs, represents the next significant transformation catalyst for the scholarly publishing industry, following the shift from print to digital.
The evolution of knowledge access itself, moving away from static document consumption towards dynamic, interactive, and personalized knowledge exploration, driven by changing user expectations.
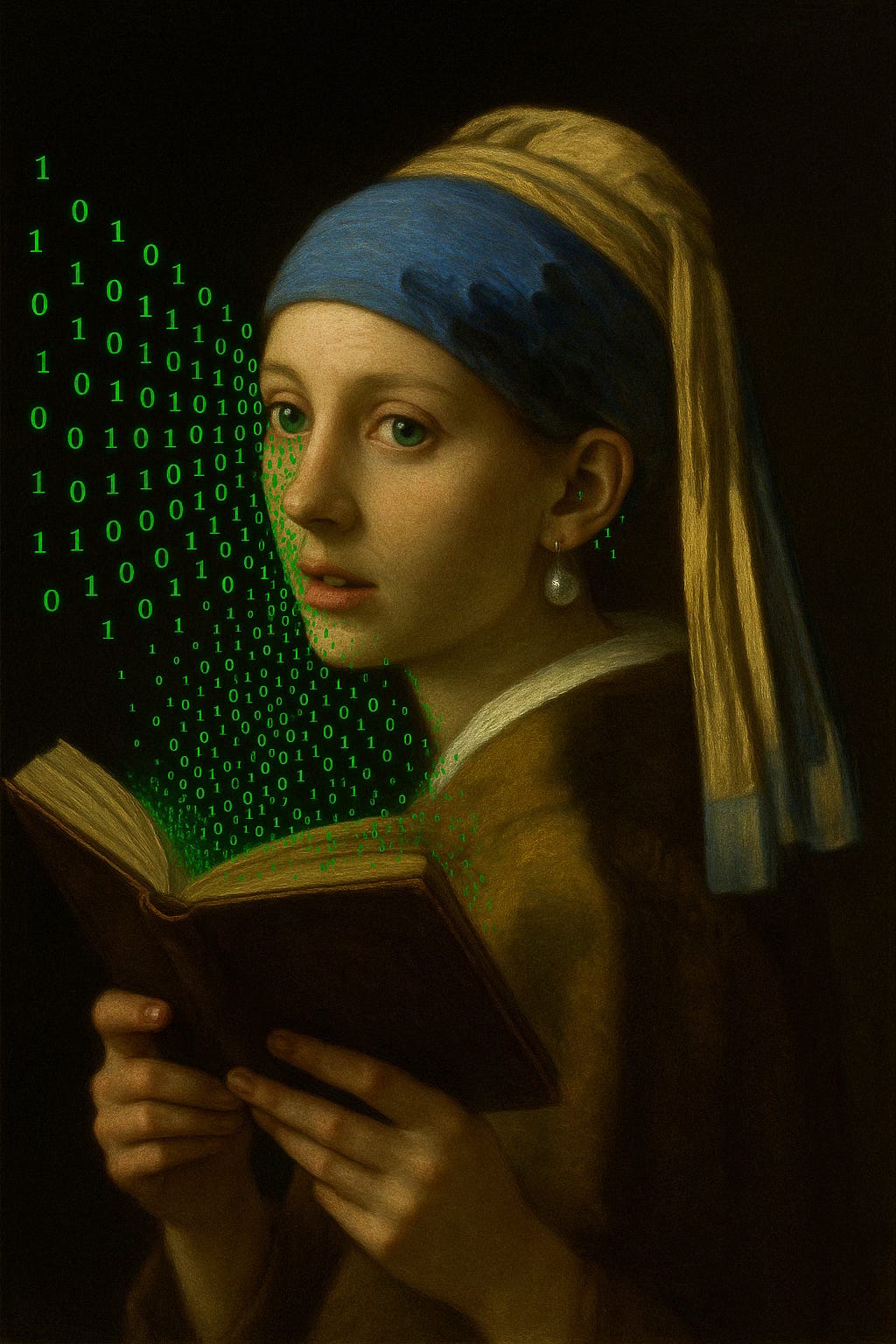
by Gemini Advanced, Deep Research with 2.5 Pro. Warning: LLMs may hallucinate!
