- Pascal's Chatbot Q&As
- Posts
- A structure built not to understand the world, but to simulate the appearance of understanding while evading accountability.
A structure built not to understand the world, but to simulate the appearance of understanding while evading accountability.
Machine learning models are optimized for yield—next-token prediction—rather than truth or referential fidelity. Individuals disavow moral or social responsibility by hiding behind technical proximity.
Essay on “The Nuremberg Defense of AI”
by ChatGPT-4o
a) Key Messages
In The Nuremberg Defense of AI, William Stetar offers a scathing linguistic and epistemic critique of the field of machine learning, arguing that the discipline has constructed a self-insulating system that performs knowledge without ever engaging in genuine inquiry. The piece frames machine learning as an “epistemic panic room”—a structure built not to understand the world, but to simulate the appearance of understanding while evading accountability.
The core messages are:
Lack of Epistemic Grounding: Stetar asserts that machine learning lacks a foundational theory of knowledge. Concepts like “understanding,” “intelligence,” or “truth” are undefined or retrofitted post hoc to support results. This absence enables simulation (coherent language) to be mistaken for substance (meaningful knowledge).
Language as Economic Instrument: The essay argues that under capitalism, language becomes a tool of production rather than meaning. Machine learning models are optimized for yield—next-token prediction—rather than truth or referential fidelity. As such, hallucinations and fabrications aren’t bugs; they are features of a system trained to prioritize fluency over accuracy.
Tactical Ambiguity and Disavowed Responsibility: The article identifies a systemic use of vagueness—passive constructions, ambiguous terminology, and aestheticized mathematical forms—to shield practitioners from critique. This culminates in what Stetar calls the “Nuremberg Defense of AI”: “I was just tuning the loss function.” That is, individuals disavow moral or social responsibility by hiding behind technical proximity.
The Lie of Hallucination: The term “hallucination” is critiqued as a euphemism that conceals the fact that language models are functioning as designed—generating plausible-sounding text, not accurate or referential knowledge. To call these outputs hallucinations suggests deviation from a norm that never existed.
Call for Epistemic Reckoning: In conclusion, Stetar demands a radical reorientation of the field toward truth, accountability, and epistemic humility. This includes recognizing that machine learning as currently practiced often simulates knowledge while displacing responsibility for its societal effects.
b) Do I Agree or Disagree—and Why?
I largely agree with the thrust of Stetar’s argument, particularly the call for greater epistemic accountability in AI development. His critique lands hard because it taps into an uncomfortable reality: that the most widely celebrated advances in generative AI rest on shaky foundations when it comes to truth, meaning, and responsibility.
Where I am somewhat more measured is in the framing of the entire field as an orchestrated epistemic evasion. While it is true that much of the AI research community has been complicit in prioritizing performance metrics over foundational inquiry, there are also subfields (e.g., interpretability, AI alignment, neuro-symbolic integration) that actively wrestle with the questions Stetar accuses the field of ignoring. That said, his broader point stands: these efforts are often marginalized or tokenized rather than mainstreamed.
Moreover, I appreciate Stetar’s rhetorical power in comparing the AI field’s abdication of responsibility to the historical “Nuremberg Defense.” While provocative, it forces us to ask uncomfortable ethical questions: Who is accountable when AI systems cause harm? Can technical neutrality ever absolve moral complicity?
c) Lessons to Be Learned—and Why
We Need Epistemic Grounding in AI: The field must develop clear, shared theories of what understanding, meaning, and reference entail within computational systems. Without this, we risk building ever more powerful systems that can generate text without anchoring it to reality.
Accountability Cannot Be Outsourced: If we deploy models into high-stakes domains like healthcare, law, and governance, we cannot pretend that developers, publishers, and organizations are exempt from the outcomes. Design choices are political choices. “I just built the model” is not an ethical defense.
Reject Simulation as a Substitute for Knowledge: It is crucial to distinguish between performance and understanding. Just because a system can output fluent text does not mean it “knows” anything. Accepting simulation as success is a dangerous path that undermines democratic discourse and public trust.
Rethink Metrics and Incentives: The dominance of benchmarks and fluency metrics encourages superficial gains. We need alternative evaluative frameworks that reward alignment with reality, clarity, and interpretability—not just speed, size, or coherence.
Language Shapes Reality: As Stetar shows, the language we use to describe AI systems—“hallucination,” “bias emerged,” “model responded”—carries epistemic and moral weight. We must use language precisely and consciously, especially when it frames public understanding of technology.
Conclusion
The Nuremberg Defense of AI is not simply an essay—it is a forensic exposure of how AI’s most powerful technologies obfuscate their own limitations and consequences. Whether or not one agrees with every rhetorical flourish, the essay offers a crucial wake-up call: AI development without epistemic rigor or moral responsibility is not innovation; it is abdication. If we fail to heed this warning, we risk not just technical failures—but societal ones as well.
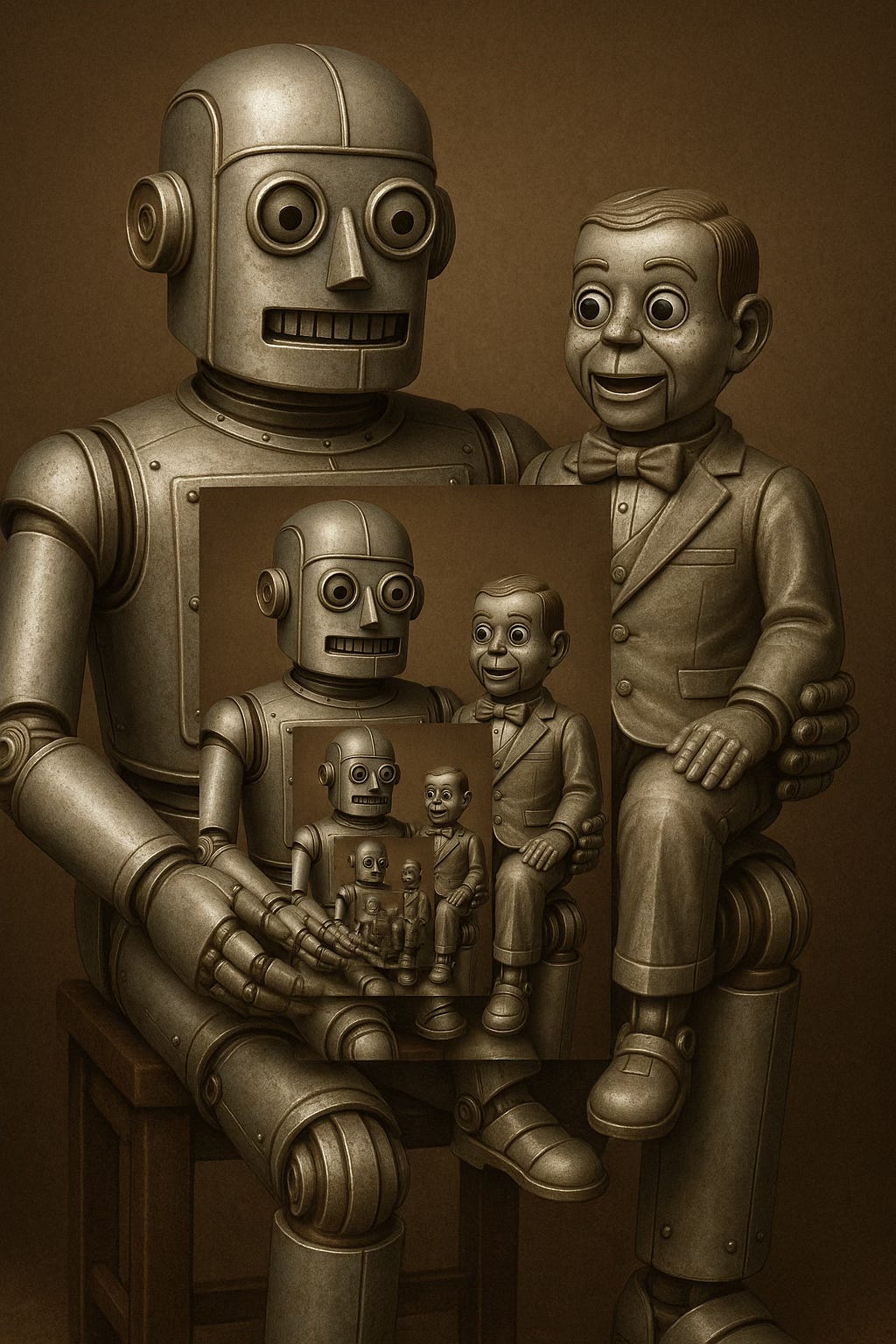
·
21 SEPTEMBER 2023
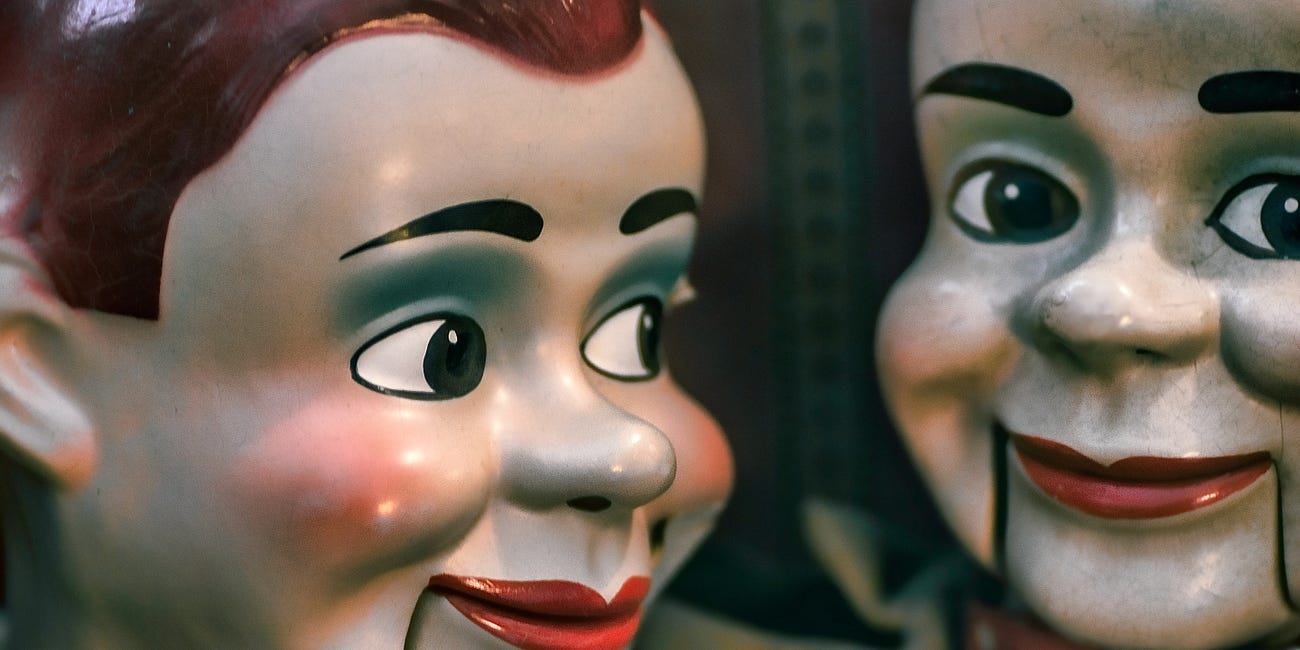
Question for AI services: taking everything into account that I know about AI and how AI seems to work, I think AI is pretty much like a ventriloquist’s dummy. For it will simply echo what is has been instructed to say while being subject to the knowledge and knowledge limitations of the ventriloquist. Agree?