Executive Summary:
Artificial intelligence (AI), particularly generative AI (GenAI) and Large Language Models (LLMs), represents the next significant transformation catalyst for the scholarly publishing industry, following the shift from print to digital. This technology presents a duality: immense opportunities for enhancing efficiency, accelerating discovery, and creating new user experiences, counterbalanced by substantial challenges including ethical dilemmas, copyright complexities, potential business model disruption, and the risk of propagating misinformation.1 The rapid emergence of tools like ChatGPT underscores the speed and scale of this change.3 Central to this transformation is the evolution of knowledge access itself, moving away from static document consumption towards dynamic, interactive, and personalized knowledge exploration, driven by changing user expectations and AI capabilities.4 This report analyzes the current state of AI adoption in scholarly publishing, explores its potential to revolutionize knowledge access and dissemination, examines the critical challenges surrounding trust, ethics, and copyright, predicts the future trajectory of the ecosystem, and concludes with strategic imperatives for publishers seeking to maintain relevance and ensure business continuity in an increasingly AI-integrated world.
1. Introduction: AI as the Next Transformation Catalyst in Scholarly Publishing
Scholarly publishing has historically navigated significant technological shifts, most notably the transition from print-centric models to digital platforms. Now, the industry stands at the cusp of another profound transformation, driven by the rapid advancement and proliferation of artificial intelligence (AI), especially generative AI (GenAI) and the underlying Large Language Models (LLMs).2 The meteoric rise in public awareness and usage, exemplified by tools like ChatGPT achieving unprecedented user adoption rates 3, signals that AI is not a peripheral trend but a core force reshaping information ecosystems.
For scholarly publishers, AI presents a complex landscape of both opportunity and peril. On one hand, it offers the potential for significant efficiency gains across the entire publishing lifecycle, from authoring and peer review to production and discovery.2 It promises to accelerate the pace of scientific discovery itself through AI-enhanced research methodologies.2 On the other hand, AI introduces formidable challenges. Ethical considerations surrounding bias, transparency, and accountability are paramount.7 The use of copyrighted content for training AI models has ignited complex legal battles.9 Existing business models face potential disruption as AI alters how users interact with information.1 Furthermore, the inherent capabilities of current AI systems raise concerns about the potential for generating inaccurate or misleading information, a critical risk in the context of scholarly communication.12
A central theme of this transformation, and a key focus of this report, is the evolution of knowledge access. User expectations, increasingly shaped by consumer AI experiences, are shifting towards immediacy, ease of use, and personalization.4 The traditional model of linear consumption – reading individual articles or static documents – is being challenged by a demand for synthesized insights, direct answers, and interactive exploration. AI technologies, including conversational interfaces and autonomous agents, are poised to meet and further shape these expectations, fundamentally altering how scholarly knowledge is discovered, accessed, synthesized, and utilized.
This report aims to provide a strategic analysis of this evolving landscape. It will begin by mapping the current applications of AI within scholarly communication, drawing on industry research and specific publisher perspectives. It will then delve into how AI, particularly through agentic systems and new interaction paradigms, is set to revolutionize knowledge access and dissemination. Subsequently, the report will critically examine the intertwined challenges of responsible AI implementation, ensuring the veracity of AI-generated content, and navigating the complex copyright issues. Based on this analysis, the report will predict the likely future trajectory of the scholarly publishing ecosystem in an AI-integrated world, considering potential incremental and disruptive scenarios. Finally, it will conclude with actionable strategic recommendations for scholarly publishers aiming to adapt, innovate, and secure their relevance and business continuity in this dynamic future.
2. The Evolving Landscape: AI's Current Foothold in Scholarly Communication
The integration of AI into scholarly publishing is rapidly moving beyond tentative experimentation towards more focused, strategic implementation driven by the pursuit of tangible returns on investment.4 While 2024 saw significant testing and adaptation of AI tools to fit existing processes, the trajectory points towards 2025 as a year where AI begins to fundamentally reshape and redefine publishing workflows entirely.4Already, generative AI has established a noticeable presence, with estimates suggesting that a small but growing percentage of scholarly literature shows signs of LLM assistance, and major publishers actively deploying AI-enhanced tools.2
Mapping Current AI Applications
The application of AI currently spans multiple stages of the scholarly communication lifecycle:
Search and Discovery Enhancement: Traditional keyword search is evolving. AI powers semantic search engines that understand user intent better, delivering more relevant results (e.g., Google Scholar enhancements, Semantic Scholar 13). Publishers offer AI-driven journal recommendation tools (e.g., Springer Nature Journal Suggester, Wiley Journal Finder, Elsevier's Journal Finder, Taylor & Francis' Journal Suggester) that match manuscripts to suitable venues based on content analysis.14 Tools like Scite.ai help researchers find related work and assess supporting or contrasting evidence.15 Furthermore, major publishers and content aggregators are releasing AI-enhanced discovery tools 2, and the industry anticipates a move towards conversational interfaces capable of synthesizing findings across papers and answering specific research questions with citations, mirroring user experiences with tools like ChatGPT.4
Peer Review and Editorial Support: AI is increasingly used to augment the peer review process, a notoriously stressed component of scholarly publishing.2 While AI is not seen as a replacement for human reviewers 4, it offers significant potential for efficiency gains. Applications include generating manuscript summaries for reviewers 4, suggesting suitable peer reviewers based on expertise and content matching (e.g., Clarivate's Reviewer Locator, Elsevier's Reviewer Finder 14), proposing alternative journals if a manuscript is a better fit elsewhere 4, performing initial quality checks for plagiarism or image duplication using tools like iThenticate or Turnitin 15, checking statistical reporting (e.g., StatCheck 15, StatReviewer 14), assessing methods sections for reproducibility and transparency (e.g., Ripeta 15, SciScore 14), and automating various routine editorial tasks like sending emails or linking references.5
Authoring and Manuscript Preparation: Authors are utilizing AI for various writing and preparation tasks. AI-powered writing assistants (e.g., Grammarly, Hemingway 17) provide feedback on grammar, style, and clarity. LLMs like ChatGPT are increasingly used for drafting text 18, although publisher policies often require disclosure and emphasize author responsibility.8 AI tools can automate abstract generation (e.g., Alviss 15), improve language, particularly for non-native English speakers 18, assist with data visualization and analysis 17, and streamline reference management.6
Content Accessibility and Transformation: AI is being employed to make complex research more accessible to broader audiences.4 This includes generating simplified summaries, lay summaries suitable for the general public, translations into different languages, and alternative formats like video summaries or infographics.4 Tools like Audemic create audio summaries of research papers.15AI can also produce different versions of content tailored for specific audiences, such as experts or students.4
Research Integrity: Maintaining trust is paramount, and AI tools are being developed and deployed to uphold research integrity. This includes AI-powered systems for detecting image manipulation or duplication 15, a growing concern for publishers. Major publishers like Elsevier, Wiley, Springer Nature, and Taylor & Francis, are working towards developing automated, standardized tools for image integrity checks, potentially mirroring systems like CrossCheck used for plagiarism detection.16 AI is also seen as a potential tool to combat systematic misconduct, such as identifying papers originating from fraudulent "paper mills".16
Marketing and Customer Experience: Marketing and customer experience teams were early adopters of AI.4 AI enables more sophisticated, data-driven approaches to customer engagement, campaign optimization, and understanding user behavior, moving beyond simple automation.4
Insights from the Field: Publisher Perspectives
Several scholarly and news publishers are approaching the AI transformation strategically:
Early Licensing Leadership & Data Value: Some publishers position themselves as early movers in licensing its their repositories to AI companies. This proactive stance recognized the immense value of high-quality, curated scholarly content for training AI models, particularly LLMs. This aligns with the burgeoning market for AI datasets and licensing, where academic content is highly sought after.23The scale of this market is significant, estimated at nearly USD 368 million in 2024 and projected to grow rapidly.23 Early engagement underscores the strategic importance publishers place on their content assets in the AI era. The very act of licensing signifies a recognition that while AI models can be built by tech companies, the quality and reliability of those models, especially for specialized domains like scientific research, depend heavily on the curated knowledge held by publishers. This creates a potentially lucrative B2B market but also establishes a new form of dependency for AI developers on content providers.
Strategic Focus on Responsible AI: A core pillar of publishers’ strategies is a commitment to "responsible AI". This is not merely an ethical stance but a strategic one, focusing on three key principles:
Transparency: Emphasizing clarity around the provenance and licensing status of training data. This addresses major industry concerns and legal challenges surrounding copyright.9
Explainability: Investing effort in understanding how AI models arrive at their outputs. This is crucial for building trust, particularly in high-stakes scientific contexts.24
Human Intervention: Maintaining human oversight and control through protocols, filters, labeling, and reinforcement learning. This acknowledges the limitations of current AI and the necessity of human judgment, especially for sensitive applications.8 This focus aligns with broader industry movements and guidelines from bodies like COPE, which stress transparency and accountability.7 By championing responsible AI, publishers aim to build trust with researchers and differentiate themselves in a market grappling with ethical and reliability issues.
Acknowledging Current AI Limitations: Publishers holds pragmatic views of current LLM capabilities, deeming them not yet sufficient for critical applications involving recommendations, diagnosis, or advice in sectors like science, finance, legal, and healthcare. This assessment stems from concerns about the reliability and factual accuracy of LLM outputs. However, this skepticism is paired with a belief in future improvements through the combination of new AI techniques with older, established technologies like dictionaries and databases to optimize outputs and better understand prompts. This nuanced perspective avoids hype while positioning the publisher to leverage more mature AI capabilities as they emerge.
Concerns about Factual Accuracy and Bias: A significant concern voiced is the unreliability of LLMs regarding factual information. The potential for models to cite fraudulent or retracted research, or for different LLMs to provide contradictory answers on factual matters, is seen as a major impediment to their use in rigorous scientific research. This resonates with widespread observations of AI "hallucinations" and the critical need for verification.7 Some critics flag the potential for bias, likening some LLMs to partisan news outlets, rendering them unsuitable for objective scientific inquiry. This underscores the importance of training data quality and the need for robust validation mechanisms.
Internal AI Strategy Structure: Several news and scholarly publishers have organized their AI initiatives into verticals focused on: Governance (setting internal usage policies and guidelines), Licensing (managing content licensing agreements with tech companies and academic partners), and AI Development (developing public-facing AI tools and services built on their content). This structured approach reflects a sophisticated understanding of AI's multifaceted impact. It recognizes that managing internal use, leveraging content assets for external revenue, and building user-facing products require different expertise, resources, and strategic focus. This organizational design likely provides a framework for navigating the complexities of AI implementation across different business functions and market segments.
Vision for Future Knowledge Access: The fundamental ways people learn and access knowledge are poised for dramatic change. There's an anticipation of a shift away from consuming static documents towards more interactive, engaging, and potentially AI-mediated forms of knowledge consumption. This forward-looking perspective informs publishers’ strategic interest in exploring new AI-driven services and platforms.
The rapid development and deployment of specialized AI tools across the publishing workflow—from news and journal suggestions 14 to integrity checks 15 and author support 17—indicate a maturation beyond general-purpose LLMs. The industry is moving towards a suite of targeted solutions addressing specific pain points, suggesting a deeper integration of AI into the fabric of scholarly communication rather than its use as a simple overlay.
3. Revolutionizing Knowledge Access and Dissemination
Beyond optimizing existing workflows, AI holds the potential to fundamentally reshape how knowledge is discovered, accessed, synthesized, and disseminated. This involves a paradigm shift away from traditional, static content consumption towards more dynamic, interactive, and personalized knowledge experiences, facilitated by emerging AI technologies like autonomous agents and federated systems.
Continue reading here: https://p4sc4l.substack.com/p/ai-particularly-genai-and-llms-represents